Fast Non-Blind Deconvolution Via Regularized Residual Networks With Long/Short Skip-Connections
2017 IEEE INTERNATIONAL CONFERENCE ON COMPUTATIONAL PHOTOGRAPHY (ICCP 2017)(2017)
摘要
This paper proposes a novel framework for non-blind deconvolution using deep convolutional network. To deal with various blur kernels, we reduce the training complexity using Wiener filter as a preprocessing step in our framework. This step generates amplified noise and ringing artifacts, but the artifacts are little correlated with the shapes of blur kernels, making the input of our network independent of the blur kernel shape. Our network is trained to effectively remove those artifacts via a residual network with long/short skip-connections. We also add a regularization to help our network robustly process untrained and inaccurate blur kernels by suppressing abnormal weights of convolutional layers that may incur overfitting. Our postprocessing step can further improve the deconvolution quality. Experimental results demonstrate that our framework can process images blurred by a variety of blur kernels with faster speed and comparable image quality to the state-of-the-art methods.
更多查看译文
关键词
fast nonblind deconvolution,regularized residual networks,long skip-connections,short skip-connections,deep convolutional network,blur kernels,training complexity,Wiener filter,amplified noise,ringing artifacts,abnormal weights suppression,image quality
AI 理解论文
溯源树
样例
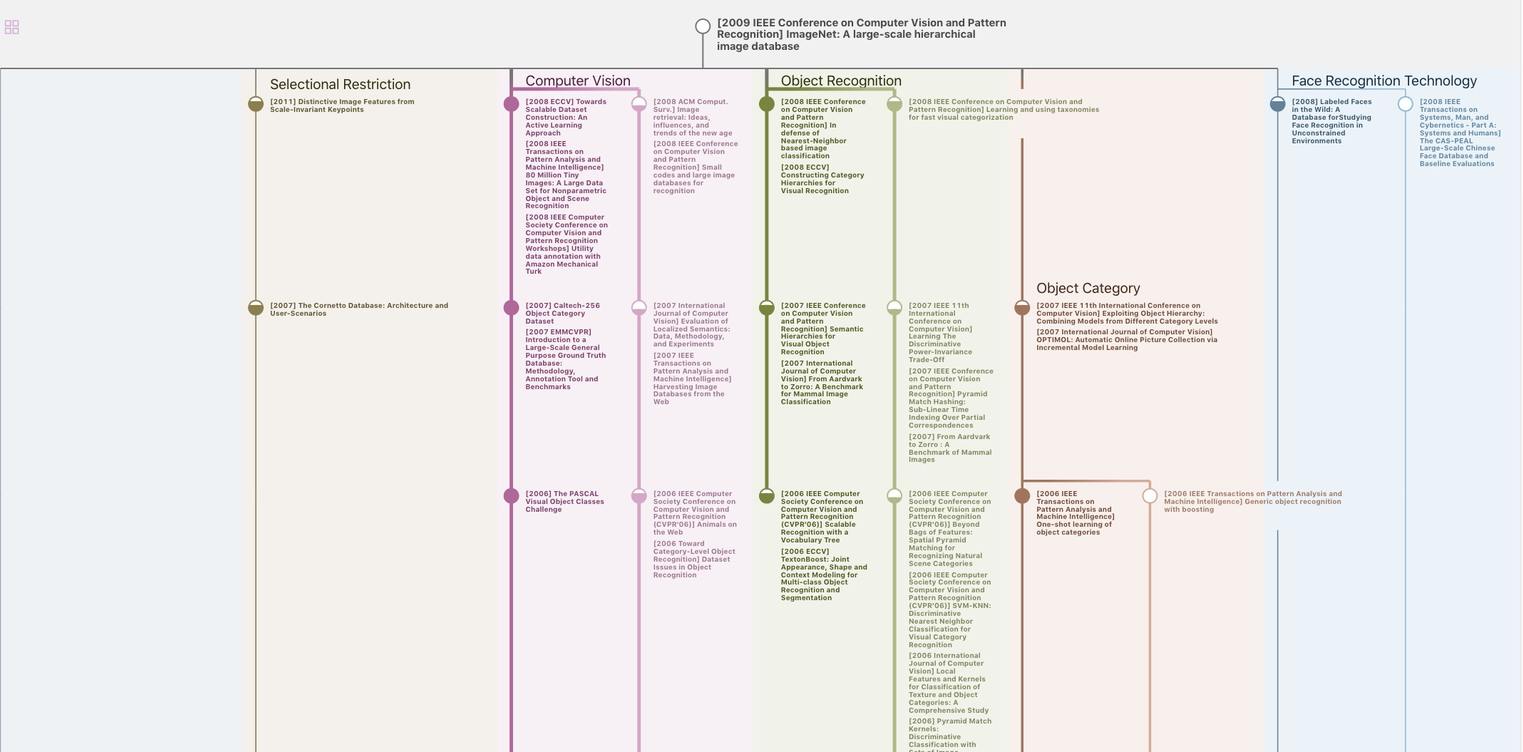
生成溯源树,研究论文发展脉络
Chat Paper
正在生成论文摘要