Sparse sampling for sensing temporal data — building an optimized envelope
2016 Conference on Technologies and Applications of Artificial Intelligence (TAAI)(2016)
摘要
IoT systems collect vast amounts of data which can be used in order to track and analyze the structure of future recorded data. However, due to limited computational power, bandwith, and storage capabilities, this data cannot be stored as is, but rather must be reduced in such a way so that the abilities to analyze future data, based on past data, will not be compromised. We propose a parameterized method of sampling the data in an optimal way. Our method has three parameters - an averaging method for constructing an average data cycle from past observations, an envelope method for defining an interval around the average data cycle, and an entropy method for comparing new data cycles to the constructed envelope. These parameters can be adjusted according to the nature of the data, in order to find the optimal representation for classifying new cycles as well as for identifying anomalies and predicting future cycle behavior. In this work we concentrate on finding the optimal envelope, given an averaging method and an entropy method. We demonstrate with a case study of meteorological data regarding El Ninio years.
更多查看译文
关键词
temporal data sensing,IoT systems,optimized envelope,recorded data structure analysis,recorded data structure tracking,averaging method,average data cycle,entropy method,optimal data representation,cycle classification,anomaly identification,future cycle behavior prediction,optimal envelope,meteorological data,El Ninio years,data storage capabilities,sparse data sampling
AI 理解论文
溯源树
样例
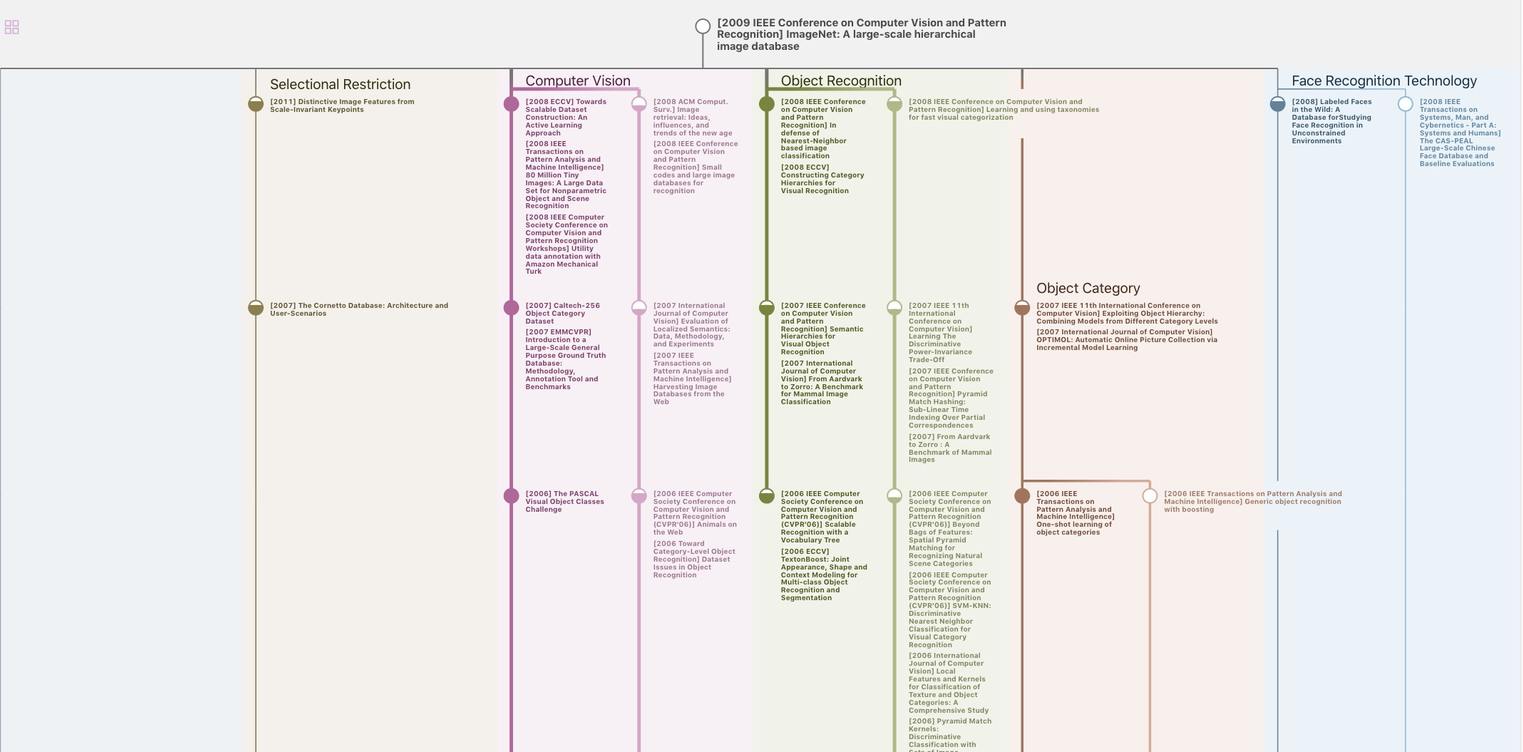
生成溯源树,研究论文发展脉络
Chat Paper
正在生成论文摘要