Brief Announcement: Certified Multiplicative Weights Update: Verified Learning Without Regret.
PODC(2017)
摘要
The Multiplicative Weights Update method (MWU) is a simple yet powerful algorithm for learning linear classifiers, for ensemble learning a la boosting, for approximately solving linear and semidefinite systems, for computing approximate solutions to multicommodity flow problems, and for online convex optimization, among other applications. In this brief announcement, we apply techniques from interactive theorem proving to define and prove correct the first formally verified implementation of MWU (specifically, we show that our MWU is no regret). Our primary application -- and one justification of the relevance of our work to the PODC community -- is to verified multi-agent systems, such as distributed multi-agent network flow and load balancing games, for which verified MWU provides a convenient method for distributed computation of approximate Coarse Correlated Equilibria.
更多查看译文
关键词
The Multiplicative Weights Update Method, Interactive Theorem Proving, Coq
AI 理解论文
溯源树
样例
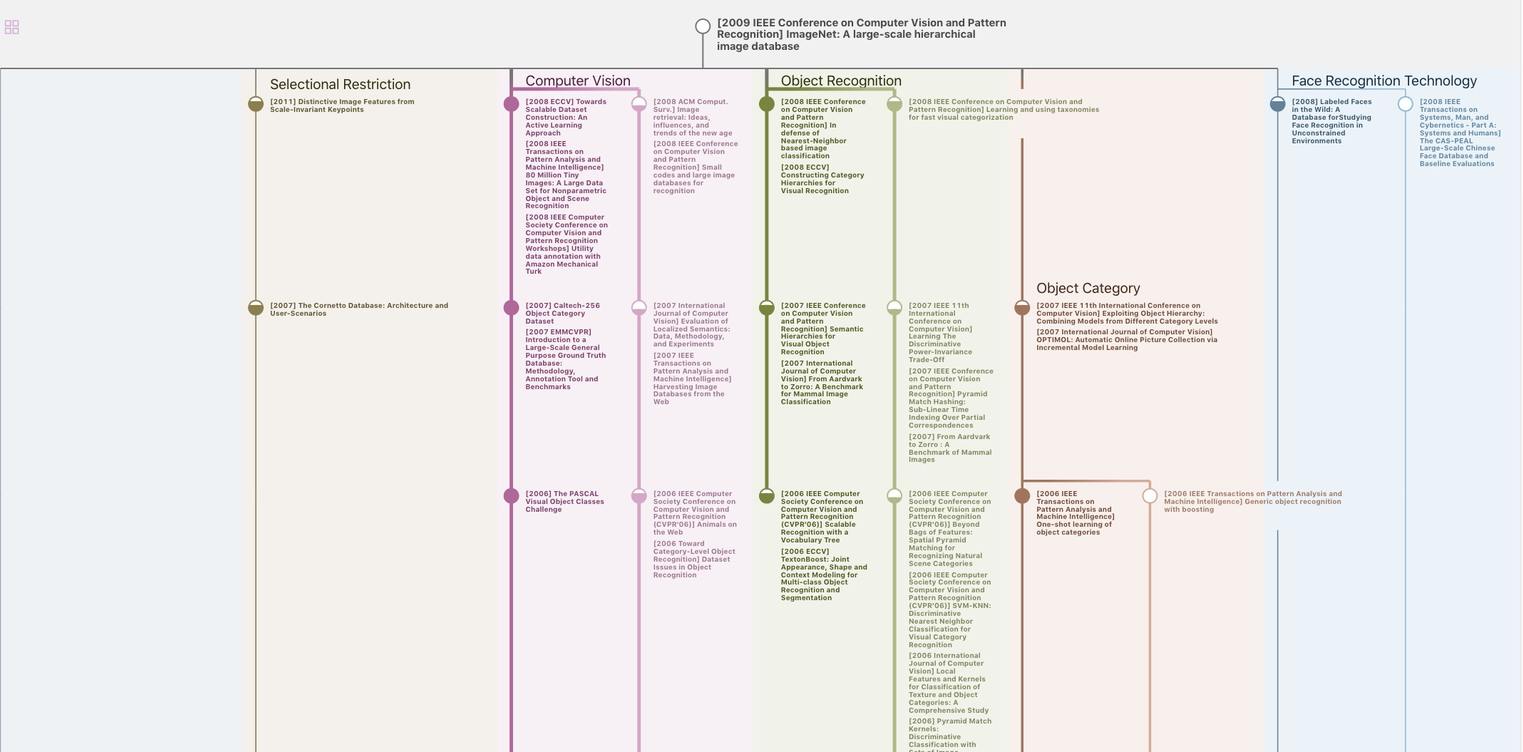
生成溯源树,研究论文发展脉络
Chat Paper
正在生成论文摘要