On Context-Dependent Clustering of Bandits.
ICML(2017)
摘要
We investigate a novel cluster-of-bandit algorithm CAB for collaborative recommendation tasks that implements the underlying feedback sharing mechanism by estimating the neighborhood of users in a context-dependent manner. CAB makes sharp departures from the state of the art by incorporating collaborative effects into inference as well as learning processes in a manner that seamlessly interleaving explore-exploit tradeoffs and collaborative steps. We prove regret bounds under various assumptions on the data, which exhibit a crisp dependence on the expected number of clusters over the users, a natural measure of the statistical difficulty of the learning task. Experiments on production and real-world datasets show that CAB offers significantly increased prediction performance against a representative pool of state-of-the-art methods.
更多查看译文
关键词
context-dependent
AI 理解论文
溯源树
样例
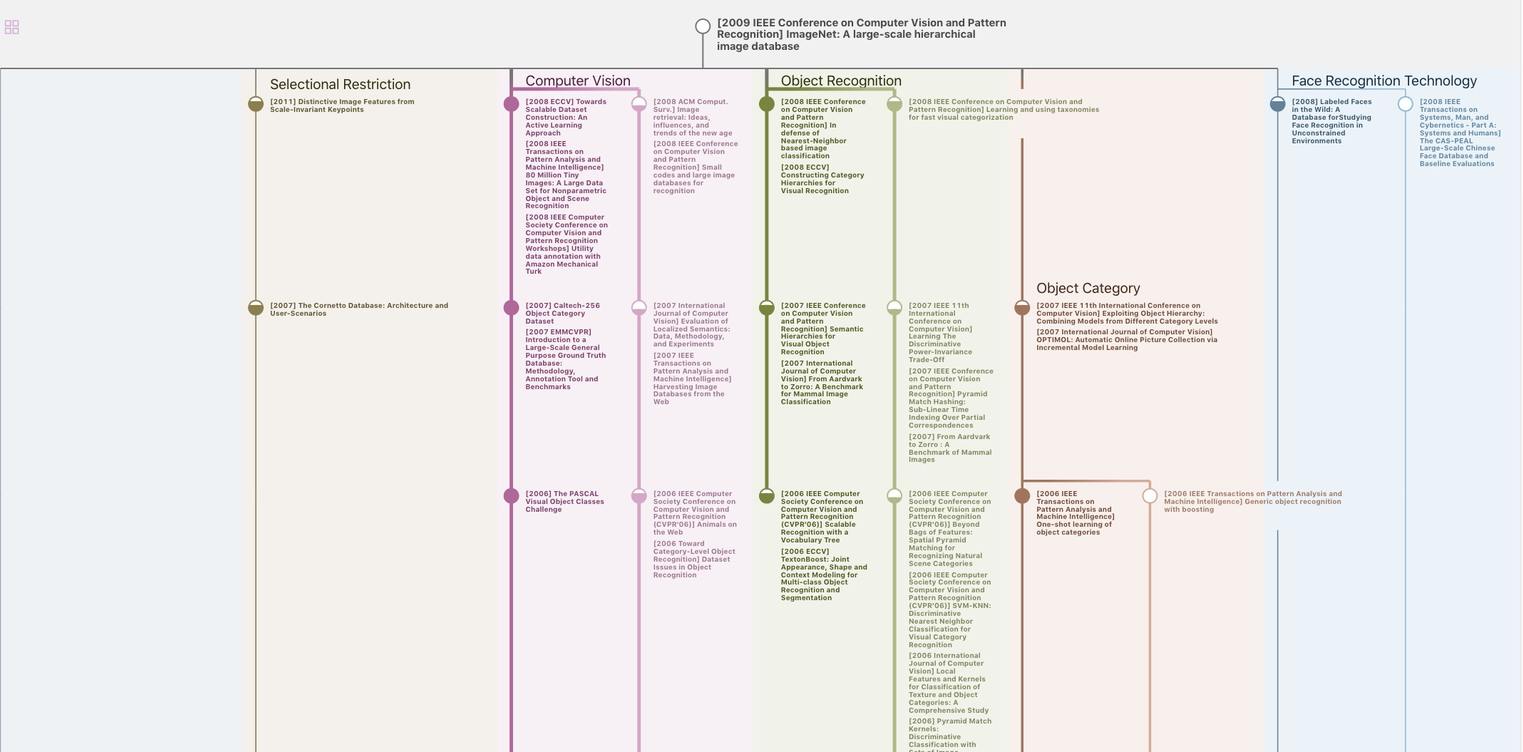
生成溯源树,研究论文发展脉络
Chat Paper
正在生成论文摘要