Learning to Represent Haptic Feedback for Partially-Observable Tasks
ICRA(2017)
摘要
The sense of touch, being the earliest sensory system to develop in a human body [1], plays a critical part of our daily interaction with the environment. In order to successfully complete a task, many manipulation interactions require incorporating haptic feedback. However, manually designing a feedback mechanism can be extremely challenging. In this work, we consider manipulation tasks that need to incorporate tactile sensor feedback in order to modify a provided nominal plan. To incorporate partial observation, we present a new framework that models the task as a partially observable Markov decision process (POMDP) and learns an appropriate representation of haptic feedback which can serve as the state for a POMDP model. The model, that is parametrized by deep recurrent neural networks, utilizes variational Bayes methods to optimize the approximate posterior. Finally, we build on deep Q-learning to be able to select the optimal action in each state without access to a simulator. We test our model on a PR2 robot for multiple tasks of turning a knob until it clicks.
更多查看译文
关键词
haptic feedback,partially-observable tasks,touch,sensory system,manipulation interactions,manipulation tasks,tactile sensor feedback,partially observable Markov decision process,POMDP,deep recurrent neural networks,variational Bayes methods,deep Q-learning
AI 理解论文
溯源树
样例
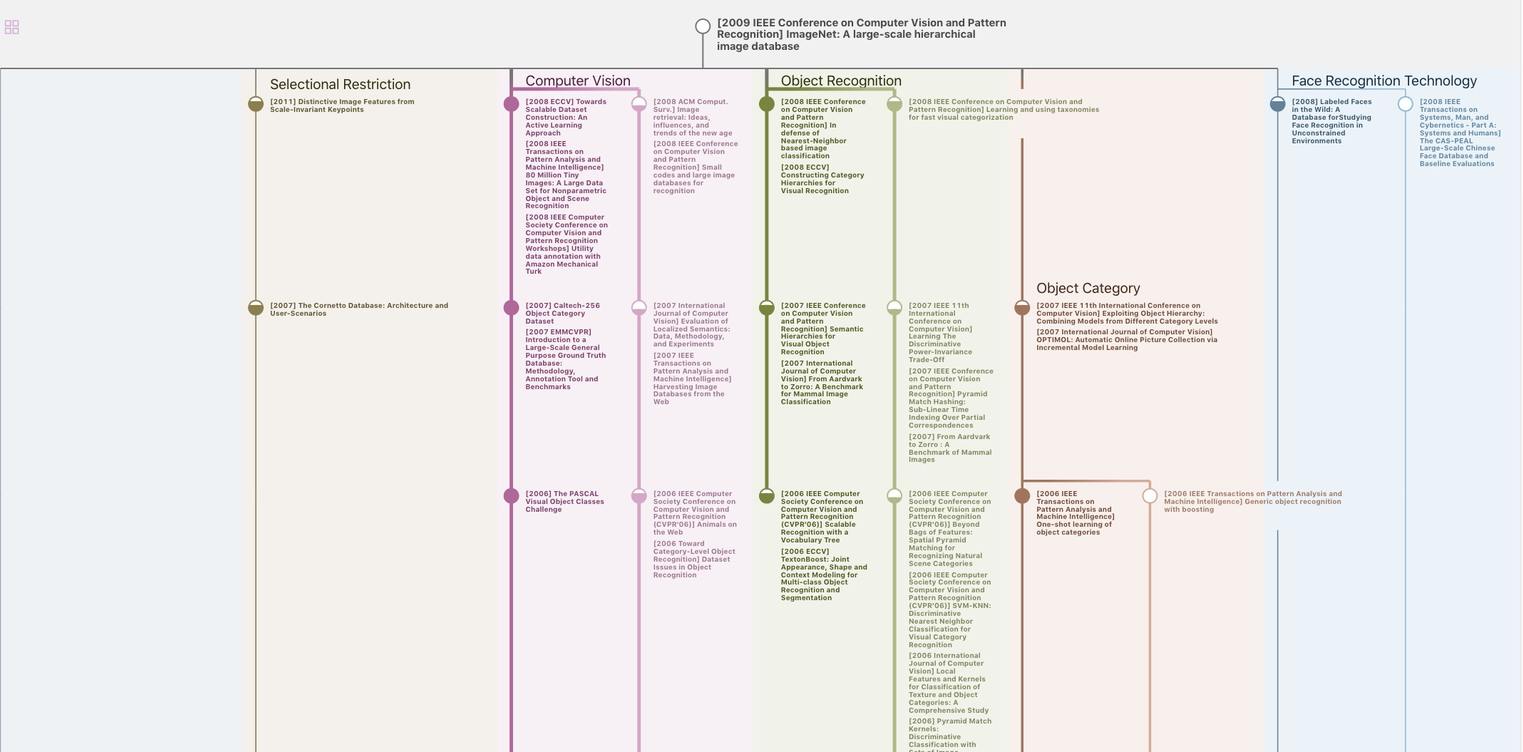
生成溯源树,研究论文发展脉络
Chat Paper
正在生成论文摘要