Reconstruction guarantee analysis of basis pursuit for binary measurement matrices in compressed sensing
IEEE Transactions on Information Theory(2017)
摘要
Recently, binary 0-1 measurement matrices, especially those from coding theory, were introduced to compressed sensing. Dimakis et al. found that the linear programming (LP) decoding of LDPC codes is very similar to the LP reconstruction of compressed sensing, and they further showed that the sparse binary parity-check matrices of good LDPC codes can be used as provably good measurement matrices for compressed sensing under basis pursuit (BP). Moreover, Khajehnejad et al. made use of girth to certify the good performances of sparse binary measurement matrices. In this paper, we examine the performance of binary measurement matrices with uniform column weight and arbitrary girth under BP. For a fixed measurement matrix, we first introduce a performance indicator wBPmin called minimum BP weight, and show that any k-sparse signals could be exactly recovered by BP if and only if k ≤ (wBPmin 1)/2. Then, lower bounds of wBPmin are studied. Borrowing ideas from the tree bound for the LDPC codes, we obtain several explicit lower bounds of wminBP , which improve on the previous results in some cases. These lower bounds also imply explicit ℓ1/ℓ1, ℓ2/ℓ1 and ℓ∞/ℓ1 sparse approximation guarantees, and further confirm that large girth has positive impacts on the performance of binary measurement matrices under BP. © 1963-2012 IEEE.
更多查看译文
关键词
basis pursuit,binary matrix,Compressed sensing,deterministic construction,girth,l1-minimization,LDPC codes,measurement matrix,minimum BP weight,reconstruction guarantee
AI 理解论文
溯源树
样例
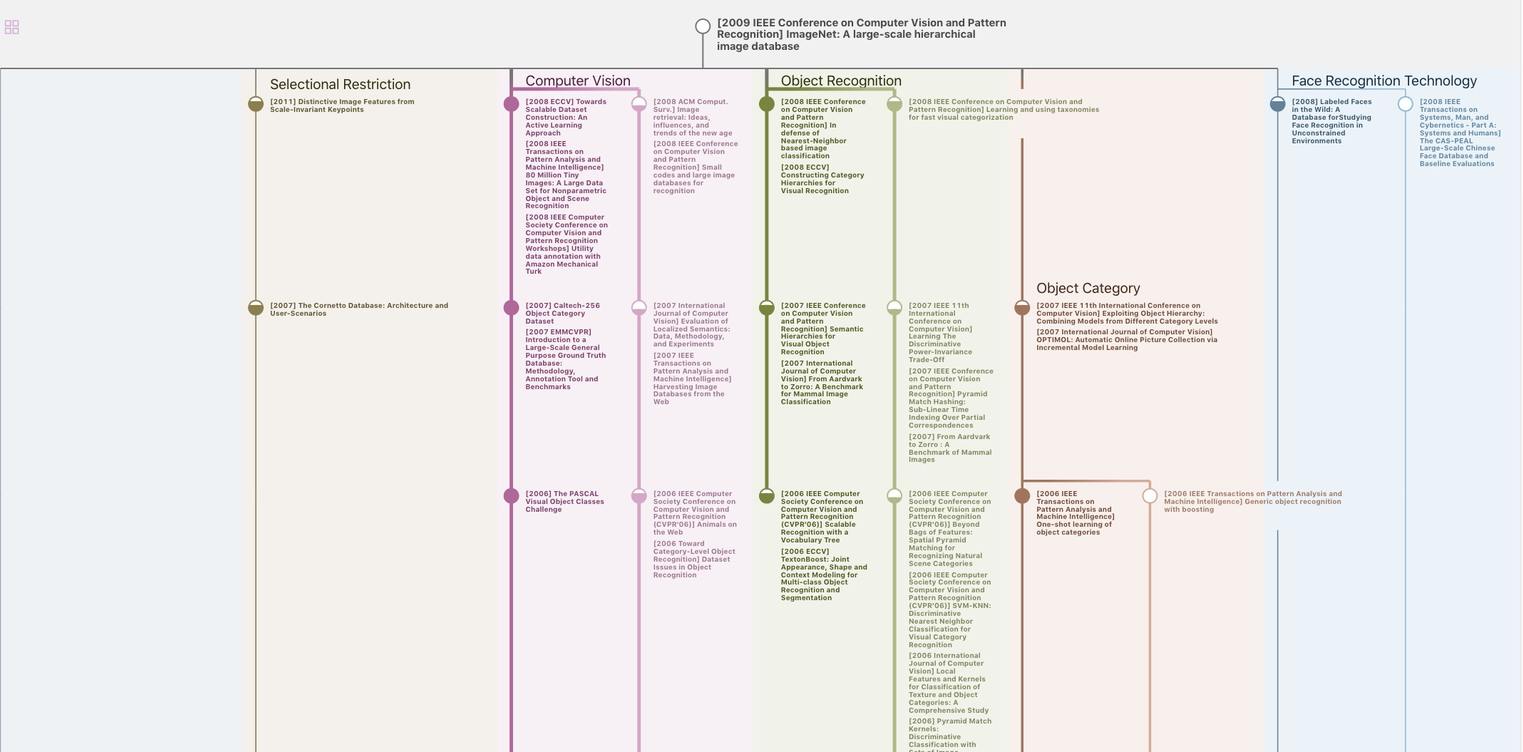
生成溯源树,研究论文发展脉络
Chat Paper
正在生成论文摘要