Physical symbol grounding and instance learning through demonstration and eye tracking.
ICRA(2017)
摘要
It is natural for humans to work with abstract plans which are often an intuitive and concise way to represent a task. However, high level task descriptions contain symbols and concepts which need to be grounded within the environment if the plan is to be executed by an autonomous robot. The problem of learning the mapping between abstract plan symbols and their physical instances in the environment is known as the problem of physical symbol grounding. In this paper, we propose a framework for Grounding and Learning Instances through Demonstration and Eye tracking (GLIDE). We associate traces of task demonstration to a sequence of fixations which we call fixation programs and exploit their properties in order to perform physical symbol grounding. We formulate the problem as a probabilistic generative model and present an algorithm for computationally feasible inference over the proposed model. A key aspect of our work is that we estimate fixation locations within the environment which enables the appearance of symbol instances to be learnt. Instance learning is a crucial ability when the robot does not have any knowledge about the model or the appearance of the symbols referred to in the plan instructions. We have conducted human experiments and demonstrate that GLIDE successfully grounds plan symbols and learns the appearance of their instances, thus enabling robots to autonomously execute tasks in initially unknown environments.
更多查看译文
关键词
physical symbol grounding,instance learning,high level task descriptions,autonomous robot,mapping learning,abstract plan symbols,grounding and learning instances through demonstration and eye tracking,GLIDE,fixation programs,probabilistic generative model,computationally feasible inference,fixation location estimation
AI 理解论文
溯源树
样例
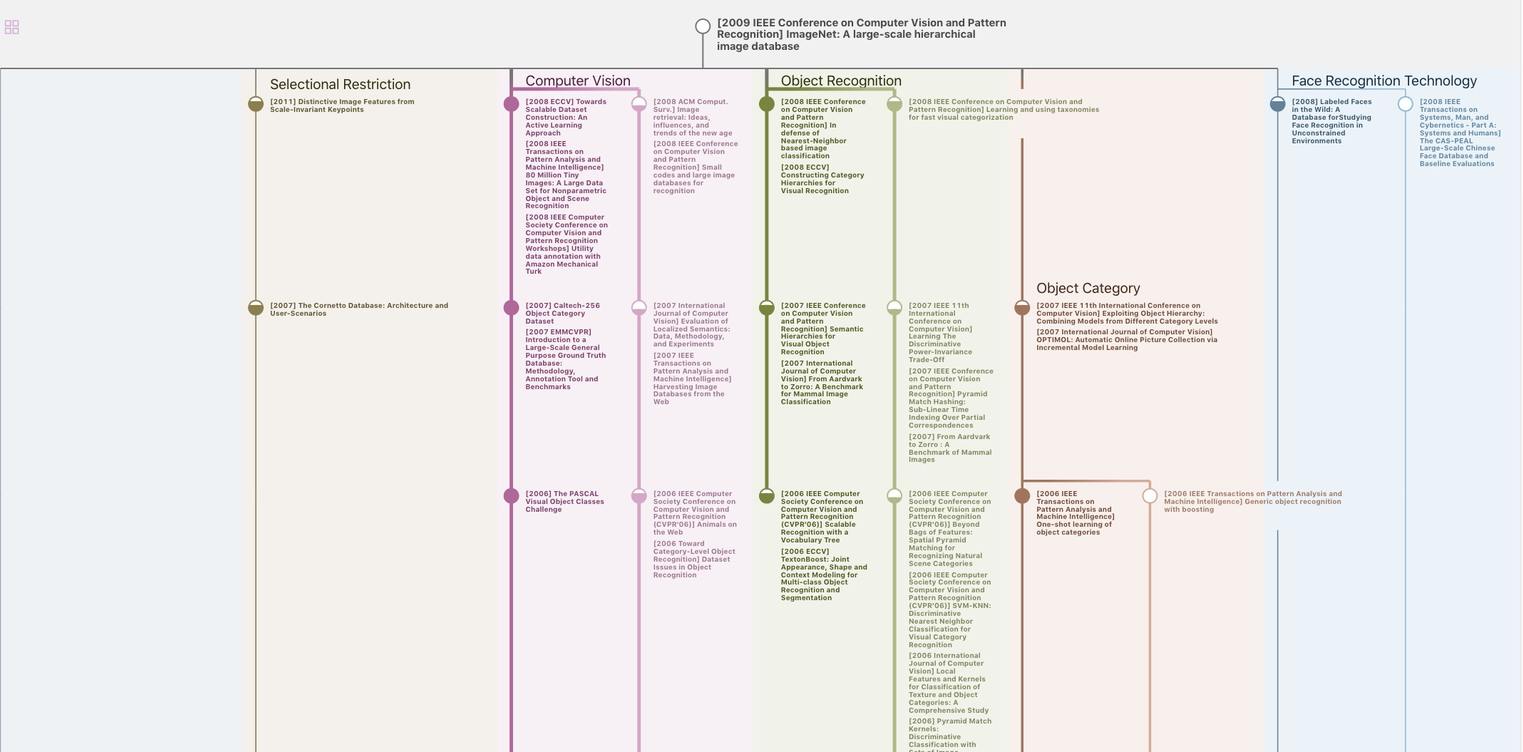
生成溯源树,研究论文发展脉络
Chat Paper
正在生成论文摘要