Trajectory Clustering Via Deep Representation Learning
2017 INTERNATIONAL JOINT CONFERENCE ON NEURAL NETWORKS (IJCNN)(2017)
摘要
Trajectory clustering, which aims at discovering groups of similar trajectories, has long been considered as a corner stone task for revealing movement patterns as well as facilitating higher-level applications like location prediction. While a plethora of trajectory clustering techniques have been proposed, they often rely on spatiotemporal similarity measures that are not space-and time-invariant. As a result, they cannot detect trajectory clusters where the within-cluster similarity occurs in different regions and time periods. In this paper, we revisit the trajectory clustering problem by learning quality low-dimensional representations of the trajectories. We first use a sliding window to extract a set of moving behavior features that capture space-and time-invariant characteristics of the trajectories. With the feature extraction module, we transform each trajectory into a feature sequence to describe object movements, and further employ a sequence to sequence auto-encoder to learn fixed-length deep representations. The learnt representations robustly encode the movement characteristics of the objects and thus lead to space-and time-invariant clusters. We evaluate the proposed method on both synthetic and real data, and observe significant performance improvements over existing methods.
更多查看译文
关键词
trajectory clustering,deep representation learning,sliding window,feature extraction module,feature sequence,object movements,sequence autoencoder,cluster analysis,trajectory pattern mining
AI 理解论文
溯源树
样例
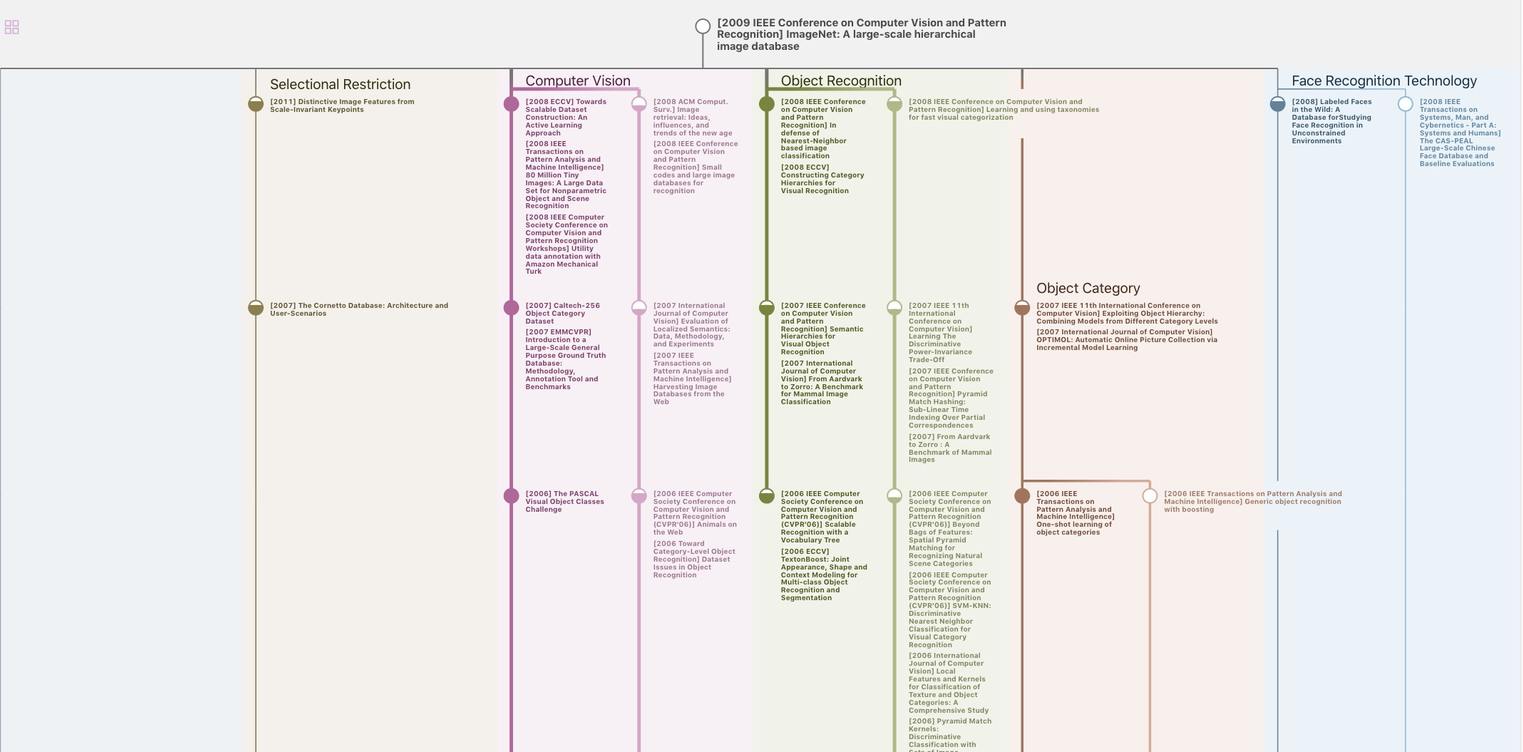
生成溯源树,研究论文发展脉络
Chat Paper
正在生成论文摘要