Model selection based on combined penalties for biomarker identification.
JOURNAL OF BIOPHARMACEUTICAL STATISTICS(2018)
摘要
The growing role of targeted medicine has led to an increased focus on the development of actionable biomarkers. Current penalized selection methods that are used to identify biomarker panels for classification in high-dimensional data, however, often result in highly complex panels that need careful pruning for practical use. In the framework of regularization methods, a penalty that is a weighted sum of the L-1 and L-0 norm has been proposed to account for the complexity of the resulting model. In practice, the limitation of this penalty is that the objective function is non-convex, non-smooth, the optimization is computationally intensive and the application to high-dimensional settings is challenging. In this paper, we propose a stepwise forward variable selection method which combines the L-0 with L-1 or L-2 norms. The penalized likelihood criterion that is used in the stepwise selection procedure results in more parsimonious models, keeping only the most relevant features. Simulation results and a real application show that our approach exhibits a comparable performance with common selection methods with respect to the prediction performance while minimizing the number of variables in the selected model resulting in a more parsimonious model as desired.
更多查看译文
关键词
Biomarker panels,combined penalties,model selection,penalized regression,regularization,sparsity,stepwise variable selection,treatment responder
AI 理解论文
溯源树
样例
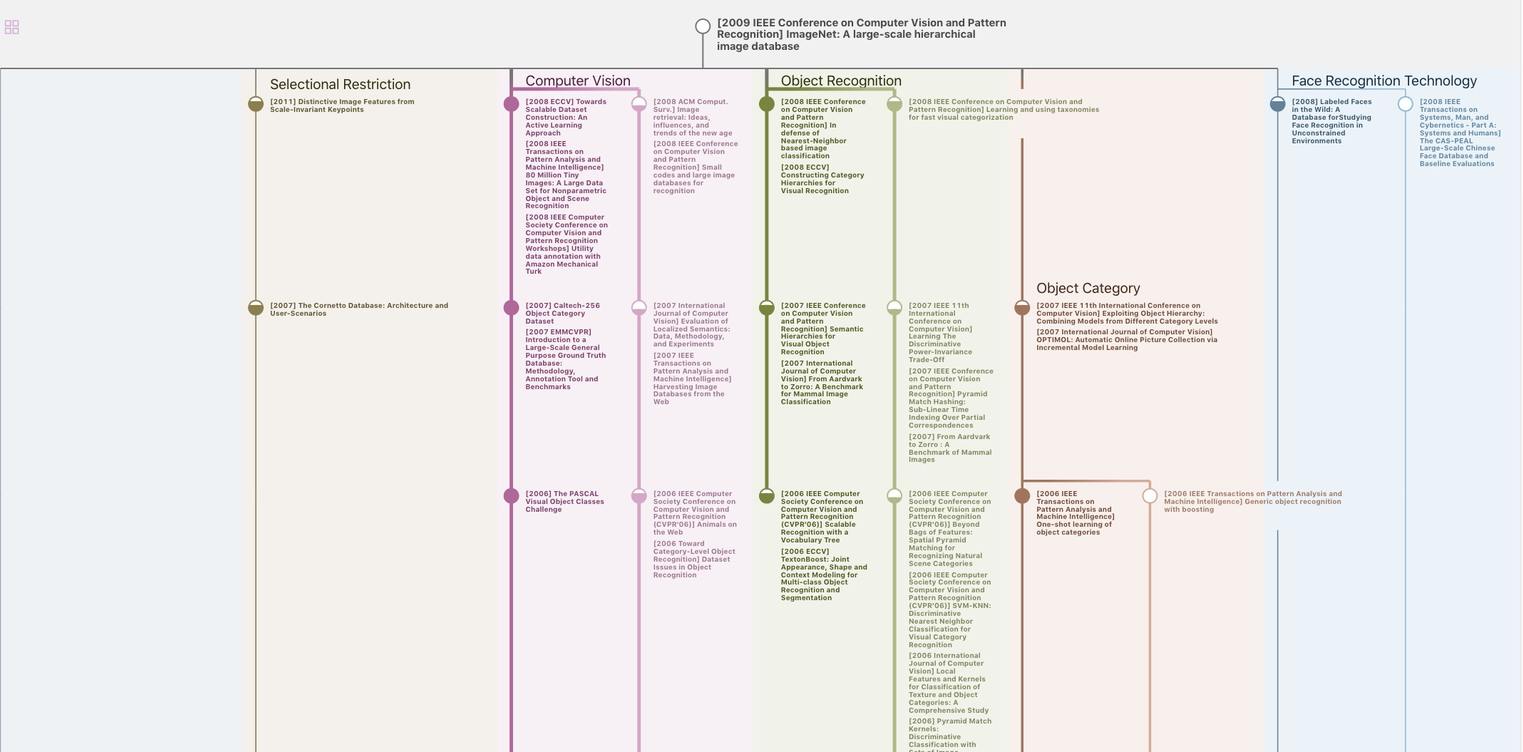
生成溯源树,研究论文发展脉络
Chat Paper
正在生成论文摘要