Crowdsourced Affinity: A Matter of Fact or Experience.
Lecture Notes in Computer Science(2017)
摘要
User-entity affinity is an essential component of many user-centric information systems such as online advertising, exploratory search, recommender system etc. The affinity is often assessed by analysing the interactions between users and entities within a data space. Among different affinity assessment techniques, content-based ones hypothesize that users have higher affinity with entities similar to the ones with which they had positive interactions in the past. Knowledge graph and folksonomy are respectively the milestones of Semantic Web and Social Web. Despite their shared crowdsourcing trait (not necessarily all knowledge graphs but some major large-scale ones), the encoded data are different in nature and structure. Knowledge graph encodes factual data with a formal ontology. Folksonomy encodes experience data with a loose structure. Many efforts have been made to make sense of folksonomy and to structure the community knowledge inside. Both data spaces allow to compute similarity between entities which can thereafter be used to calculate user-entity affinity. In this paper, we are interested in observing their comparative performance in the affinity assessment task. To this end, we carried out a first experiment within a travel destination recommendation scenario on a gold standard dataset. Our main findings are that knowledge graph helps to assess more accurately the affinity but folksonomy helps to increase the diversity and the novelty. This interesting complementarity motivated us to develop a semantic affinity framework to harvest the benefits of both data spaces. A second experiment with real users showed the utility of the proposed framework and confirmed our findings.
更多查看译文
关键词
Crowdsourcing,Affinity,Similarity,Semantic,Knowledge graph,Folksonomy,Travel,e-tourism,Semantic affinity framework,Diversity,Novelty
AI 理解论文
溯源树
样例
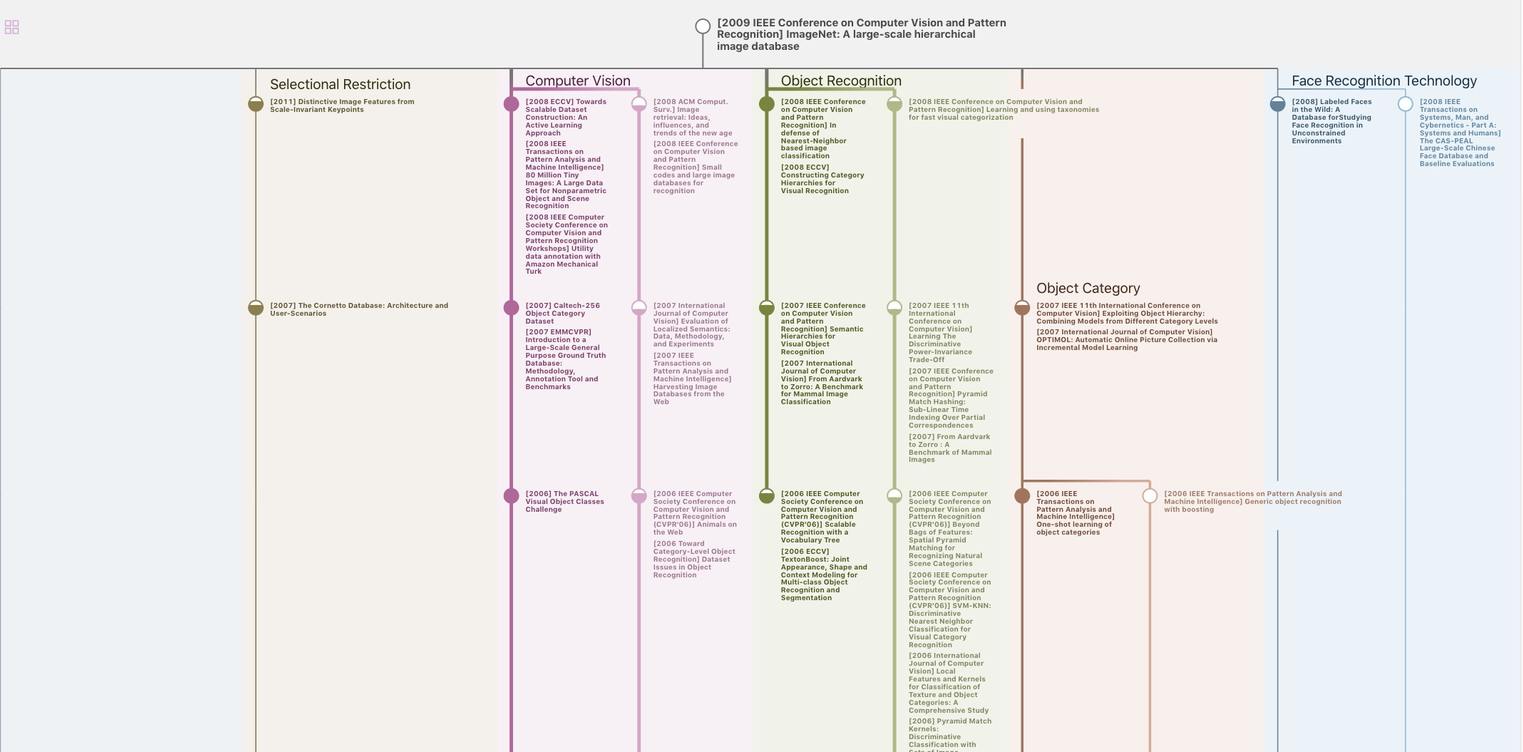
生成溯源树,研究论文发展脉络
Chat Paper
正在生成论文摘要