A constant-time SLAM back-end in the continuum between global mapping and submapping
Periodicals(2016)
摘要
AbstractThis work addresses the development and application of a novel approach, called sparser relative bundle adjustment SRBA, which exploits the inherent flexibility of the relative bundle adjustment RBA framework to devise a continuum of strategies, ranging from RBA with linear graphs to classic bundle adjustment BA in global coordinates, where submapping with local maps emerges as a natural intermediate solution. This method leads to graphs that can be optimized in bounded time even at loop closures, regardless of the loop length. Furthermore, it is shown that the pattern in which relative coordinate variables are defined among keyframes has a significant impact on the graph optimization problem. By using the proposed scheme, optimization can be done more efficiently than in standard RBA, allowing the optimization of larger local maps for any given maximum computational cost. The main algorithms involved in the graph management, along with their complexity analyses, are presented to prove their bounded-time nature. One key advance of the present work is the demonstration that, under mild assumptions, the spanning trees for every single keyframe in the map can be incrementally built by a constant-time algorithm, even for arbitrary graph topologies. We validate our proposal within the scope of visual stereo simultaneous localization and mapping SLAM by developing a complete system that includes a front-end that seamlessly integrates several state-of-the-art computer vision techniques such as ORB features and bag-of-words, along with a decision scheme for keyframe insertion and a SRBA-based back-end that operates as graph optimizer. Finally, a set of experiments in both indoor and outdoor conditions is presented to test the capabilities of this approach. Open-source implementations of the SRBA back-end and the stereo front-end have been released online.
更多查看译文
AI 理解论文
溯源树
样例
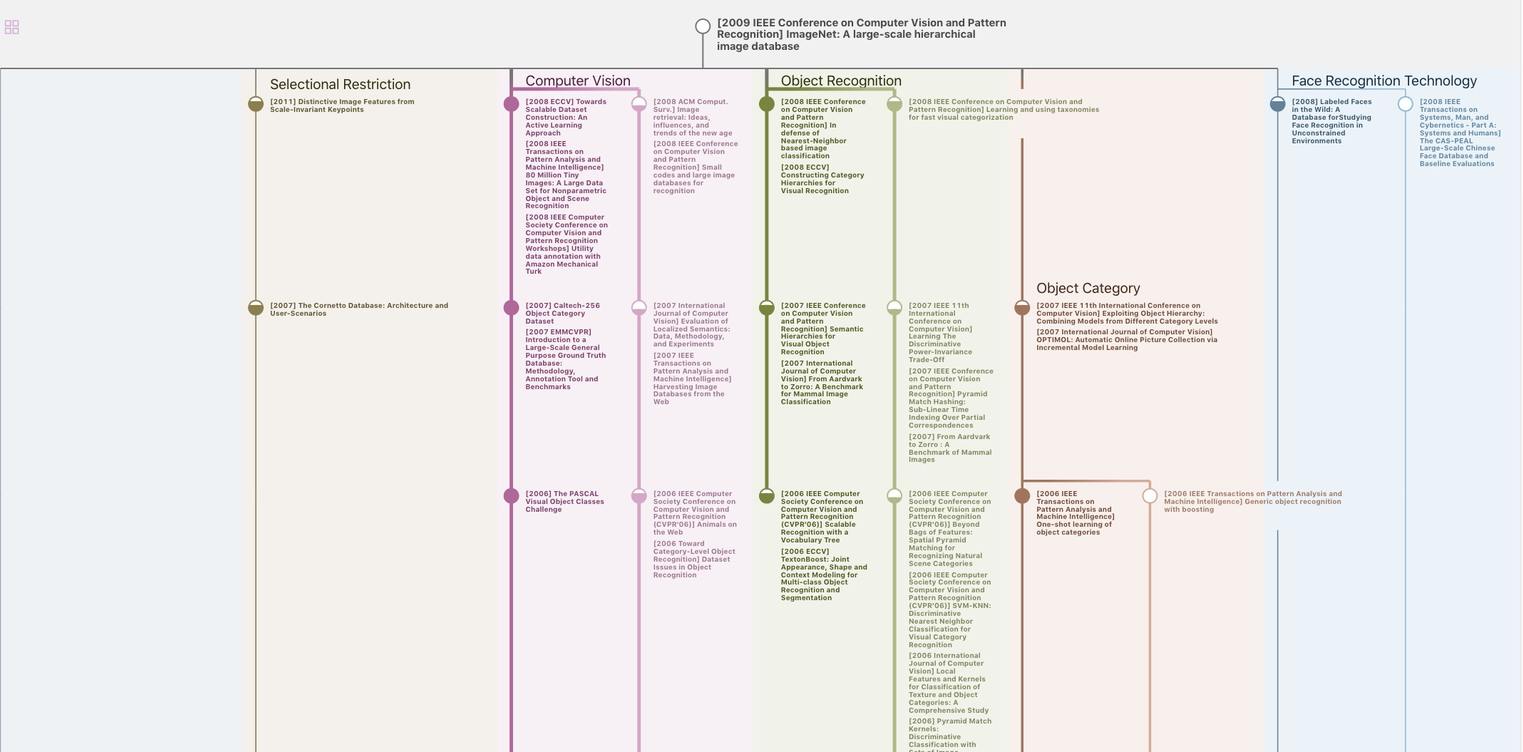
生成溯源树,研究论文发展脉络
Chat Paper
正在生成论文摘要