Self-organizing Neural Network for Adaptive Operator Selection in Evolutionary Search.
Lecture Notes in Computer Science(2016)
摘要
Evolutionary Algorithm is a well-known meta-heuristics para-digm capable of providing high-quality solutions to computationally hard problems. As with the other meta-heuristics, its performance is often attributed to appropriate design choices such as the choice of crossover operators and some other parameters. In this chapter, we propose a continuous state Markov Decision Process model to select crossover operators based on the states during evolutionary search. We propose to find the operator selection policy efficiently using a self-organizing neural network, which is trained offline using randomly selected training samples. The trained neural network is then verified on test instances not used for generating the training samples. We evaluate the efficacy and robustness of our proposed approach with benchmark instances of Quadratic Assignment Problem.
更多查看译文
关键词
Self-organizing Neural Network, Evolutionary Search (ES), Adaptive Operator Selection (AOS), Markov Decision Process (MDP), Continuous State Markov Decision Processes
AI 理解论文
溯源树
样例
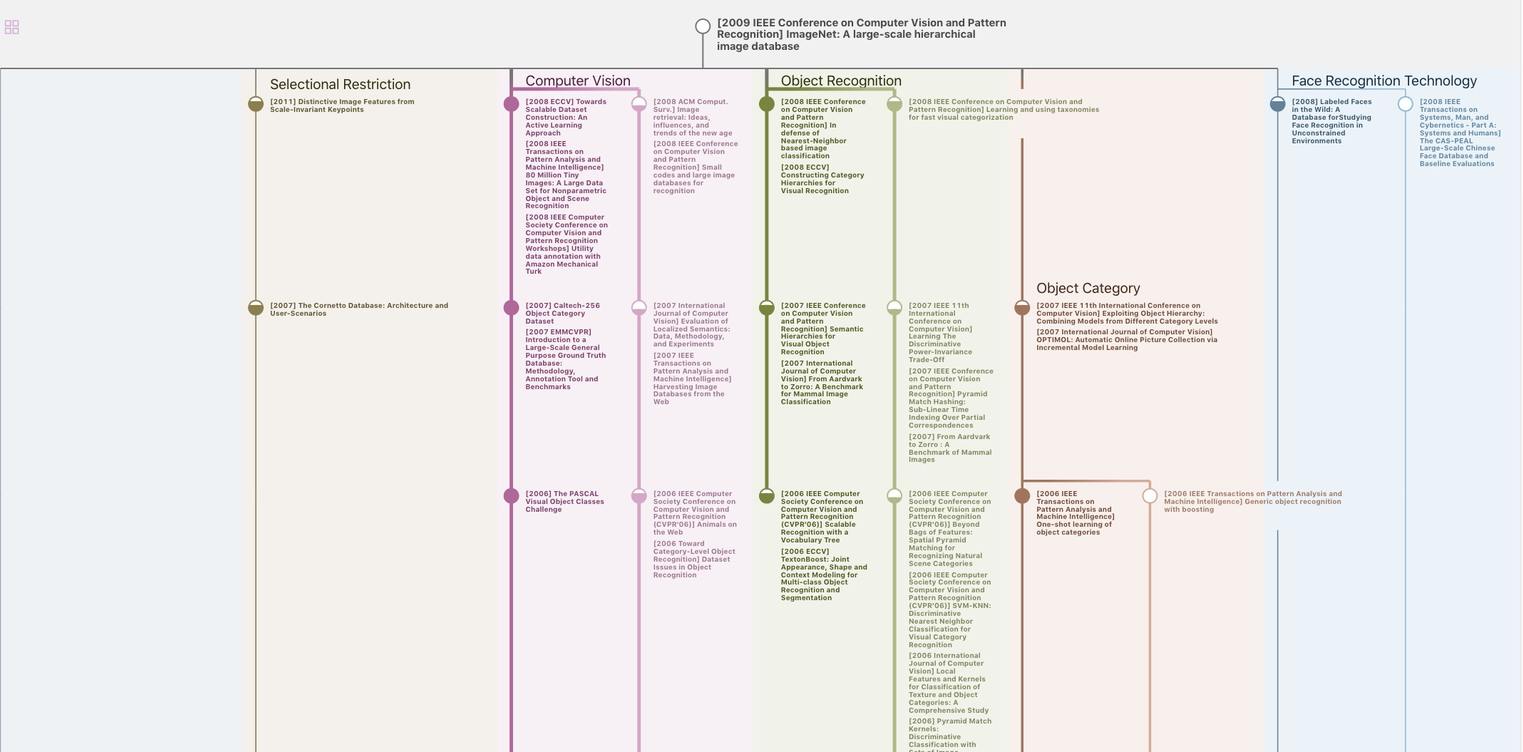
生成溯源树,研究论文发展脉络
Chat Paper
正在生成论文摘要