Radiometric Identification Based on Low-Rank Representation and Minimum Prediction Error Regularization.
IEEE Communications Letters(2017)
摘要
Recently, radiometric identification has attracted much attention. Previous research works considered feature extraction and classification separately, which resulted in that the extracted RF fingerprint features may not be good features and not be suitable for a designated classifier. In this letter, a novel radiometric identification method is proposed to improve the performance of radiometric identification. This method attempts to find a low-rank representation matrix of original data and the optimal classifier parameter simultaneously, by imposing a regularization item of minimum prediction error. Since the recovered radio-frequency fingerprint features are discriminative, the proposed method is suitable for radiometric identification. Experiments on real data sets demonstrate that the proposed method not only outperforms the competitive methods with a higher accuracy of radiometric identification but also has a better robustness against noise. The results indicate that the proposed algorithm can discriminate different emitters with high classification accuracy even if they are from the same manufacturer.
更多查看译文
关键词
Feature extraction,Radio frequency,Radiometry,Optimization,Robustness,Training data,Transient analysis
AI 理解论文
溯源树
样例
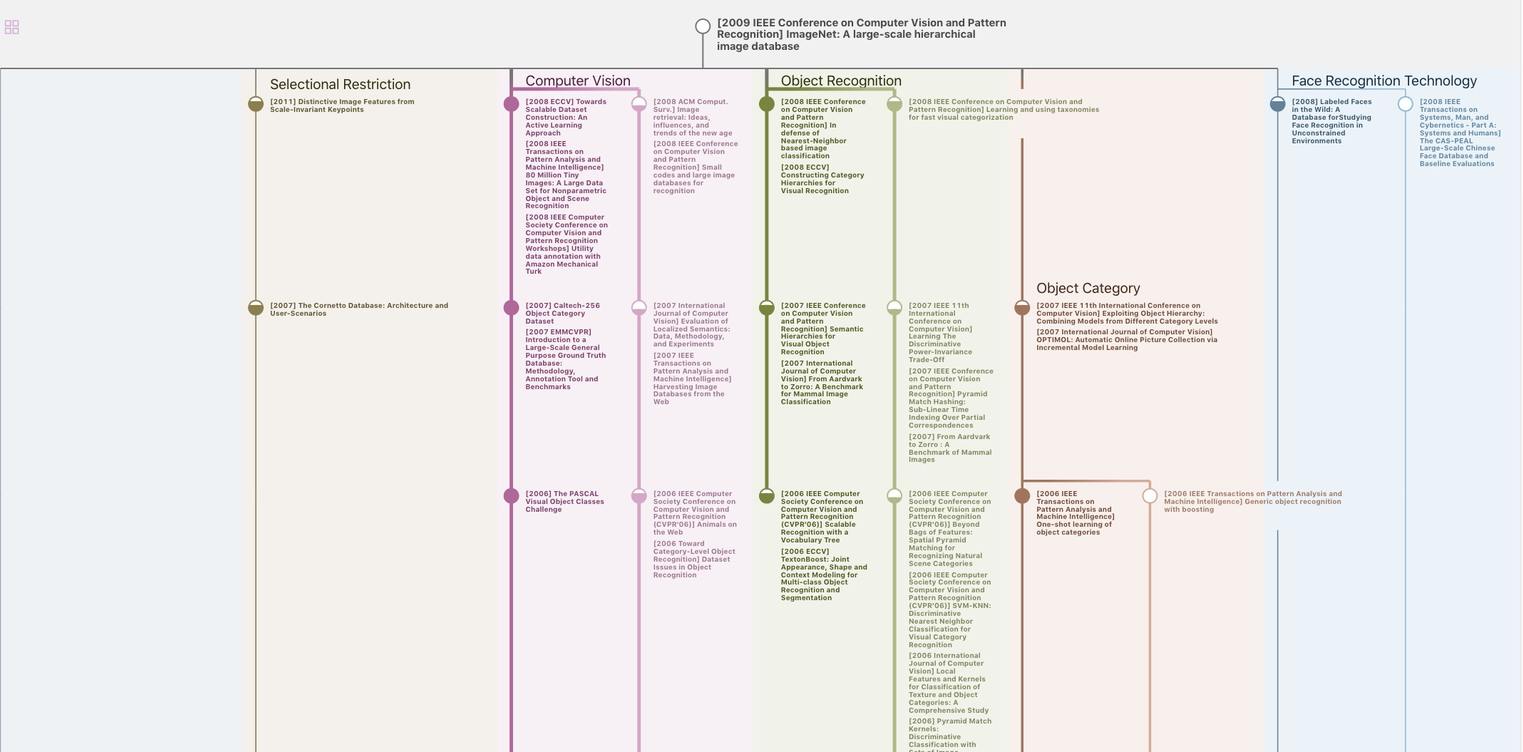
生成溯源树,研究论文发展脉络
Chat Paper
正在生成论文摘要