Extraction Of Temporal Events From Clinical Text Using Semi-Supervised Conditional Random Fields
DATA MINING AND BIG DATA, DMBD 2017(2017)
摘要
The huge amount of clinical text in Electronic Medical Records (EMRs) has opened a stage for text processing and information extraction for healthcare and medical research. Extracting temporal information in clinical text is much more difficult than the newswire text due to implicit expression of temporal information, domain-specific nature and lack of writing quality, among others. Despite of these constraints, some of the existing works established rule-based, machine learning and hybrid methods to extract temporal information with the help of annotated corpora. However obtaining the annotated corpora is costly, time consuming and requires much manual effort and thus their small size inevitably affects the processing quality. Motivated by this fact, in this work we propose a novel two-stage semi-supervised framework to exploit the abundant unannotated clinical text to automatically detect temporal events and then subsequently improve the stability and the accuracy of temporal event extraction. We trained and evaluated our semi-supervised model with the selected features of testing dataset, resulting F-measure of 89.76% for event extraction.
更多查看译文
关键词
Temporal event extraction, Electronic medical records, Clinical text, Natural language processing, Machine learning
AI 理解论文
溯源树
样例
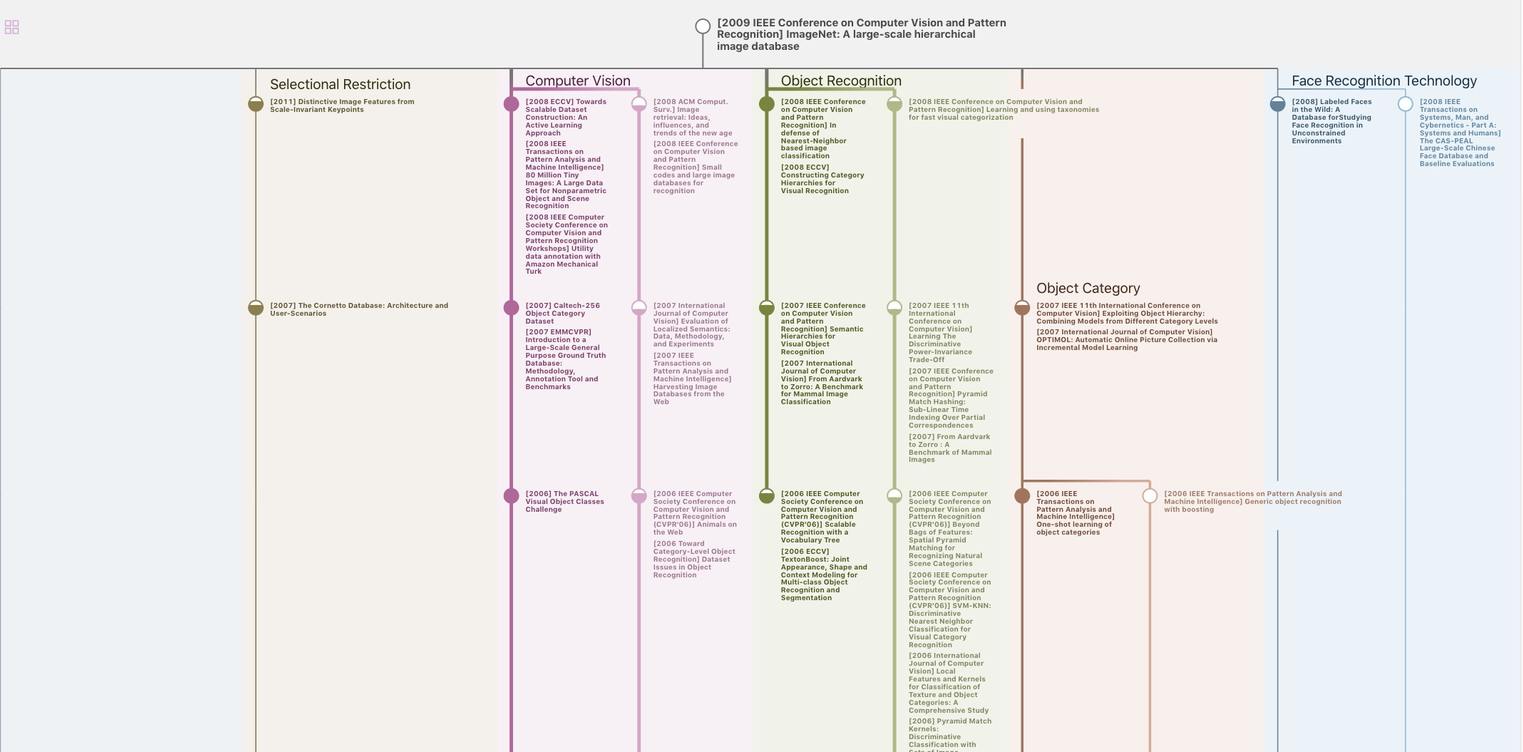
生成溯源树,研究论文发展脉络
Chat Paper
正在生成论文摘要