Perception oriented transmission estimation for high quality image dehazing.
Neurocomputing(2017)
摘要
Single image dehazing has captured much attention due to increasing applications. However, state-of-the-art image dehazing algorithms often suffer from undesirable quantization artifacts and noises in heavily hazy regions or sky patches of hazy image where dense scattering often occurs, so that dehazed results may have poor image quality or even lose the original spectral or structural information. To address this problem, we propose a perception oriented transmission estimation method for high quality image dehazing. As the key contribution, a novel transmission model is firstly developed by posing image dehazing as a local contrast optimization problem. This transmission model can flexibly adjust haze removal to accommodate the expected local contrast gain. Specially, this model can lead to a solution which is similar to the one using the dark channel prior, but it is not confined to the dark channel prior assumption. Then, in order to remove haze and simultaneously suppress quantization artifacts and noises, two specific steps are introduced. First, we develop a scattering-aware method via a Bayesian framework to estimate the scattering probability of each pixel in a hazy image. Second, a perceptually adaptive parameter selection scheme is proposed to determine the expected contrast gain for the transmission estimation by taking advantage of the just-noticeable-distortion (JND) model. Experimental results demonstrate that the proposed algorithm can effectively remove haze and suppress undesirable degradation on dehazed images, both quantitatively and qualitatively, when compared with the state-of-the-art algorithms under dense scattering conditions.
更多查看译文
关键词
Image dehazing,Just-noticeable-distortion,Scattering-aware,Perceptual transmission estimation,Quantization artifacts or noise suppression,High image quality
AI 理解论文
溯源树
样例
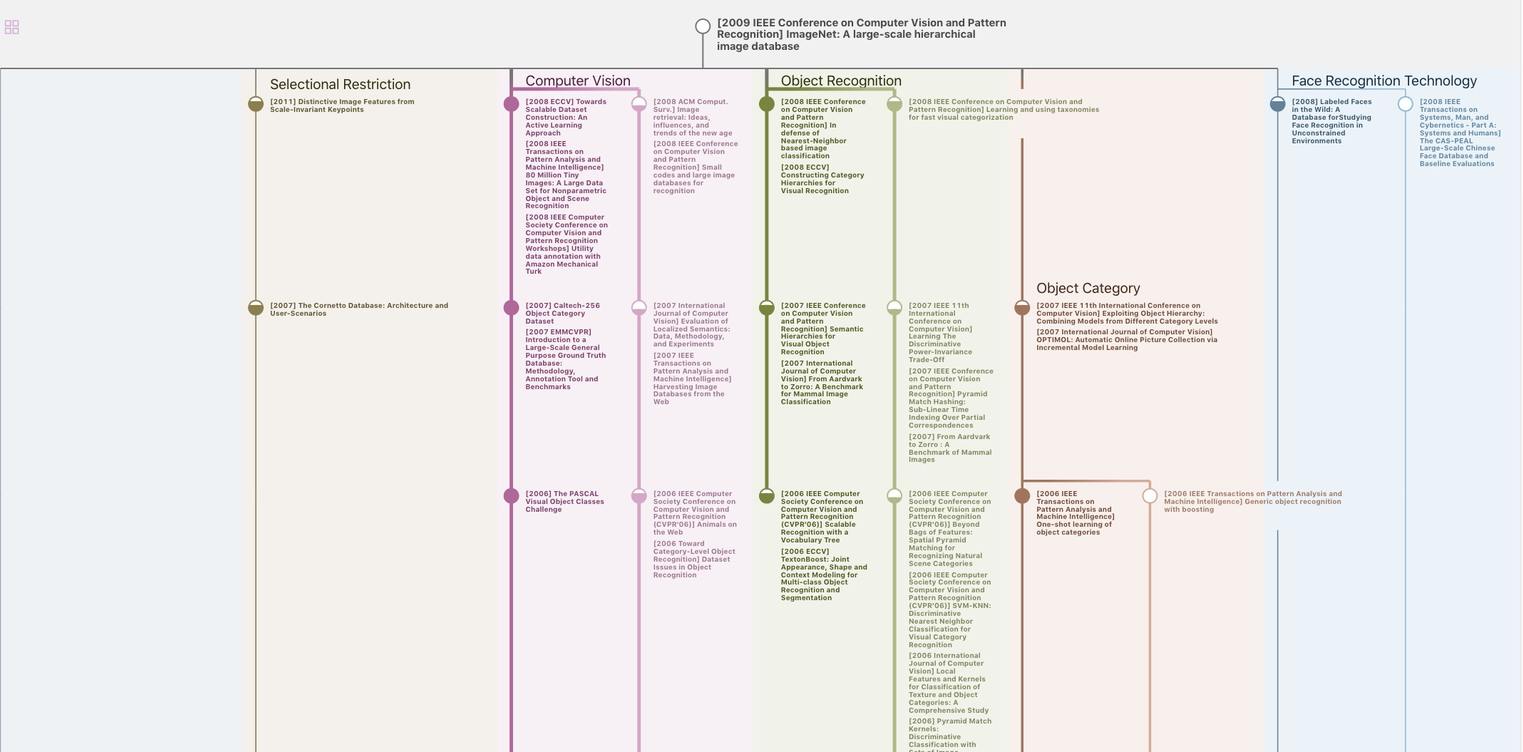
生成溯源树,研究论文发展脉络
Chat Paper
正在生成论文摘要