An Improved Faster R-CNN for Same Object Retrieval.
IEEE ACCESS(2017)
摘要
An improved faster region-based convolutional neural network (R-CNN) [same object retrieval (SOR) faster R-CNN] is proposed to retrieve the same object in different scenes with few training samples. By concatenating the feature maps of shallow and deep convolutional layers, the ability of Regions of Interest (RoI) pooling to extract more detailed features is improved. In the training process, a pretrained CNN model is fine-tuned using a query image data set, so that the confidence score can identify an object proposal to the object level rather than the classification level. In the query process, we first select the ten images for which the object proposals have the closest confidence scores to the query object proposal. Then, the image for which the detected object proposal has the minimum cosine distance to the query object proposal is considered as the query result. The proposed SOR faster R-CNN is applied to our Coke cans data set and three public image data sets, i.e., Oxford Buildings 5k, Paris Buildings 6k, and INS 13. The experimental results confirm that SOR faster R-CNN has better identification performance than fine-tuned faster R-CNN. Moreover, SOR faster R-CNN achieves much higher accuracy for detecting low-resolution images than the fine-tuned faster R-CNN on the Coke cans (0.094 mAP higher), Oxford Buildings (0.043 mAP higher), Paris Buildings (0.078 mAP higher), and INS 13 (0.013 mAP higher) data sets.
更多查看译文
关键词
CNN,faster R-CNN,same object retrieval
AI 理解论文
溯源树
样例
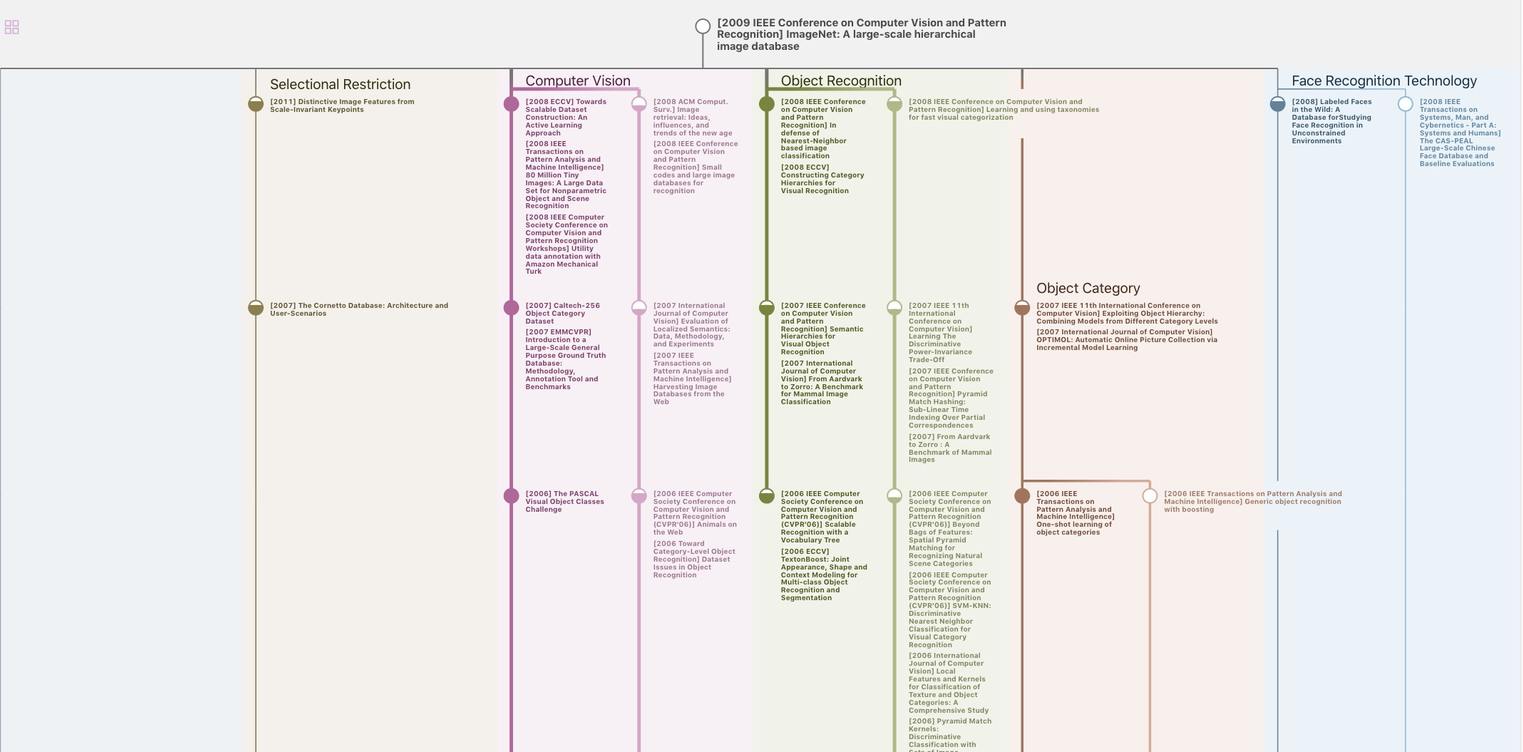
生成溯源树,研究论文发展脉络
Chat Paper
正在生成论文摘要