Unsupervised P2P Rental Recommendations via Integer Programming.
KDD '17: The 23rd ACM SIGKDD International Conference on Knowledge Discovery and Data Mining Halifax NS Canada August, 2017(2017)
摘要
Due to the sparseness of quality rating data, unsupervised recommender systems are used in many applications in Peer to Peer (P2P) rental marketplaces such as Airbnb, FlipKey, and HomeAway. We present an integer programming based recommender systems, where both accommodation benefits and community risks of lodging places are measured and incorporated into an objective function as utility measurements. More specifically, we first present an unsupervised fused scoring method for quantifying the accommodation benefits and community risks of a lodging with crowd-sourced geo-tagged data. In order to the utility of recommendations, we formulate the unsupervised P2P rental recommendations as a constrained integer programming problem, where the accommodation benefits of recommendations are maximized and the community risks of recommendations are minimized, while maintaining constraints on personalization. Furthermore, we provide an efficient solution for the optimization problem by developing a learning-to-integer-programming method for combining aggregated listwise learning to rank into branching variable selection. We apply the proposed approach to the Airbnb data of New York City and provide lodging recommendations to travelers. In our empirical experiments, we demonstrate both the efficiency and effectiveness of our method in terms of striving a trade-off between the user satisfaction, time on market, and the number of reviews, and achieving a balance between positive and negative sides.
更多查看译文
关键词
Unsupervised Recommendations,Integer Programming,Learning To Optimize
AI 理解论文
溯源树
样例
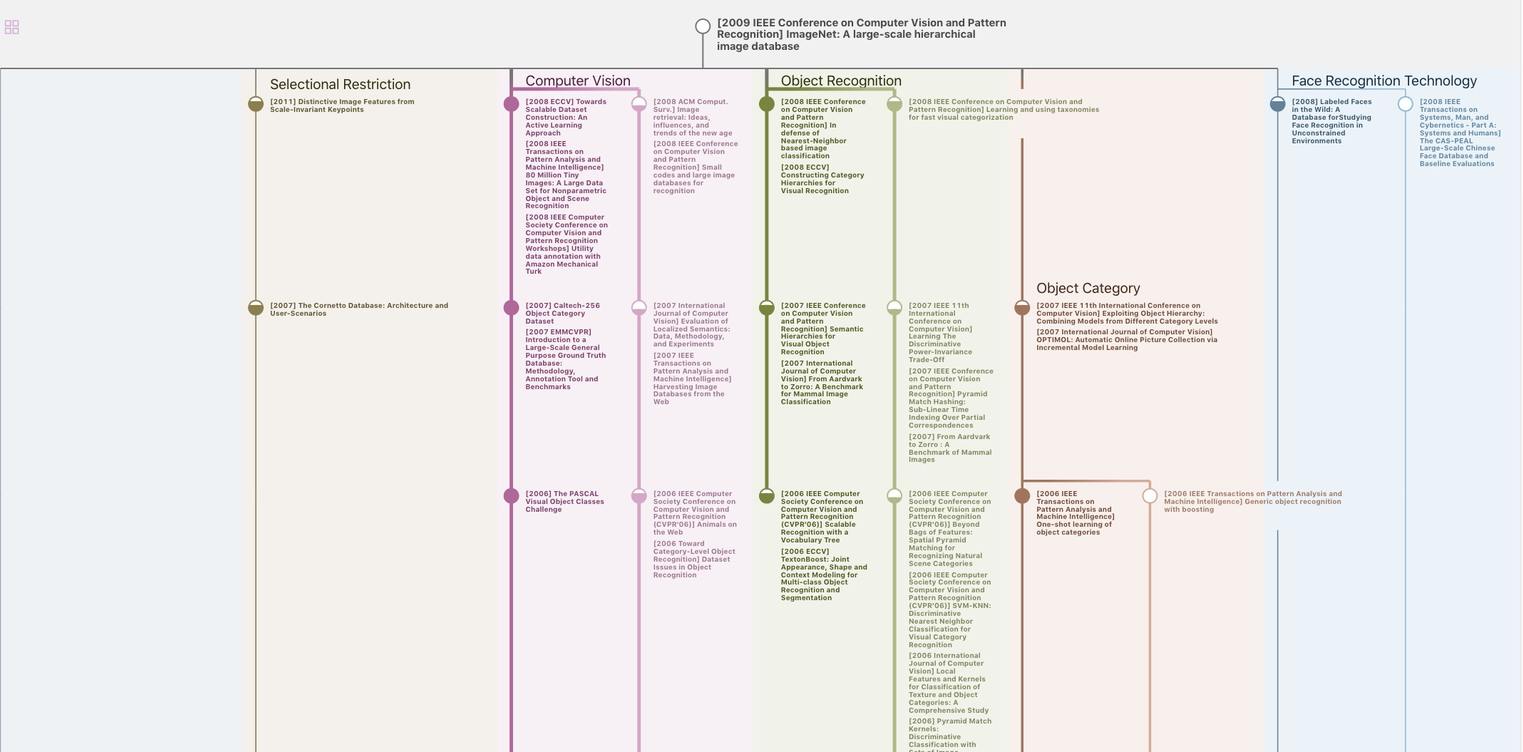
生成溯源树,研究论文发展脉络
Chat Paper
正在生成论文摘要