Dynamical Functional Theory for Compressed Sensing
2017 IEEE International Symposium on Information Theory (ISIT)(2017)
摘要
We introduce a theoretical approach for designing generalizations of the approximate message passing (AMP) algorithm for compressed sensing which are valid for large observation matrices that are drawn from an invariant random matrix ensemble. By design, the fixed points of the algorithm obey the Thouless-Anderson-Palmer (TAP) equations corresponding to the ensemble. Using a dynamical functional approach we are able to derive an effective stochastic process for the marginal statistics of a single component of the dynamics. This allows us to design memory terms in the algorithm in such a way that the resulting fields become Gaussian random variables allowing for an explicit analysis. The asymptotic statistics of these fields are consistent with the replica ansatz of the compressed sensing problem.
更多查看译文
关键词
dynamical functional theory,compressed sensing,designing generalizations,approximate message passing,AMP algorithm,observation matrices,invariant random matrix,Thouless-Anderson-Palmer equations,TAP equations,dynamical functional approach,effective stochastic process,Gaussian random variables
AI 理解论文
溯源树
样例
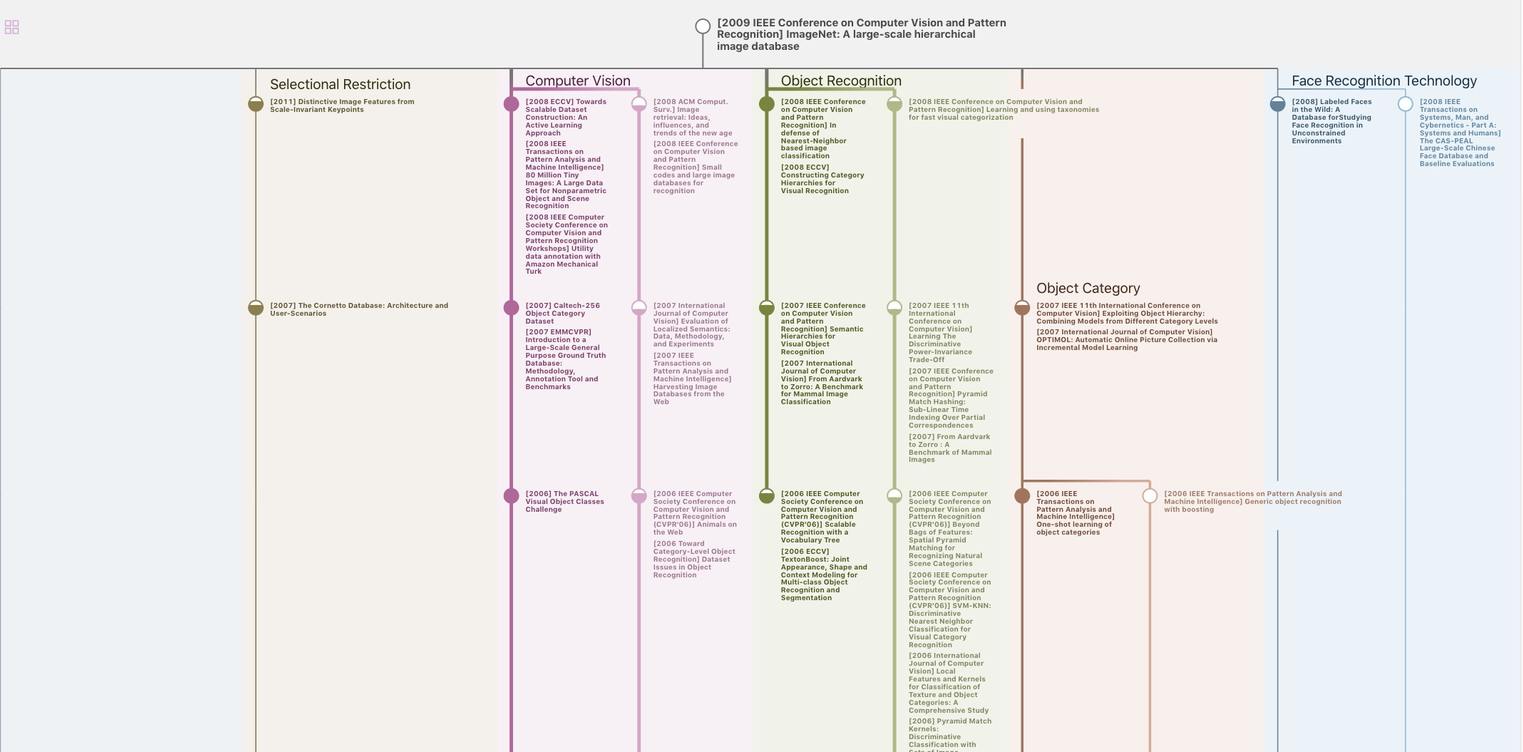
生成溯源树,研究论文发展脉络
Chat Paper
正在生成论文摘要