Link Prediction Across Aligned Networks With Sparse And Low Rank Matrix Estimation
2017 IEEE 33RD INTERNATIONAL CONFERENCE ON DATA ENGINEERING (ICDE 2017)(2017)
摘要
Users' addiction to online social networks is discovered to be highly correlated with their social connections in the networks. Dense social connections can effectively help online social networks retain their active users and improve the social network services. Therefore, it is of great importance to make a good prediction of the social links among users. Meanwhile, to enjoy more social network services, users nowadays are usually involved in multiple online social networks simultaneously. Formally, the social networks which share a number of common users are defined as the "aligned networks". With the information transferred from multiple aligned social networks, we can gain a more comprehensive knowledge about the social preferences of users in the pre-specified target network, which will benefit the social link prediction task greatly. However, when transferring the knowledge from other aligned source networks to the target network, there usually exists a shift in information distribution between different networks, namely domain difference. In this paper, we study the social link prediction problem of the target network, which is aligned with multiple social networks concurrently. To accommodate the domain difference issue, we project the features extracted for links from different aligned networks into a shared lower-dimensional feature space. Moreover, users in social networks usually tend to form communities and would only connect to a small number of users. Thus, the target network structure has both the low-rank and sparse properties. We propose a novel optimization framework, SLAMPRED, to combine both these two properties aforementioned of the target network and the information of multiple aligned networks with nice domain adaptations. Since the objective function is a linear combination of convex and concave functions involving non-differentiable regularizers, we propose a novel optimization method to iteratively solve it. Extensive experiments have been done on real-world aligned social networks, and the experimental results demonstrate the effectiveness of the proposed model.
更多查看译文
关键词
link prediction,aligned networks,low rank matrix estimation,online social networks,social connections,social network services,user social preference,information distribution,SLAMPRED framework,optimization method
AI 理解论文
溯源树
样例
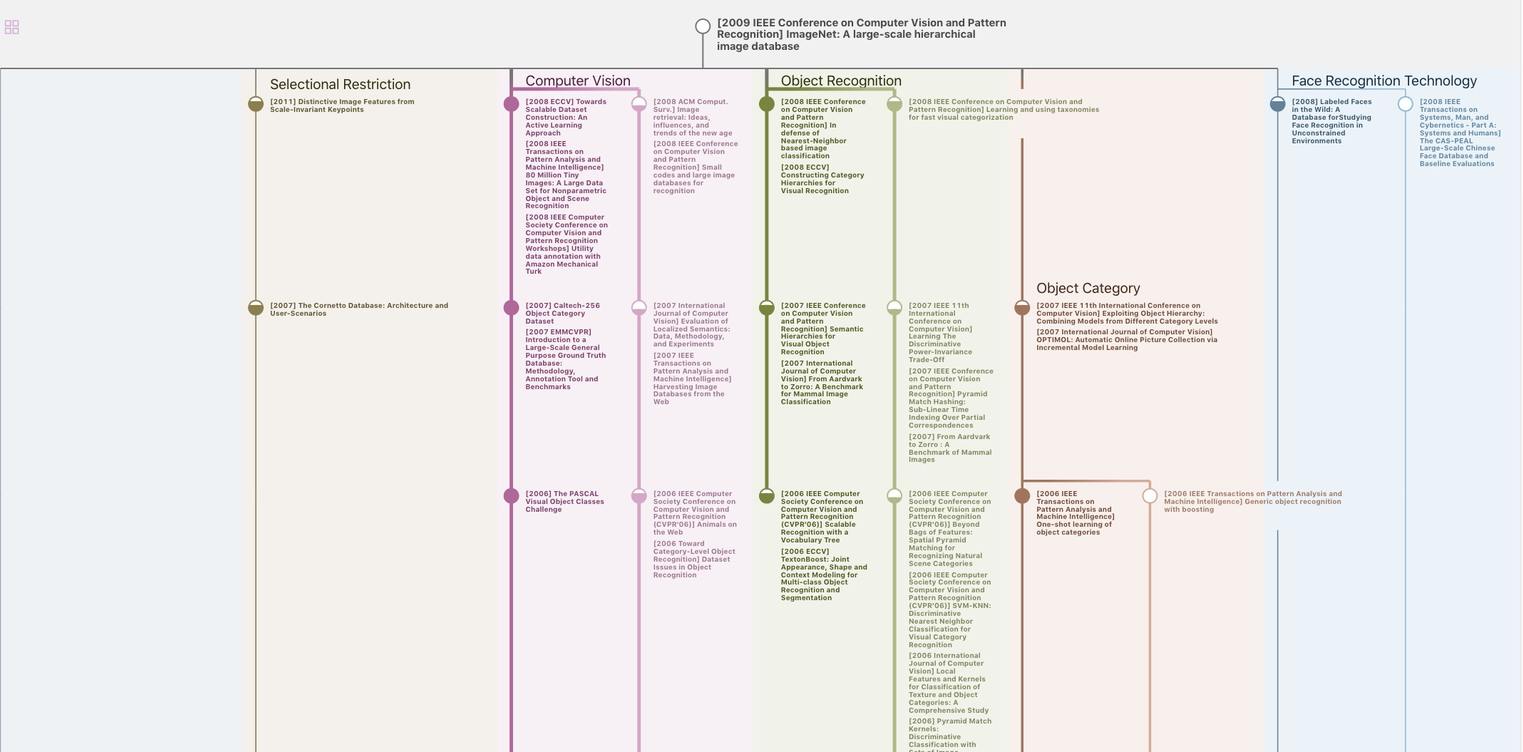
生成溯源树,研究论文发展脉络
Chat Paper
正在生成论文摘要