How To Get Pavement Distress Detection Ready For Deep Learning? A Systematic Approach
2017 INTERNATIONAL JOINT CONFERENCE ON NEURAL NETWORKS (IJCNN)(2017)
摘要
Road condition acquisition and assessment are the key to guarantee their permanent availability. In order to maintain a country's whole road network, millions of high-resolution images have to be analyzed annually. Currently, this requires cost and time excessive manual labor. We aim to automate this process to a high degree by applying deep neural networks. Such networks need a lot of data to be trained successfully, which are not publicly available at the moment. In this paper, we present the GAPs dataset, which is the first freely available pavement distress dataset of a size, large enough to train high-performing deep neural networks. It provides high quality images, recorded by a standardized process fulfilling German federal regulations, and detailed distress annotations. For the first time, this enables a fair comparison of research in this field. Furthermore, we present a first evaluation of the state of the art in pavement distress detection and an analysis of the effectiveness of state of the art regularization techniques on this dataset.
更多查看译文
关键词
pavement distress detection,deep learning,road condition acquisition,road condition assessment,road network,high-resolution images,deep neural networks,GAPs dataset,high quality images,German federal regulations,distress annotations
AI 理解论文
溯源树
样例
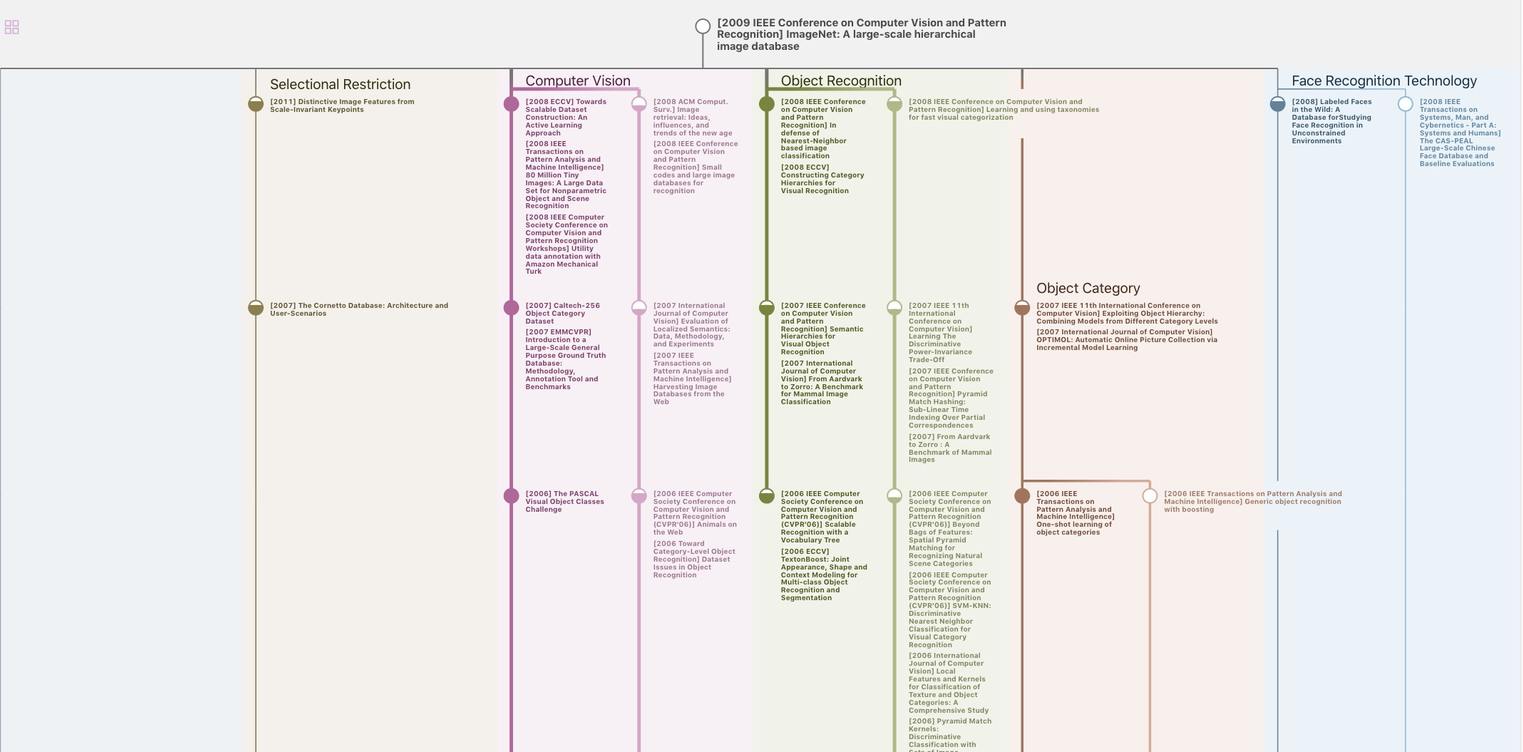
生成溯源树,研究论文发展脉络
Chat Paper
正在生成论文摘要