Multi-objective Bandits: Optimizing the Generalized Gini Index
ICML(2017)
摘要
We study the multi-armed bandit (MAB) problem where the agent receives a vectorial feedback that encodes many possibly competing objectives to be optimized. The goal of the agent is to find a policy, which can optimize these objectives simultaneously in a fair way. This multi-objective online optimization problem is formalized by using the Generalized Gini Index (GGI) aggregation function. We propose an online gradient descent algorithm which exploits the convexity of the GGI aggregation function, and controls the exploration in a careful way achieving a distribution-free regret (T^-1/2 ) with high probability. We test our algorithm on synthetic data as well as on an electric battery control problem where the goal is to trade off the use of the different cells of a battery in order to balance their respective degradation rates.
更多查看译文
关键词
index,multi-objective
AI 理解论文
溯源树
样例
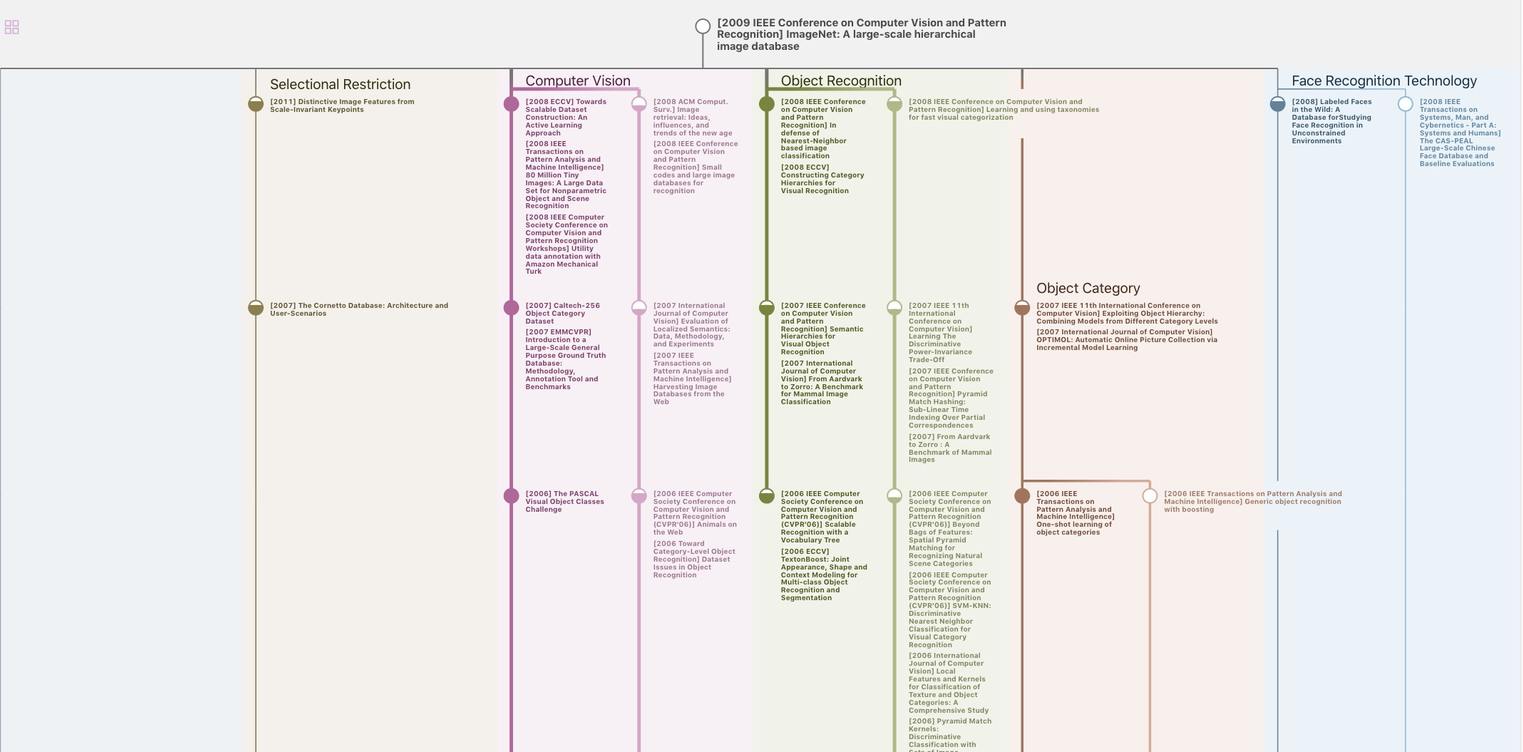
生成溯源树,研究论文发展脉络
Chat Paper
正在生成论文摘要