Blind Deconvolution With Model Discrepancies.
IEEE Trans. Image Processing(2017)
摘要
Blind deconvolution is a strongly ill-posed problem comprising of simultaneous blur and image estimation. Recent advances in prior modeling and/or inference methodology led to methods that started to perform reasonably well in real cases. However, as we show here, they tend to fail if the convolution model is violated even in a small part of the image. Methods based on variational Bayesian inference play a prominent role. In this paper, we use this inference in combination with the same prior for noise, image, and blur that belongs to the family of independent non-identical Gaussian distributions, known as the automatic relevance determination prior. We identify several important properties of this prior useful in blind deconvolution, namely, enforcing non-negativity of the blur kernel, favoring sharp images over blurred ones, and most importantly, handling non-Gaussian noise, which, as we demonstrate, is common in real scenarios. The presented method handles discrepancies in the convolution model, and thus extends applicability of blind deconvolution to real scenarios, such as photos blurred by camera motion and incorrect focus.
更多查看译文
关键词
Convolution,Deconvolution,Computational modeling,Kernel,Estimation,Bayes methods,Gaussian distribution
AI 理解论文
溯源树
样例
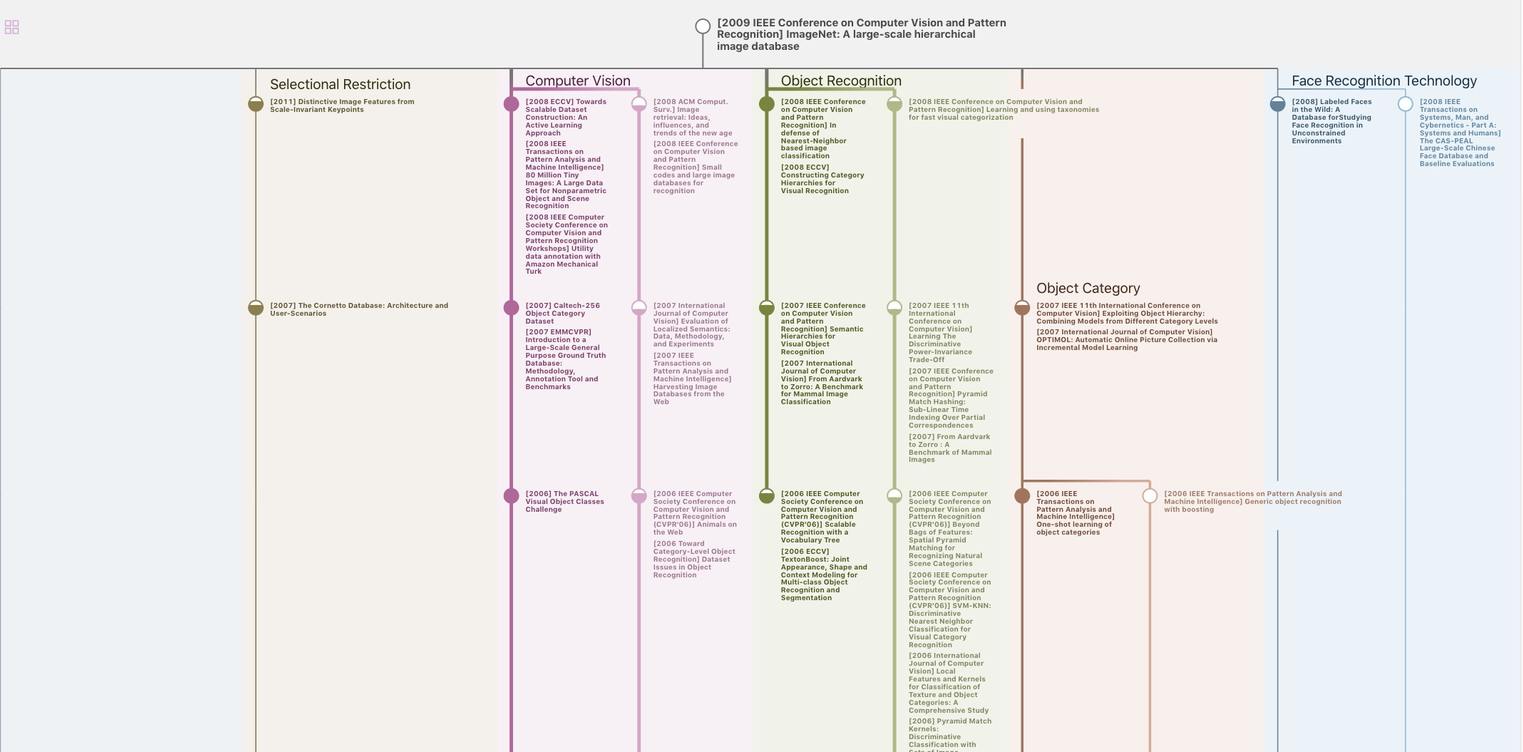
生成溯源树,研究论文发展脉络
Chat Paper
正在生成论文摘要