Strong NP-Hardness for Sparse Optimization with Concave Penalty Functions.
ICML(2017)
摘要
Consider the regularized sparse minimization problem, which involves empirical sums of loss functions for $n$ data points (each of dimension $d$) and a nonconvex sparsity penalty. We prove that finding an $mathcal{O}(n^{c_1}d^{c_2})$-optimal solution to the regularized sparse optimization problem is strongly NP-hard for any $c_1, c_2in [0,1)$ such that $c_1+c_2u003c1$. The result applies to a broad class of loss functions and sparse penalty functions. It suggests that one cannot even approximately solve the sparse optimization problem in polynomial time, unless P $=$ NP.
更多查看译文
AI 理解论文
溯源树
样例
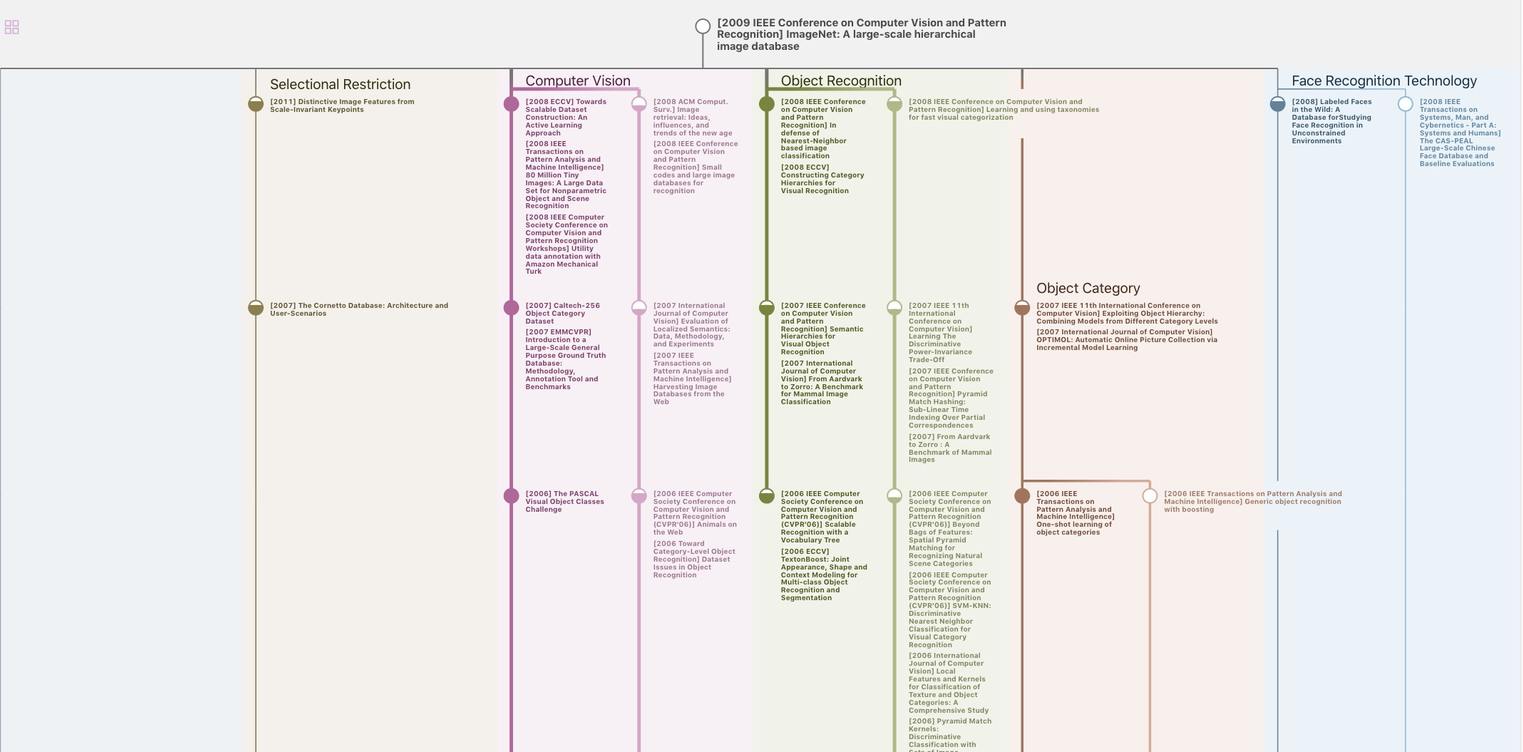
生成溯源树,研究论文发展脉络
Chat Paper
正在生成论文摘要