Predicting Actions To Act Predictably: Cooperative Partial Motion Planning With Maximum Entropy Models
2016 IEEE/RSJ International Conference on Intelligent Robots and Systems (IROS)(2016)
摘要
This paper reports on a data-driven motion planning approach for interaction-aware, socially-compliant robot navigation among human agents. Autonomous mobile robots navigating in workspaces shared with human agents require motion planning techniques providing seamless integration and smooth navigation in such. Smooth integration in mixed scenarios calls for two abilities of the robot: predicting actions of others and acting predictably for them. The former requirement requests trainable models of agent behaviors in order to accurately forecast their actions in the future, taking into account their reaction on the robot's decisions. A human-like navigation style of the robot facilitates other agents most likely not aware of the underlying planning technique applied to predict the robot motion vice versa, resulting in smoother joint navigation. The approach presented in this paper is based on a feature-based maximum entropy model and is able to guide a robot in an unstructured, real-world environment. The model is trained to predict joint behavior of heterogeneous groups of agents from onboard data of a mobile platform. We evaluate the benefit of interaction-aware motion planning in a realistic public setting with a total distance traveled of over 4 km. Interestingly the motion models learned from human-human interaction did not hold for robot-human interaction, due to the high attention and interest of pedestrians in testing basic braking functionality of the robot.
更多查看译文
关键词
actions prediction,cooperative partial motion planning,data-driven motion planning,interaction-aware socially-compliant robot navigation,human agents,autonomous mobile robots,agent behaviors,robot motion,feature-based maximum entropy model,mobile platform,interaction-aware motion planning,robot-human interaction,human-human interaction,pedestrians
AI 理解论文
溯源树
样例
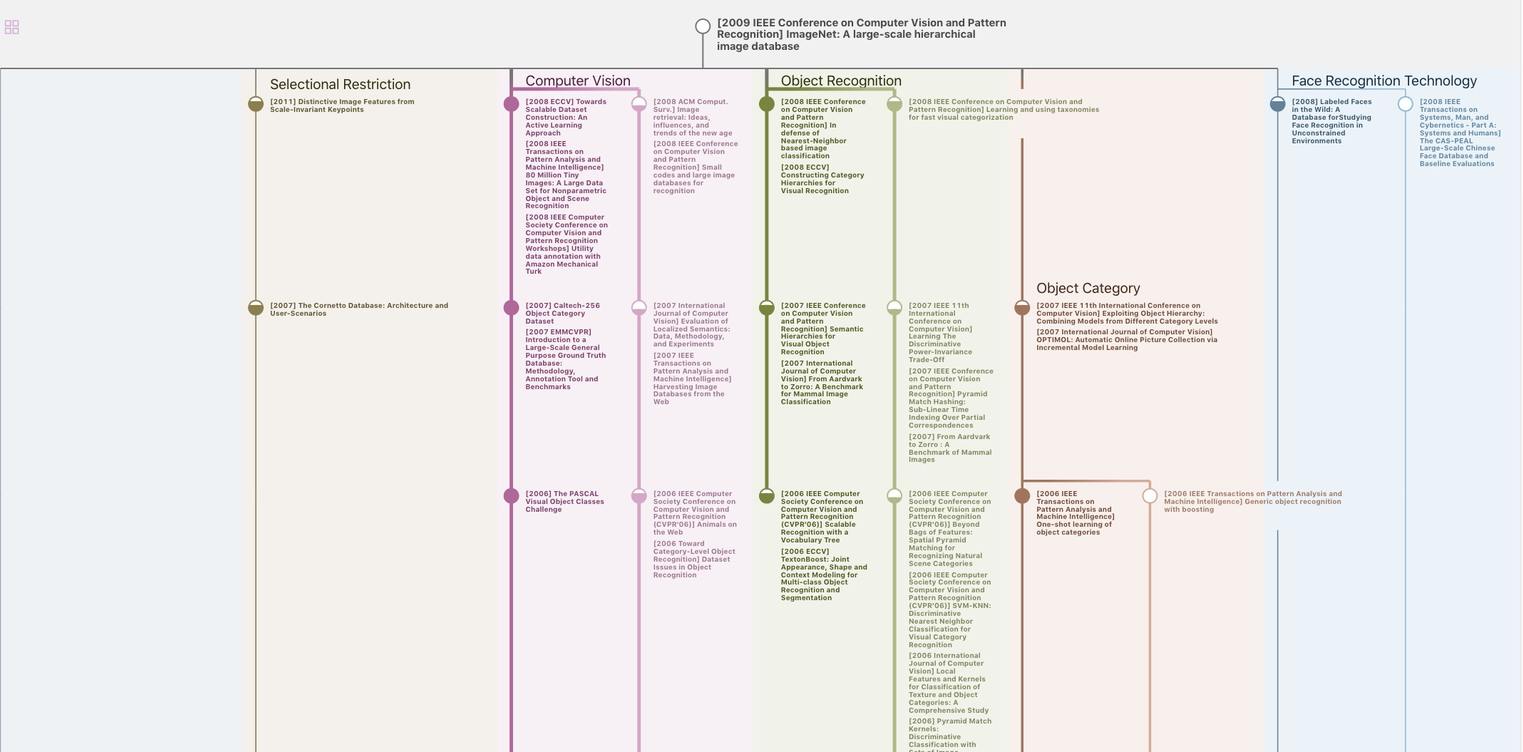
生成溯源树,研究论文发展脉络
Chat Paper
正在生成论文摘要