Detecting anomalous events in videos by learning deep representations of appearance and motion.
Computer Vision and Image Understanding(2017)
摘要
To the best of our knowledge, this paper represents the first attempt to address the anomalous event detection task using deep learning architectures. In this way, discriminative feature representations are automatically learned for the scene of interest, showing significant advantages over previous methods based on hand-crafted features.The proposed approach for learning feature representations combines appearance and motion information. Deep learning methods for fusing multiple modalities have been investigated in previous works. However, none of these works consider the problem of anomaly detection in intelligent video surveillance.A novel double fusion scheme is proposed to integrate appearance and motion deep repre- sentations for detecting unusual activities in video surveillance streams.We carried out an extensive evaluation of the proposed approach on three publicly available datasets, namely UCSD (Ped1 and Ped2), Subway and Train, and our approach yields very competitive performance with respect to state of the art methods. Anomalous event detection is of utmost importance in intelligent video surveillance. Currently, most approaches for the automatic analysis of complex video scenes typically rely on hand-crafted appearance and motion features. However, adopting user defined representations is clearly suboptimal, as it is desirable to learn descriptors specific to the scene of interest. To cope with this need, in this paper we propose Appearance and Motion DeepNet (AMDN), a novel approach based on deep neural networks to automatically learn feature representations. To exploit the complementary information of both appearance and motion patterns, we introduce a novel double fusion framework, combining the benefits of traditional early fusion and late fusion strategies. Specifically, stacked denoising autoencoders are proposed to separately learn both appearance and motion features as well as a joint representation (early fusion). Then, based on the learned features, multiple one-class SVM models are used to predict the anomaly scores of each input. Finally, a novel late fusion strategy is proposed to combine the computed scores and detect abnormal events. The proposed ADMN is extensively evaluated on publicly available video surveillance datasets including UCSD pedestian, Subway, and Train, showing competitive performance with respect to state of the art approaches.
更多查看译文
关键词
Video surveillance,Abnormal event detection,Unsupervised learning,Stacked denoising auto-encoders,Feature fusion
AI 理解论文
溯源树
样例
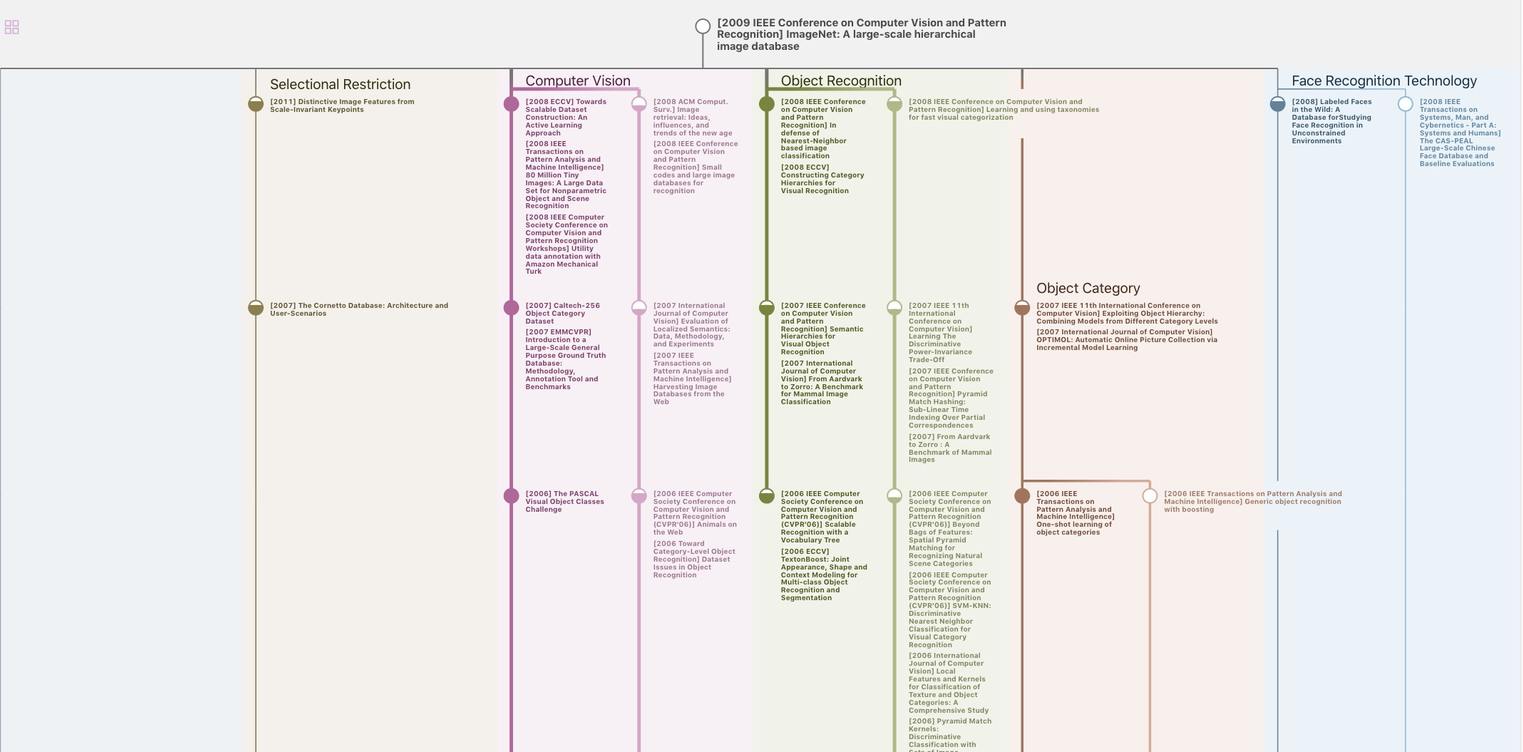
生成溯源树,研究论文发展脉络
Chat Paper
正在生成论文摘要