Pricing and Optimization in Shared Vehicle Systems: An Approximation Framework.
EC(2017)
摘要
Optimizing shared vehicle systems (bike-sharing/car-sharing/ride-sharing) is more challenging compared to traditional resource allocation settings due to the presence of complex network externalities. In particular, changes in the demand/supply at any location (via dynamic pricing, rebalancing of empty vehicles, etc.) affect future supply throughout the system within short timescales. Such externalities are well captured by steady-state Markovian models, which are therefore widely used to analyze and design shared vehicle systems. However, using such models to design pricing/control policies is computationally difficult since the resulting optimization problems are high-dimensional and non-convex. To this end, we develop a general approximation framework for designing pricing policies in shared vehicle systems, based on a novel convex relaxation which we term elevated flow relaxation. Our approach provides the first efficient algorithms with rigorous approximation guarantees for a wide range of objective functions (throughput, revenue, welfare). For any shared vehicle system with $n$ stations and m vehicles, our framework provides a pricing policy with an approximation ratio of 1+(n-1)/m. This guarantee is particularly meaningful when m/n, the average number of vehicles per station is large, as is often the case in practice. Further, the simplicity of our approach allows us to extend it to more complex settings. Apart from pricing, shared vehicle systems enable other control levers for modulating demand and supply, e.g. rebalancing empty vehicles, redirecting riders to nearby vehicles, etc. Our approach yields efficient algorithms with the same approximation guarantees for all these problems, and in the process, obtains as special cases several existing heuristics and asymptotic guarantees. We also extend our approach to obtain bi-criterion guarantees in multi-objective settings; we illustrate this with the example of Ramsey pricing. From a technical perspective, our work develops a new approach for obtaining control policies with approximation guarantees in steady-state Markovian models. Our approach can be distilled into the following three-step program: (i) construct an upper bound via a relaxation to the original problem that encodes essential conservation laws of the system, (ii) identify a family of control policies inducing known steady-state distributions that achieve the value of the relaxed solution in an appropriate scaling limit (in our case, state-independent policies in the limit m++), and (iii) characterize the performance loss between the finite system (i.e. fixed m) and the scaling limit. This technique may be of independent interest for other settings.
更多查看译文
关键词
Qeueing systems, Revenue management, Sharing Economy
AI 理解论文
溯源树
样例
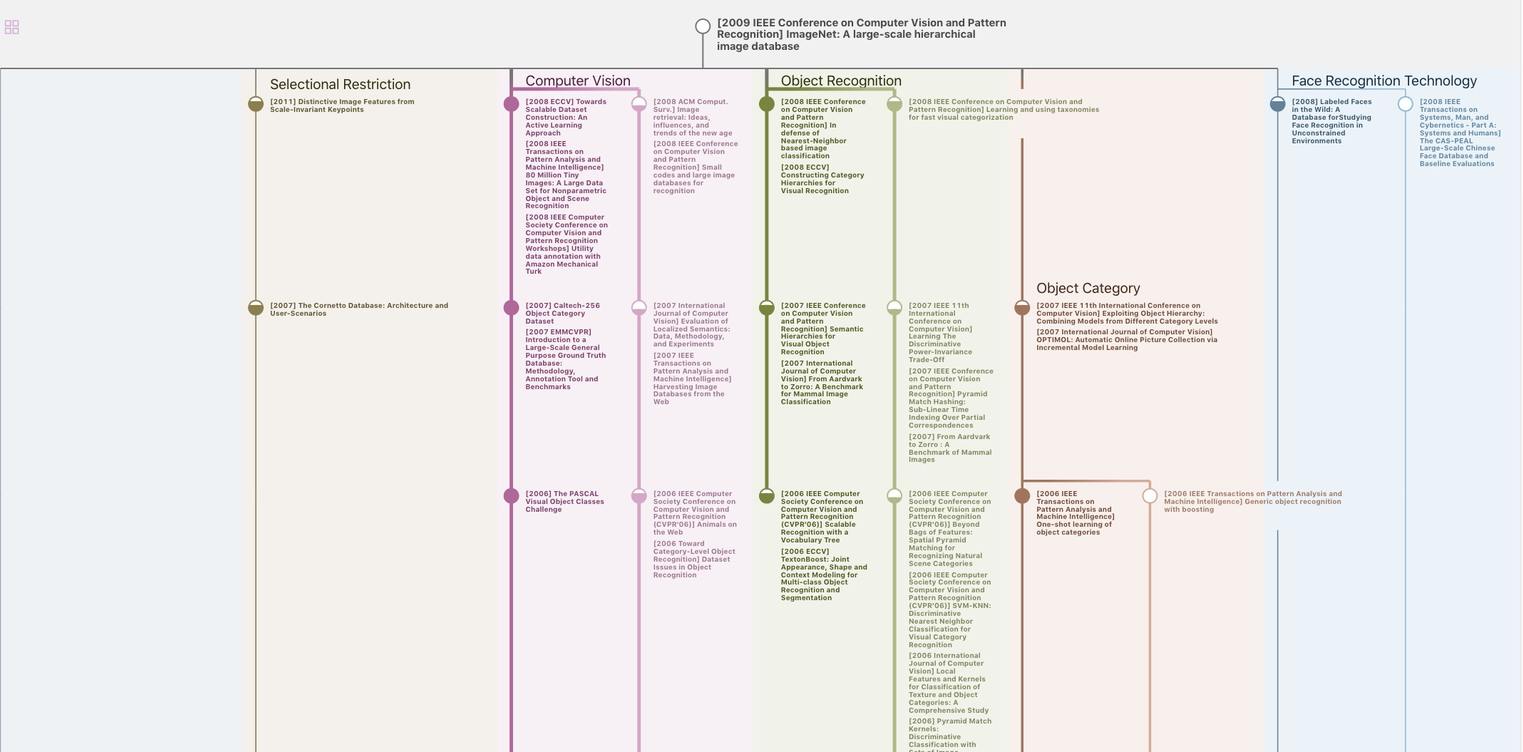
生成溯源树,研究论文发展脉络
Chat Paper
正在生成论文摘要