CFNN: Correlation Filter Neural Network for Visual Object Tracking.
IJCAI(2017)
摘要
Albeit convolutional neural network (CNN) has shown promising capacity in many computer vision tasks, applying it to visual tracking is yet far from solved. Existing methods either employ a large external dataset to undertake exhaustive pretraining or suffer from less satisfactory results in terms of accuracy and robustness. To track single target in a wide range of videos, we present a novel Correlation Filter Neural Network architecture, as well as a complete visual tracking pipeline, The proposed approach is a special case of CNN, whose initialization does not need any pre-training on the external dataset. The initialization of network enjoys the merits of cyclic sampling to achieve the appealing discriminative capability, while the network updating scheme adopts advantages from back-propagation in order to capture new appearance variations. The tracking pipeline integrates both aspects well by making them complementary to each other. We validate our tracker on OTB- 2013 benchmark. The proposed tracker obtains the promising results compared to most of existing representative trackers.
更多查看译文
AI 理解论文
溯源树
样例
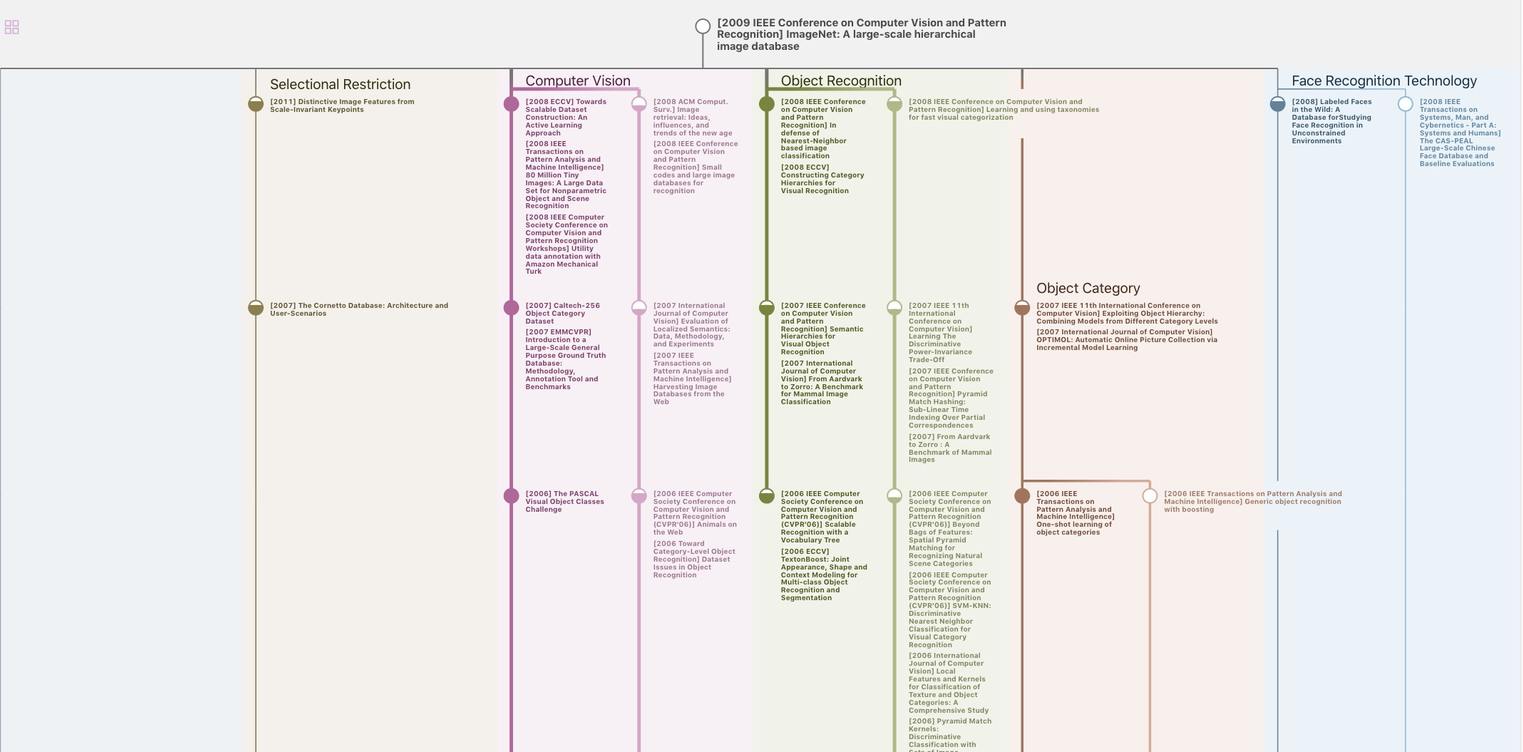
生成溯源树,研究论文发展脉络
Chat Paper
正在生成论文摘要