Leverage triple relational structures via low-rank feature reduction for multi-output regression
Multimedia Tools Appl.(2016)
摘要
Multi-output regression aims at learning a mapping from feature variables to multiple output variables. It is significant to utilize variety of inherent relational structure information of observations to conduct multi-output regression task when learning a best mapping from high-dimensional data. In this paper, we propose a new multi-output regression method, which simultaneously takes advantage of the low-rank constraint, sample selection, and feature selection in a unified framework. We first take the effect of low-rank constraint to search the correlation of output variables and impose ℓ 2, p -norm regularization on the coefficient matrix to capture the correlation between features and outputs. And then, the ℓ 2, p -norm on the loss function is designed to discover the correlation between samples, so as to select those informative samples to learn the model for improving predictive capacity. Thirdly, orthogonal subspace learning is exploited to ensure multi-output variables share the same low-rank structure of data by rotating the results of feature selection. In addition, to get the optimal solution of the objective function, we propose an effective iterative optimization algorithm. Finally, we conduct sets of experimental results on real datasets, and show the proposed method outperforms the state-of-the-art methods in terms of aCC and aRMSE.
更多查看译文
关键词
Multi-output regression, Low-rank regression, Feature selection, Orthogonal subspace learning
AI 理解论文
溯源树
样例
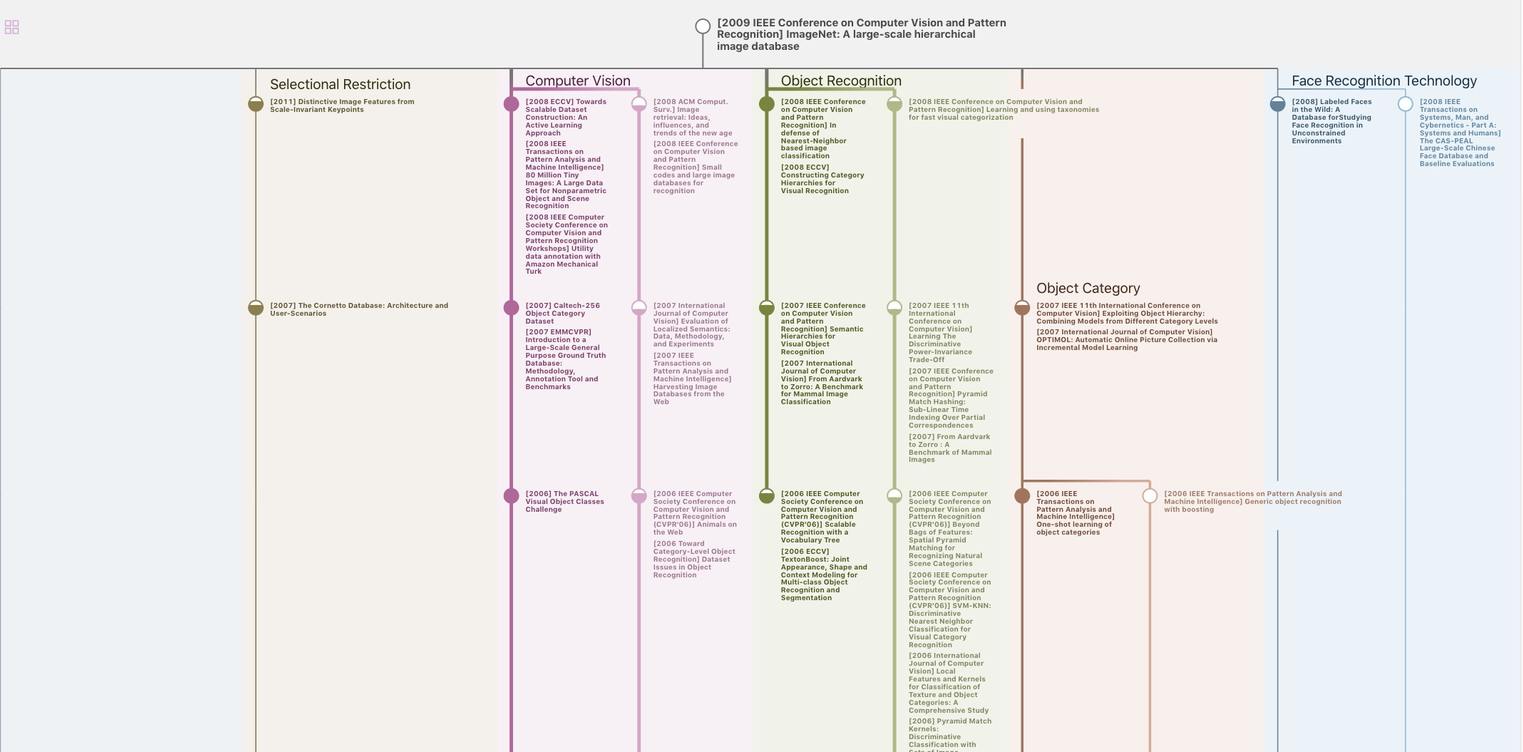
生成溯源树,研究论文发展脉络
Chat Paper
正在生成论文摘要