DeepSD: Supply-Demand Prediction for Online Car-Hailing Services Using Deep Neural Networks
2017 IEEE 33rd International Conference on Data Engineering (ICDE)(2017)
摘要
The online car-hailing service has gained great popularity all over the world. As more passengers and more drivers use the service, it becomes increasingly more important for the the car-hailing service providers to effectively schedule the drivers to minimize the waiting time of passengers and maximize the driver utilization, thus to improve the overall user experience. In this paper, we study the problem of predicting the real-time car-hailing supply-demand, which is one of the most important component of an effective scheduling system. Our objective is to predict the gap between the car-hailing supply and demand in a certain area in the next few minutes. Based on the prediction, we can balance the supply-demands by scheduling the drivers in advance. We present an end-to-end framework called Deep Supply-Demand (DeepSD) using a novel deep neural network structure. Our approach can automatically discover complicated supply-demand patterns from the car-hailing service data while only requires a minimal amount hand-crafted features. Moreover, our framework is highly flexible and extendable. Based on our framework, it is very easy to utilize multiple data sources (e.g., car-hailing orders, weather and traffic data) to achieve a high accuracy. We conduct extensive experimental evaluations, which show that our framework provides more accurate prediction results than the existing methods.
更多查看译文
关键词
traffic data source,weather data source,car-hailing orders,supply-demand pattern discovery,deep neural network structure,scheduling system,user experience improvement,driver utilization maximization,passenger waiting time minimization,online car-hailing service providers,deep-supply-demand prediction,DeepSD
AI 理解论文
溯源树
样例
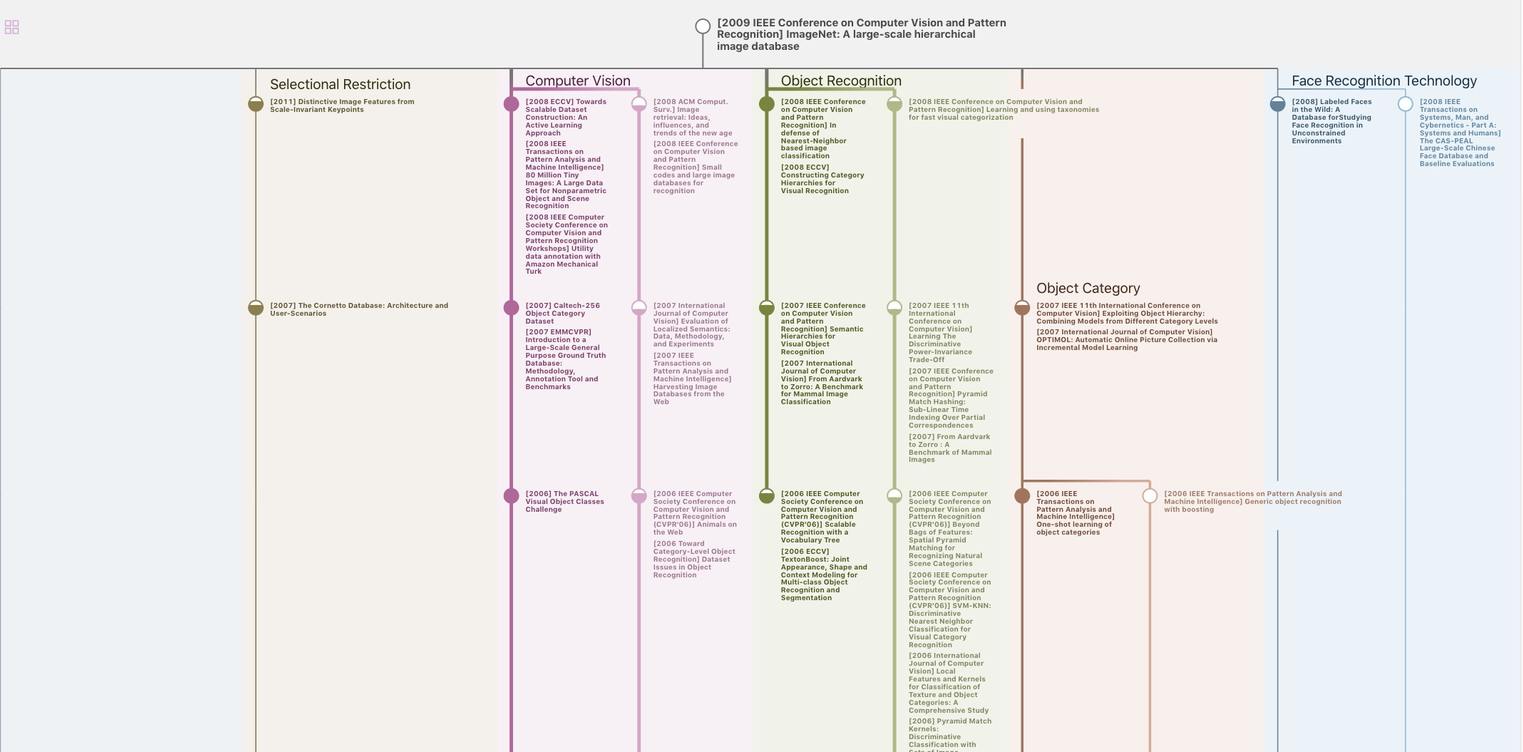
生成溯源树,研究论文发展脉络
Chat Paper
正在生成论文摘要