Multimodal learning for topic sentiment analysis in microblogging.
Neurocomputing(2017)
摘要
Microblogging has become a widely-spread platform of human communication. The massive amount of opinion-rich data in microblogging is helpful to analyze and manage public opinion and social emotion. Different from traditional texts, microblogging data are multimodal, containing multifarious data such as emoticons, image, etc. Most existing sentiment and topic detection approaches treat the unique microblogging data as noise. However, this may lead to unsatisfactoriness in sentiment classification and topic identification. In order to address the issue, we propose a multimodal joint sentiment topic model (MJST) for weakly supervised sentiment analysis in microblogging, which applies latent Dirichlet allocation (LDA) to simultaneously analyze sentiment and topic hidden in messages based the introduction of emoticons and microbloggers personality. Extensive experiments show that MJST outperforms state-of-the-art unsupervised approaches JST, SLDA and DPLDA significantly in terms of sentiment classification accuracy and is promising.
更多查看译文
关键词
Sentiment analysis,Microblogging,Latent dirichlet allocation (LDA)
AI 理解论文
溯源树
样例
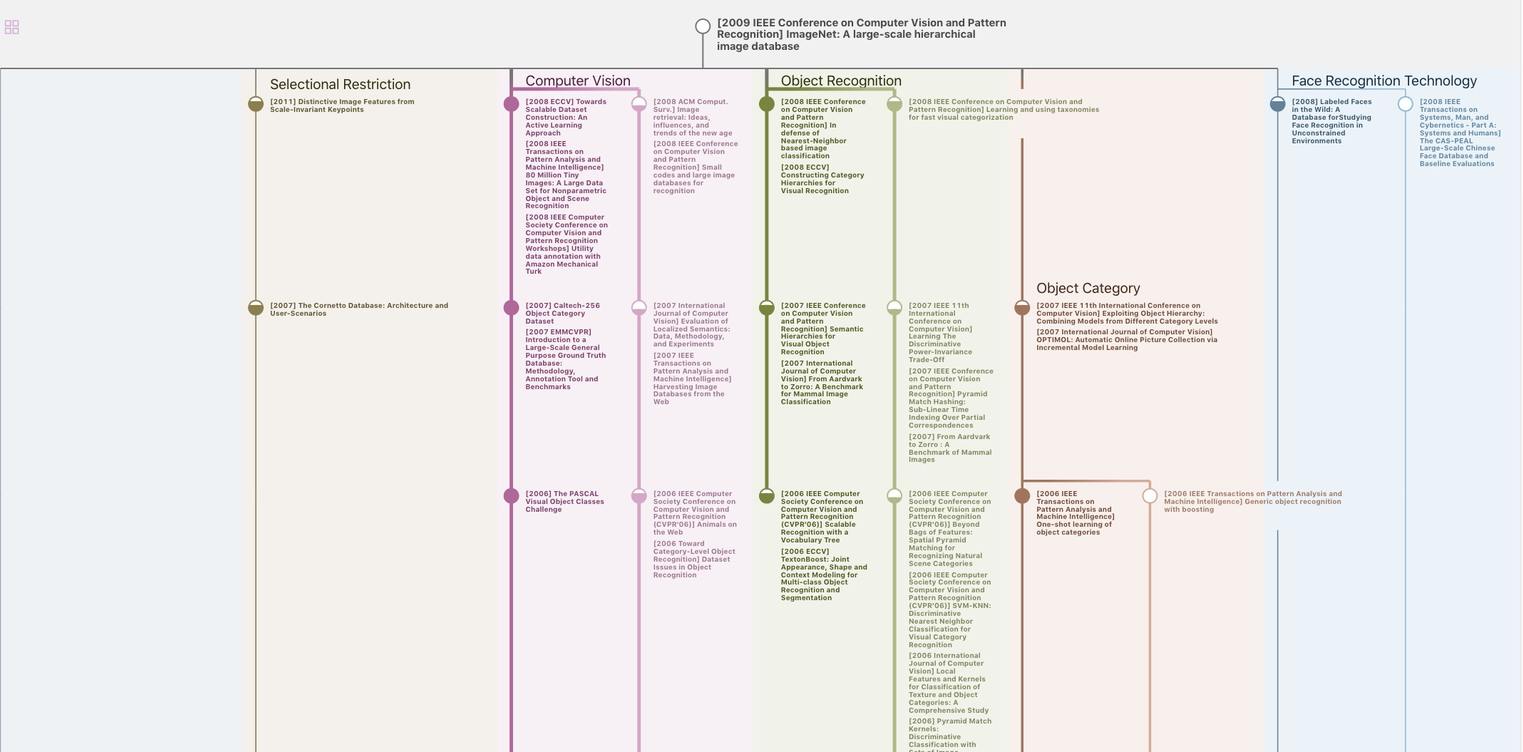
生成溯源树,研究论文发展脉络
Chat Paper
正在生成论文摘要