Efficient False Positive Reduction In Computer-Aided Detection Using Convolutional Neural Networks And Random View Aggregation
DEEP LEARNING AND CONVOLUTIONAL NEURAL NETWORKS FOR MEDICAL IMAGE COMPUTING: PRECISION MEDICINE, HIGH PERFORMANCE AND LARGE-SCALE DATASETS(2017)
摘要
In clinical practice and medical imaging research, automated computer-aided detection (CADe) is an important tool. While many methods can achieve high sensitivities, they typically suffer from high false positives (FP) per patient. In this study, we describe a two-stage coarse-to-fine approach using CADe candidate generation systems that operate at high sensitivity rates (close to 100% recall). In a second stage, we reduce false positive numbers using state-of-the-art machine learning methods, namely deep convolutional neural networks (ConvNet). The ConvNets are trained to differentiate hard false positives from true-positives utilizing a set of 2D (two-dimensional) or 2.5D re-sampled views comprising random translations, rotations, and multi-scale observations around a candidate's center coordinate. During the test phase, we apply the ConvNets on unseen patient data and aggregate all probability scores for lesions (or pathology). We found that this second stage is a highly selective classifier that is able to reject difficult false positives while retaining good sensitivity rates. The method was evaluated on three data sets (sclerotic metastases, lymph nodes, colonic polyps) with varying numbers patients (59, 176, and 1,186, respectively). Experiments show that the method is able to generalize to different applications and increasing data set sizes. Marked improvements are observed in all cases: sensitivities increased from 57 to 70%, from 43 to 77% and from 58 to 75% for sclerotic metastases, lymph nodes and colonic polyps, respectively, at low FP rates per patient (3 FPs/patient).
更多查看译文
AI 理解论文
溯源树
样例
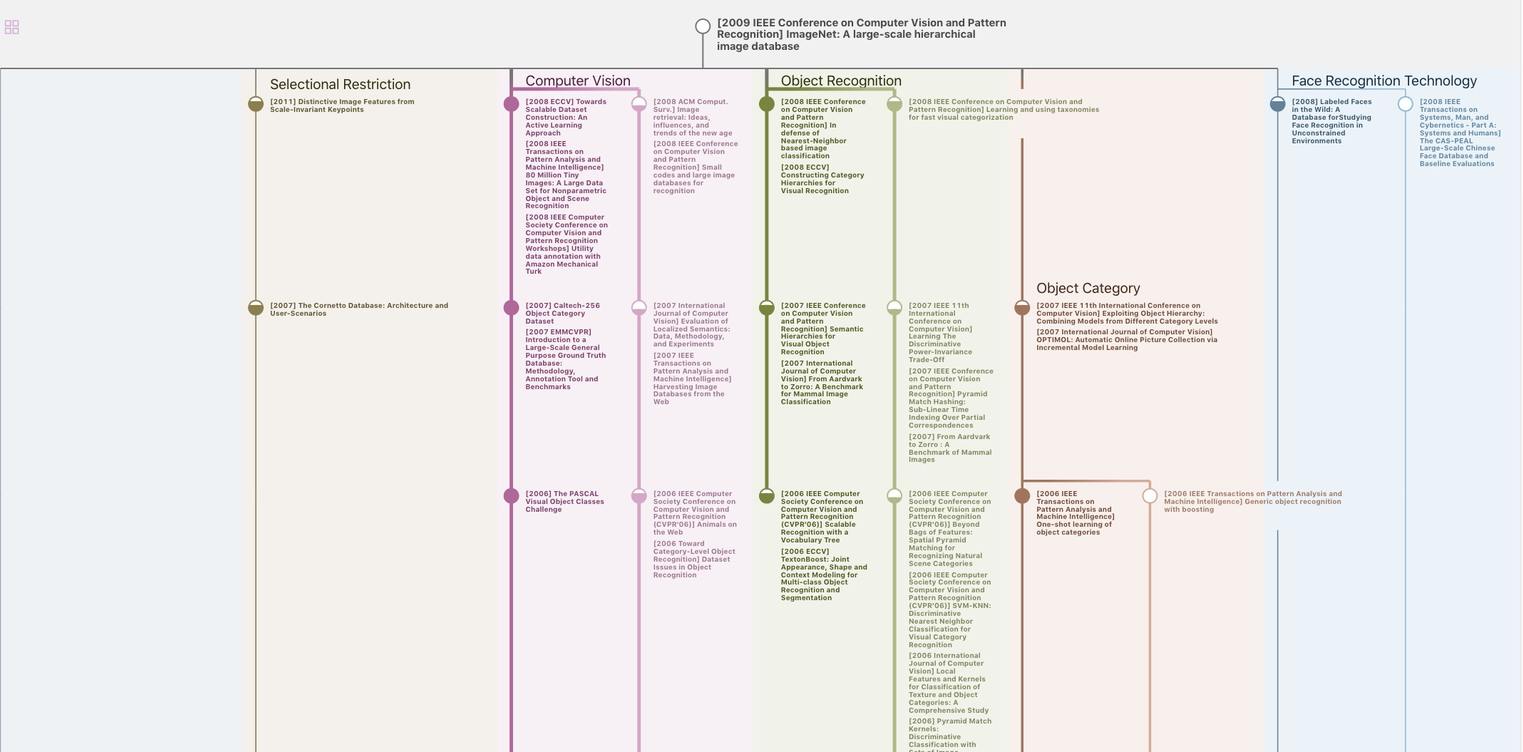
生成溯源树,研究论文发展脉络
Chat Paper
正在生成论文摘要