Metric Learning for Generalizing Spatial Relations to New Objects
2017 IEEE/RSJ International Conference on Intelligent Robots and Systems (IROS)(2017)
摘要
Human-centered environments are rich with a wide variety of spatial relations between everyday objects. For autonomous robots to operate effectively in such environments, they should be able to reason about these relations and generalize them to objects with different shapes and sizes. For example, having learned to place a toy inside a basket, a robot should be able to generalize this concept using a spoon and a cup. This requires a robot to have the flexibility to learn arbitrary relations in a lifelong manner, making it challenging for an expert to pre-program it with sufficient knowledge to do so beforehand. In this paper, we address the problem of learning spatial relations by introducing a novel method from the perspective of distance metric learning. Our approach enables a robot to reason about the similarity between pairwise spatial relations, thereby enabling it to use its previous knowledge when presented with a new relation to imitate. We show how this makes it possible to learn arbitrary spatial relations from non-expert users using a small number of examples and in an interactive manner. Our extensive evaluation with real-world data demonstrates the effectiveness of our method in reasoning about a continuous spectrum of spatial relations and generalizing them to new objects.
更多查看译文
关键词
spatial relations generalization,human-centered environments,arbitrary spatial relation learning,continuous spectrum,pairwise spatial relations,distance metric learning,arbitrary relations,autonomous robots
AI 理解论文
溯源树
样例
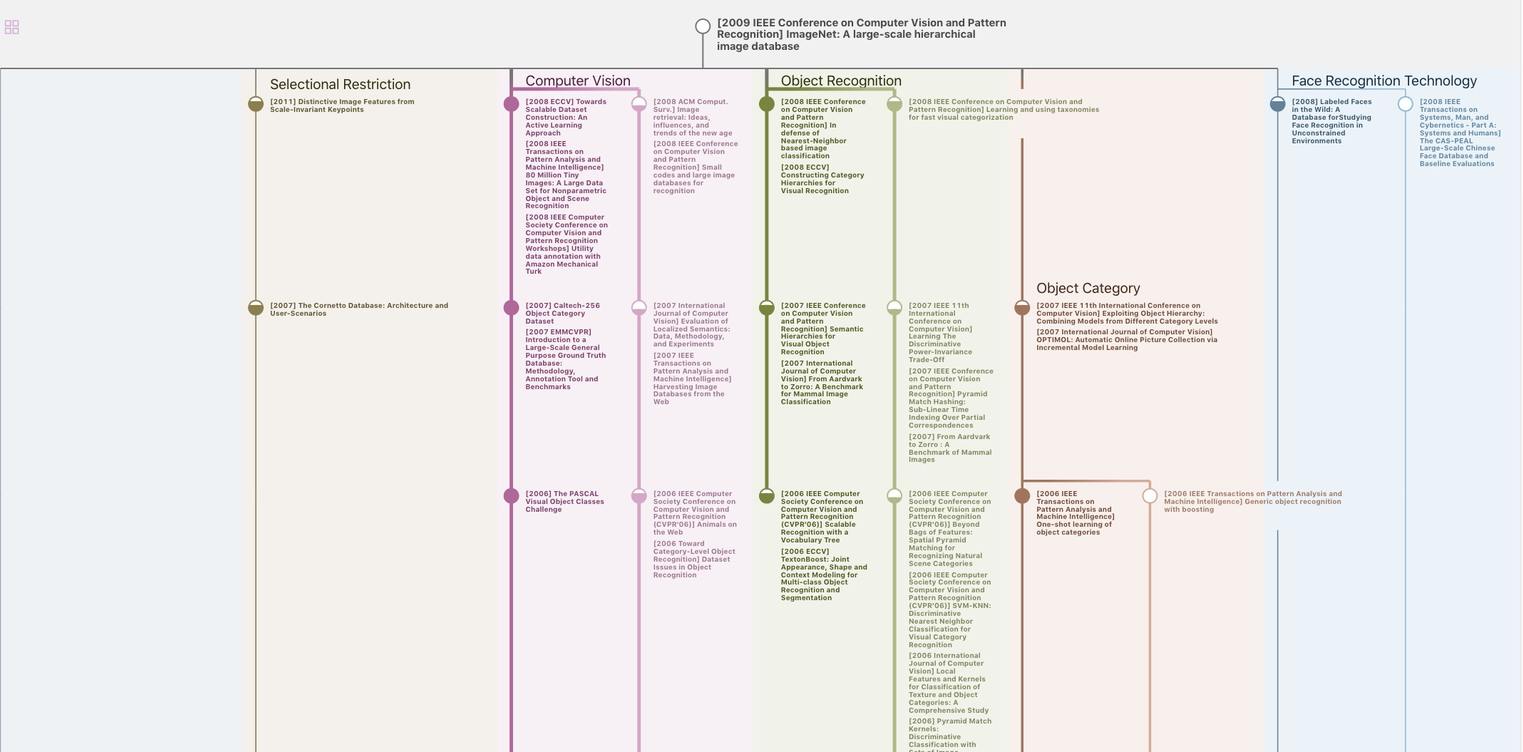
生成溯源树,研究论文发展脉络
Chat Paper
正在生成论文摘要