Coarrays, MUSIC, and the Cramér–Rao Bound
IEEE Transactions on Signal Processing(2017)
摘要
Sparse linear arrays, such as coprime arrays and nested arrays, have the attractive capability of providing enhanced degrees of freedom. By exploiting the coarray structure, an augmented sample covariance matrix can be constructed and MUtiple SIgnal Classification (MUSIC) can be applied to identify more sources than the number of sensors. While such a MUSIC algorithm works quite well, its performance has not been theoretically analyzed. In this paper, we derive a simplified asymptotic mean square error (MSE) expression for the MUSIC algorithm applied to the coarray model, which is applicable even if the source number exceeds the sensor number. We show that the directly augmented sample covariance matrix and the spatial smoothed sample covariance matrix yield the same asymptotic MSE for MUSIC. We also show that when there are more sources than the number of sensors, the MSE converges to a positive value instead of zero when the signal-to-noise ratio (SNR) goes to infinity. This finding explains the “saturation” behavior of the coarray-based MUSIC algorithms in the high-SNR region observed in previous studies. Finally, we derive the Cramér–Rao bound for sparse linear arrays, and conduct a numerical study of the statistical efficiency of the coarray-based estimator. Experimental results verify theoretical derivations and reveal the complex efficiency pattern of coarray-based MUSIC algorithms.
更多查看译文
关键词
Multiple signal classification,Covariance matrices,Sensor arrays,Array signal processing,Signal to noise ratio,Signal processing algorithms
AI 理解论文
溯源树
样例
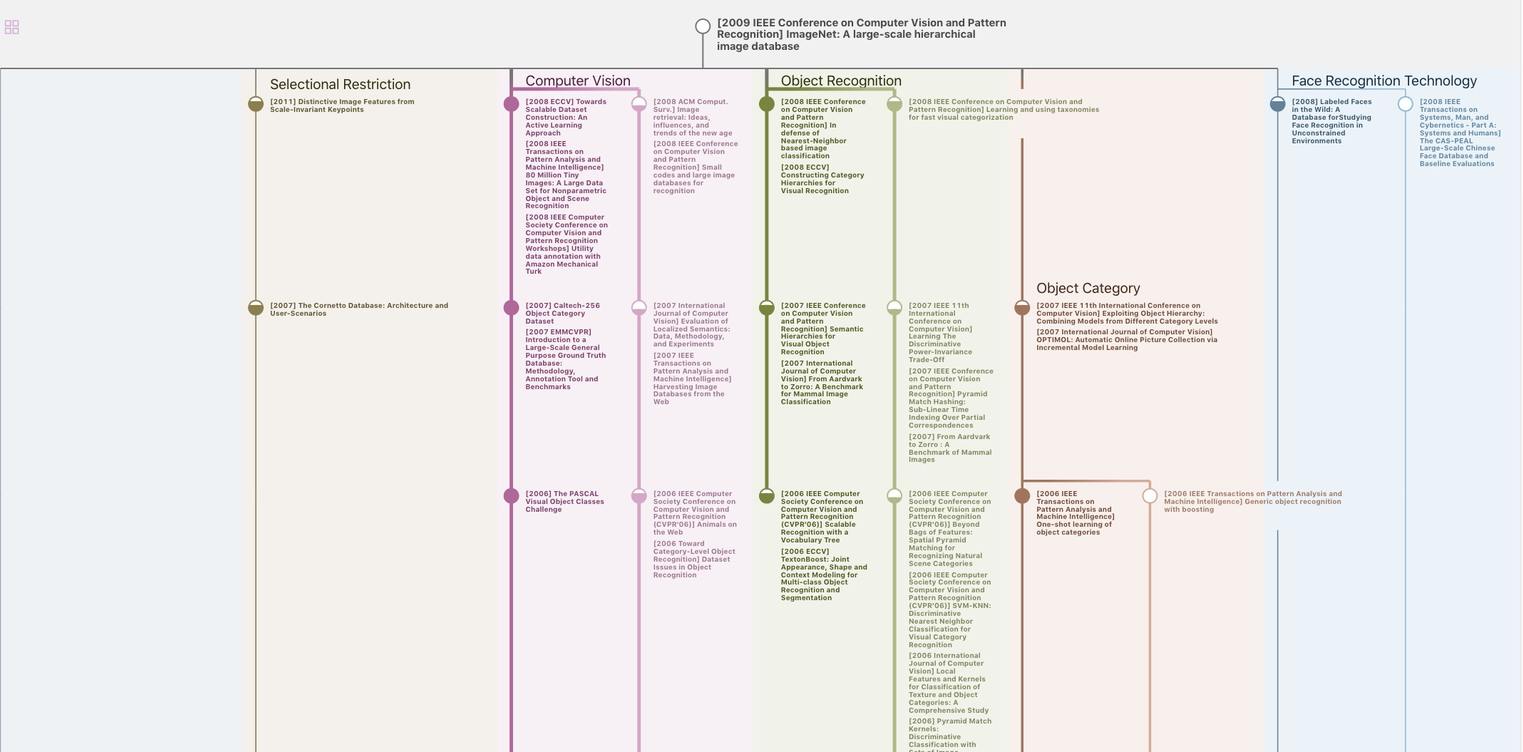
生成溯源树,研究论文发展脉络
Chat Paper
正在生成论文摘要