Salient motion detection using proximal robust principal component analysis method.
JOURNAL OF ELECTRONIC IMAGING(2017)
摘要
The recently proposed robust principal component analysis (RPCA) theory and its derived methods have attracted much attention in many computer vision and machine intelligence applications. From a wide view of these methods, independent motion objects are modeled as pixel-wised sparse or structurally sparse outliers from a highly correlated background signal, and all these methods are implemented under an l(1)-penalized optimization. Real data experiments reveal that even if l(1)-penalty is convex, the optimization sometimes cannot be satisfactorily solved, especially when the signal-to-noise ratio is relatively high. In addition, the unexpected background motion (e.g., periodic or stochastic motion) may also be included. We propose a moving object detection method based on a proximal RPCA along with saliency detection. Convex penalties including low-rank and sparse regularizations are substituted with proximal norms to achieve robust regression. After the foreground candidates have been extracted, a motion saliency map using spatiotemporal filtering is constructed. The foreground objects are then filtered out by dynamically adjusting the penalty parameter according to the corresponding saliency values. Evaluations on challenging video clips and qualitative and quantitative comparisons with several state-of-the-art methods demonstrate that the proposed approach works efficiently and robustly. (C) 2016 SPIE and IS&T
更多查看译文
关键词
moving object detection,saliency detection,proximal robust principal component analysis,nonconvex penalty
AI 理解论文
溯源树
样例
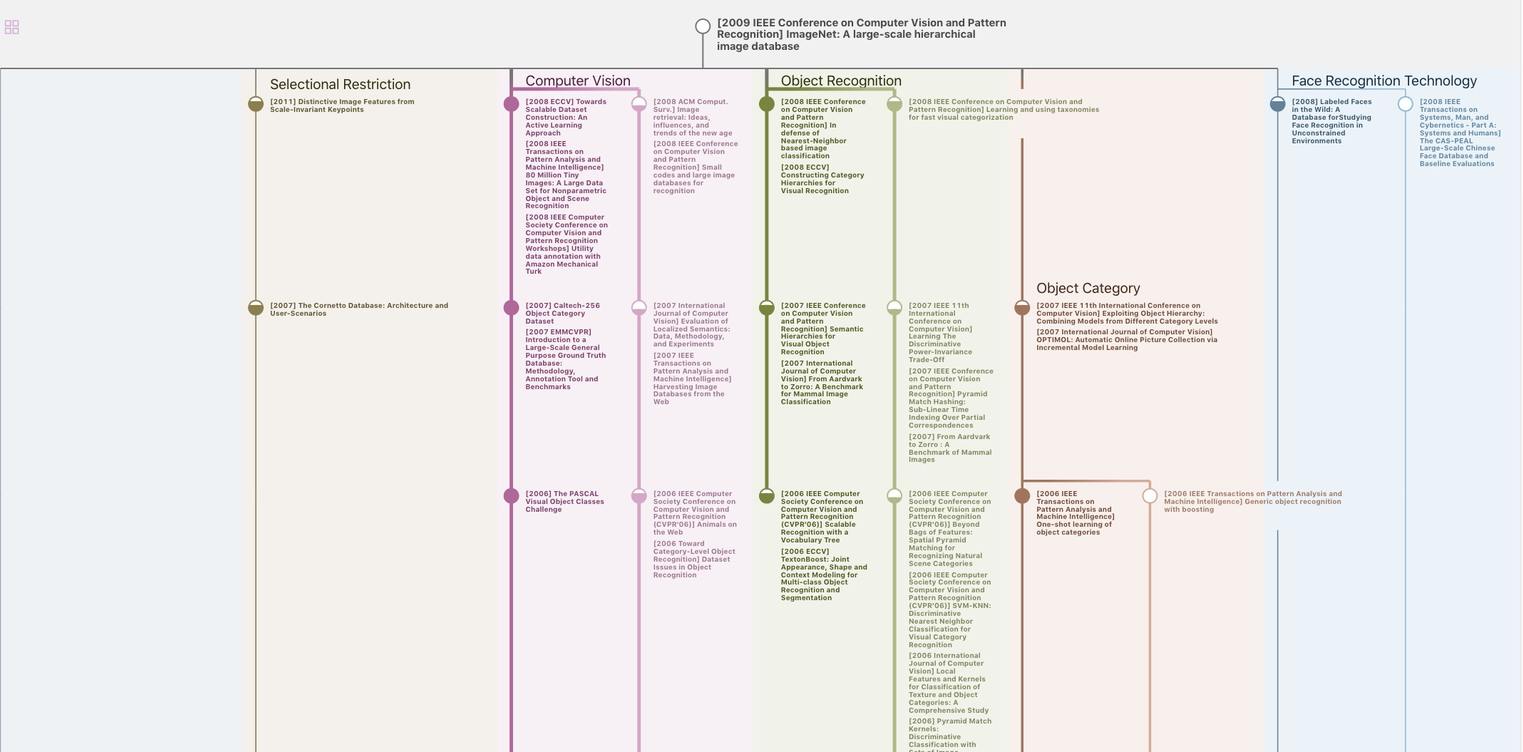
生成溯源树,研究论文发展脉络
Chat Paper
正在生成论文摘要