An efficient semi-supervised SVM for anomaly detection.
IJCNN(2017)
摘要
Semi-Supervised Support Vector Machines (S3VMs) have been proposed to deal with the proliferation of partially labelled data available in many large-scale complex systems. Since most existing S3VMs do not correspond to convex problems nor have practical solutions especially on large imbalanced data, this limits their utility in practice. In this work, we propose an efficient approach to the semi-supervised problem especially for anomaly detection, termed S3VMAD. We formulate S3VMAD in unsupervised paradigm for anomaly detection. It is a convex objective function which is guaranteed to have a global optimum solution. The proposed approach is compatible with both supervised Support Vector Machines (SVMs) and unsupervised One-Class SVM (OCSVM). In addition, it exploits efficient optimisation techniques in an online fashion as well as in batch mode, which enables the solution to be scalable and therefore practical in the case of large datasets. In order to investigate the efficiency and effectiveness of the proposed S3VMAD, experiments on various sample datasets compare with supervised SVM learning, Semi-Supervised Anomaly Detection (SSAD), and unsupervised learning.
更多查看译文
关键词
semisupervised SVM,anomaly detection,semisupervised support vector machines,partially labelled data proliferation,large-scale complex systems,large imbalanced data,S3VMAD,unsupervised paradigm,convex objective function,global optimum solution,supervised support vector machines,unsupervised one-class SVM
AI 理解论文
溯源树
样例
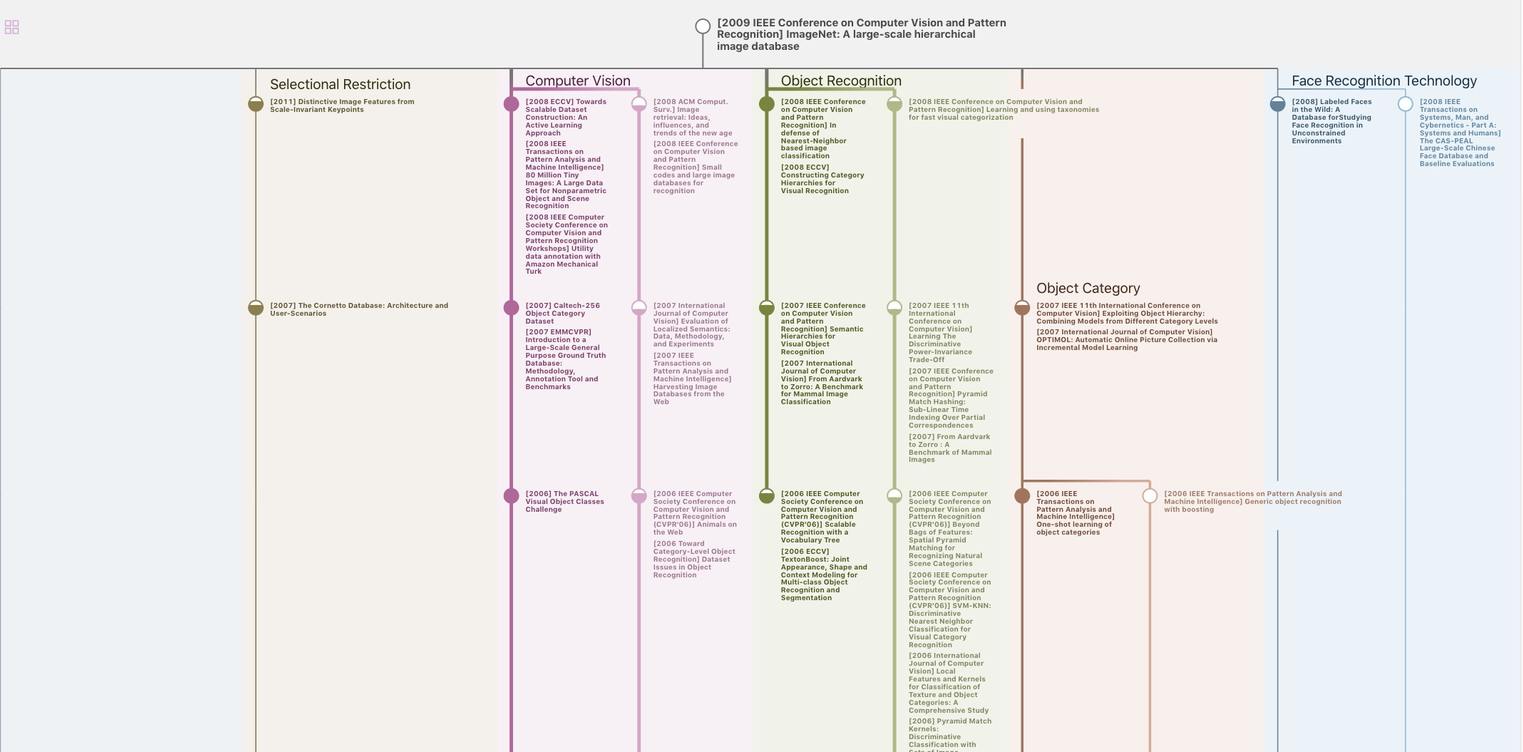
生成溯源树,研究论文发展脉络
Chat Paper
正在生成论文摘要