Multi-View Learning Based Robust Collimation Detection In Digital Radiographs
Medical Imaging: Image Processing(2014)
摘要
In X-ray examinations, it is essential that radiographers carefully use collimation to the appropriate anatomy of interest to minimize the overall integral dose to the patient. The shadow regions are not diagnostically meaningful and could impair the overall image quality. Thus, it is desirable to detect the collimation and exclude the shadow regions to optimize image display. However, due to the large variability of collimated images, collimation detection remains a challenging task. In this paper, we consider a region of interest (ROT) in an image, such as the collimation, can be described by two distinct views, a cluster of pixels within the ROT and the corners of the ROT. Based on this observation, we propose a robust multi-view learning based strategy for collimation detection in digital radiography. Specifically, one view is from random forests learning based region detector, which provides pixel-wise image classification and each pixel is labeled as either in-collimation or out-of-collimation. The other view is from a discriminative, learning-based landmark detector, which detects the corners and localizes the collimation within the image. Nevertheless, given the huge variability of the collimated images, the detection from either view alone may not be perfect. Therefore, we adopt an adaptive view fusing step to obtain the final detection by combining region and corner detection. We evaluate our algorithm in a database with 665 X-ray images in a wide variety of types and dosages and obtain a high detection accuracy (95%), compared with using region detector alone (87%) and landmark detector alone (83%).
更多查看译文
关键词
Collimation detection,multi-view learning,random forests,region detection,landmark detection
AI 理解论文
溯源树
样例
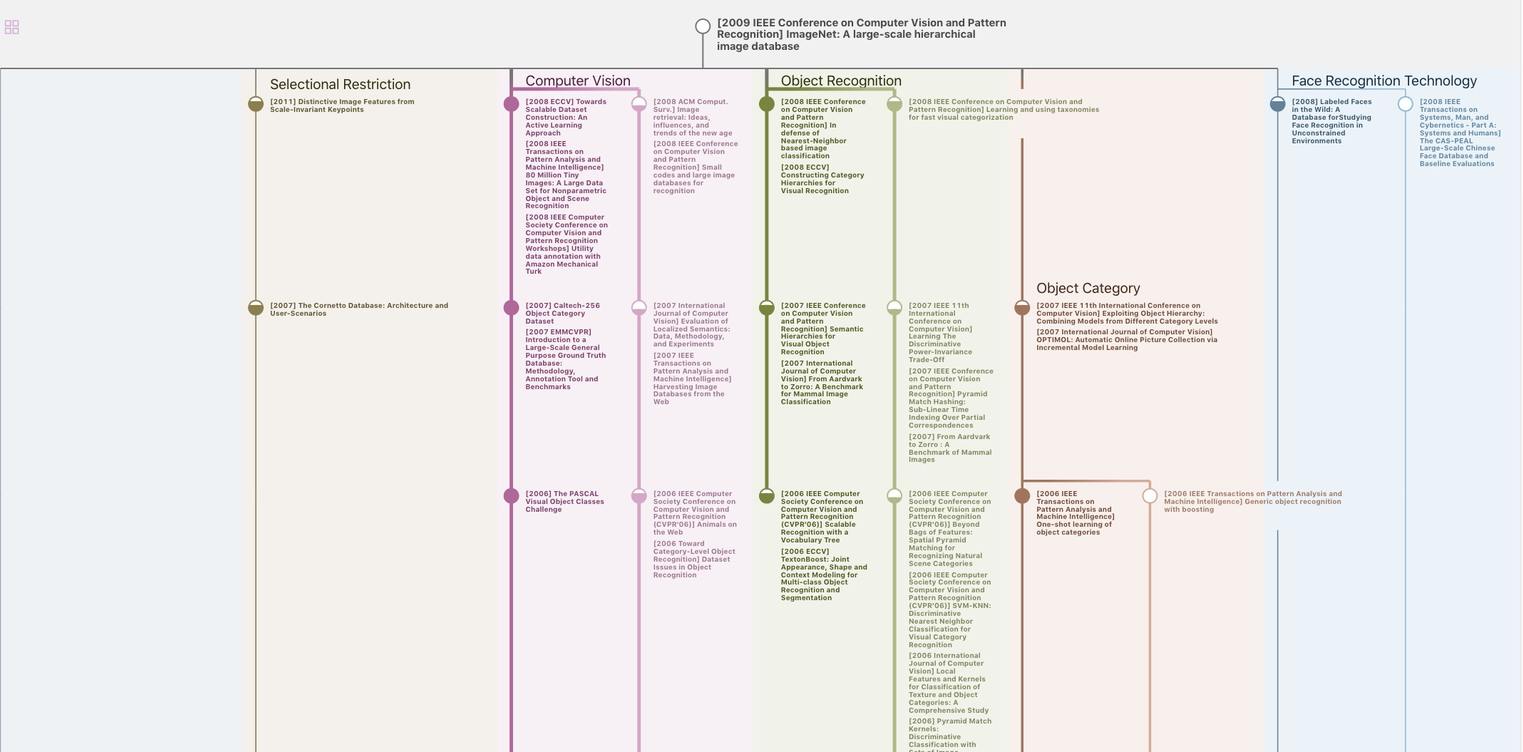
生成溯源树,研究论文发展脉络
Chat Paper
正在生成论文摘要