Mapping fine-scale population distributions at the building level by integrating multisource geospatial big data.
International Journal of Geographical Information Science(2017)
摘要
Fine-scale population distribution data at the building level play an essential role in numerous fields, for example urban planning and disaster prevention. The rapid technological development of remote sensing RS and geographical information system GIS in recent decades has benefited numerous population distribution mapping studies. However, most of these studies focused on global population and environmental changes; few considered fine-scale population mapping at the local scale, largely because of a lack of reliable data and models. As geospatial big data booms, Internet-collected volunteered geographic information VGI can now be used to solve this problem. This article establishes a novel framework to map urban population distributions at the building scale by integrating multisource geospatial big data, which is essential for the fine-scale mapping of population distributions. First, Baidu points-of-interest POIs and real-time Tencent user densities RTUD are analyzed by using a random forest algorithm to down-scale the street-level population distribution to the grid level. Then, we design an effective iterative building-population gravity model to map population distributions at the building level. Meanwhile, we introduce a densely inhabited index DII, generated by the proposed gravity model, which can be used to estimate the degree of residential crowding. According to a comparison with official community-level census data and the results of previous population mapping methods, our method exhibits the best accuracy Pearson R = .8615, RMSE = 663.3250, p
更多查看译文
关键词
Population mapping, social sensing, geospatial big data, gravity model, random forest
AI 理解论文
溯源树
样例
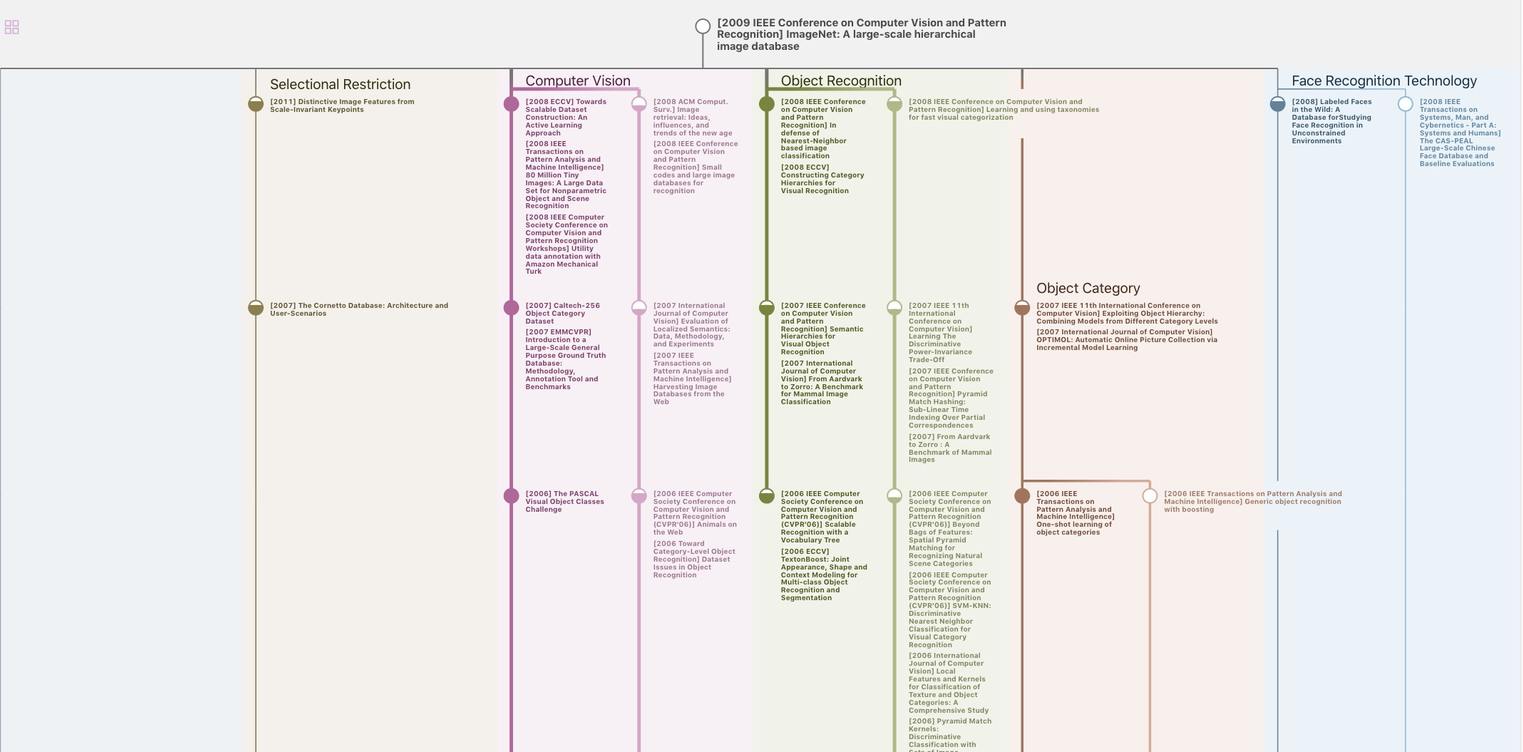
生成溯源树,研究论文发展脉络
Chat Paper
正在生成论文摘要