ACTS: An Active Learning Method for Time Series Classification
2017 IEEE 33rd International Conference on Data Engineering (ICDE)(2017)
摘要
Active learning has been widely used to select the most informative data for labeling in classification tasks, except for time series classification. The main challenge of active learning in time series classification is to evaluate the informativeness of a time series instance. Specifically, many informativeness metrics have been proposed for traditional active learning, however, none of them is particularly effective on time series data. In this paper, we design an informativeness metric that considers the characteristics of time series data in defining our instance uncertainty and utility. We prove that our informativeness metric is a submodular set function, and further develop an effective and efficient algorithm to select the most informative time series instances for training. In the experiment, we validate our method on a variety of datasets in the UCR Time Series Data Archive. The results show that our method achieves a higher classification accuracy than existing methods, using only 50% of the training instances.
更多查看译文
关键词
ACTS,active learning method,time series classification,informativeness metric,submodular set function,UCR Time Series Data Archive,uncertainty metric,utility metric
AI 理解论文
溯源树
样例
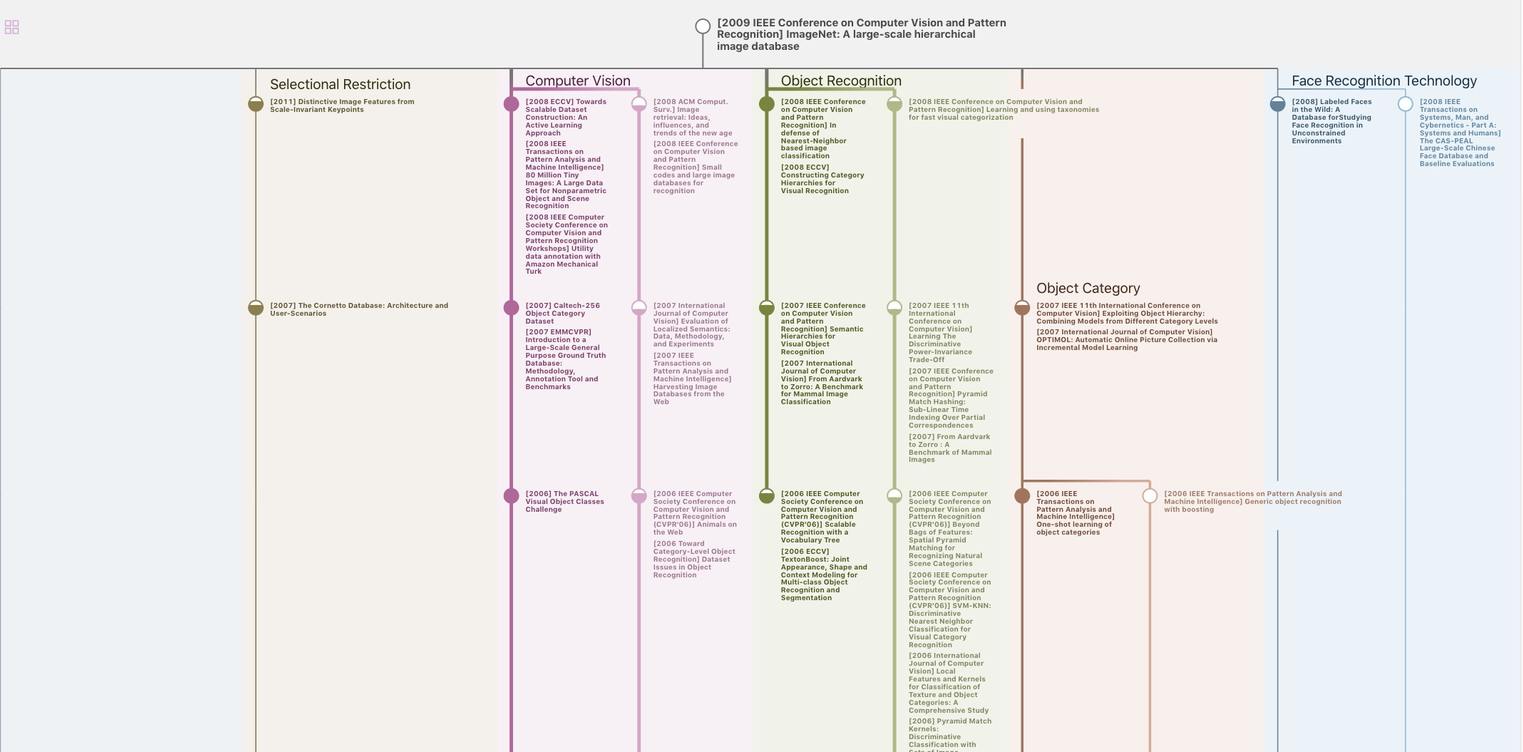
生成溯源树,研究论文发展脉络
Chat Paper
正在生成论文摘要