Unambiguous Text Localization, Retrieval, and Recognition for Cluttered Scenes
30TH IEEE CONFERENCE ON COMPUTER VISION AND PATTERN RECOGNITION (CVPR 2017)(2022)
摘要
Text instance as one category of self-described objects provides valuable information for understanding and describing cluttered scenes. The rich and precise high-level semantics embodied in the text could drastically benefit the understanding of the world around us. While most recent visual phrase grounding approaches focus on general objects, this paper explores extracting designated texts and predicting unambiguous scene text information, i.e., to accurately localize and recognize a specific targeted text instance in a cluttered image from natural language descriptions (referring expressions). To address this issue, first a novel recurrent dense text localization network (DTLN) is proposed to sequentially decode the intermediate convolutional representations of a cluttered scene image into a set of distinct text instance detections. Our approach avoids repeated text detections at multiple scales by recurrently memorizing previous detections, and effectively tackles crowded text instances in close proximity. Second, we propose a context reasoning text retrieval (CRTR) model, which jointly encodes text instances and their context information through a recurrent network, and ranks localized text bounding boxes by a scoring function of context compatibility. Third, a recurrent text recognition module is introduced to extend the applicability of aforementioned DTLN and CRTR models, via text verification or transcription. Quantitative evaluations on standard scene text extraction benchmarks and a newly collected scene text retrieval dataset demonstrate the effectiveness and advantages of our models for the joint scene text localization, retrieval, and recognition task.
更多查看译文
关键词
Natural language description,text detection,text retrieval,text recognition,deep neural network,referring expression
AI 理解论文
溯源树
样例
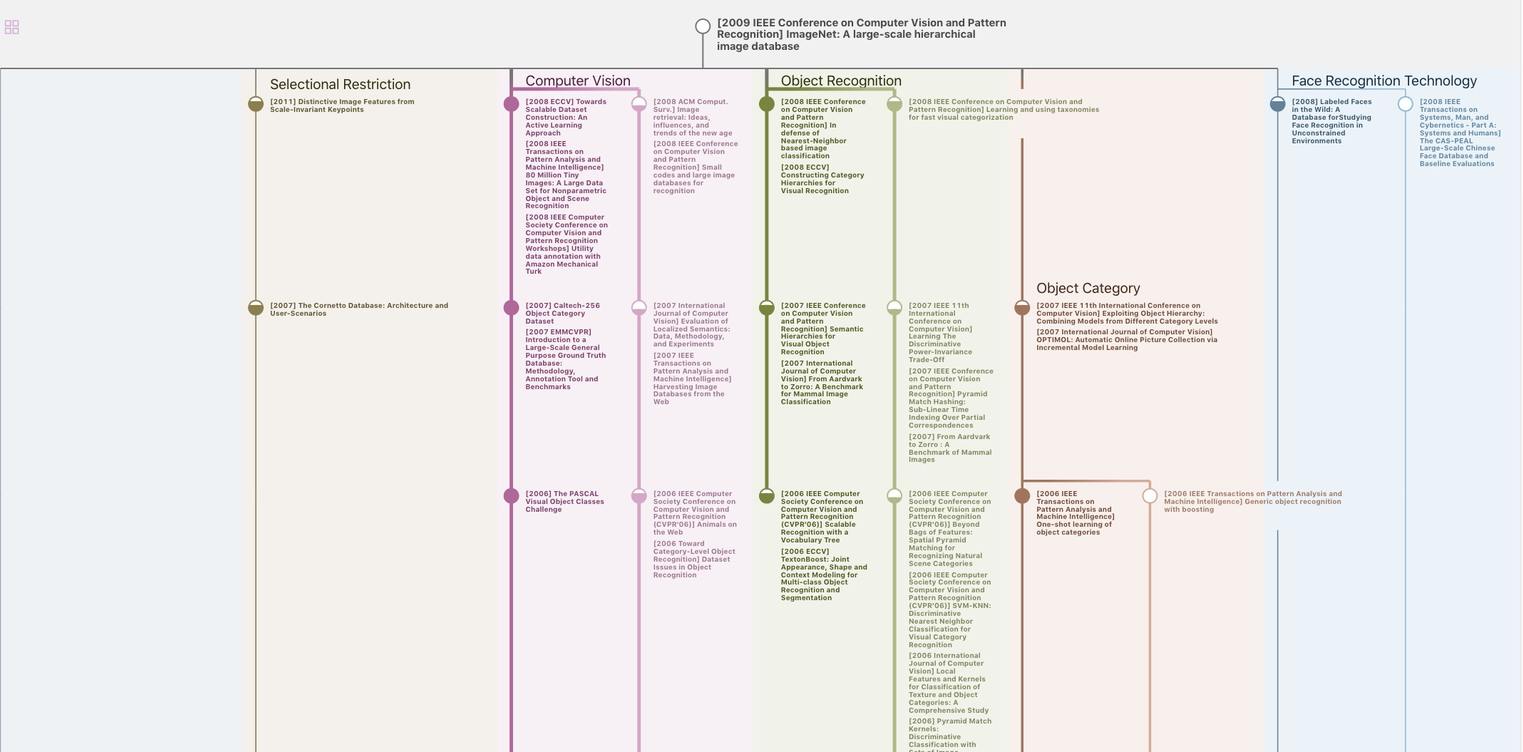
生成溯源树,研究论文发展脉络
Chat Paper
正在生成论文摘要