It'S Like Deja Vu All Over Again: Learning Place-Dependent Terrain Assessment For Visual Teach And Repeat
2016 IEEE/RSJ International Conference on Intelligent Robots and Systems (IROS)(2016)
摘要
This paper presents a learned, place-dependent terrain-assessment classifier that improves over time. Whereas typical methods aim to assess all of the terrain in a given environment, we exploit the fact that many robotic navigation tasks are well-suited to visual-teach-and-repeat navigation where robot motion is restricted to previously driven paths. In such scenarios, we argue that general terrain assessment is not required, and we can instead solve the much easier problem of detecting changes along the path; we sacrifice the ability to generalize off the path in favour of improved performance on the path. Terrain along a pretaught path is compared to terrain seen at the same location during previous, human-supervised traverses, and any significant differences cause that location to be labelled as unsafe. By storing all of our previous experiences, we are able to continuously improve our estimates as we revisit the same locations multiple times. We tested our method on two datasets collected at the University of Toronto and show that we improve over existing place-independent (both learned and not) methods, enabling nearly full autonomy in challenging, varied terrain using only a stereo camera.
更多查看译文
关键词
learning place-dependent terrain assessment classifier,visual-teach-and-repeat navigation,robot motion,stereo camera,robotic navigation tasks
AI 理解论文
溯源树
样例
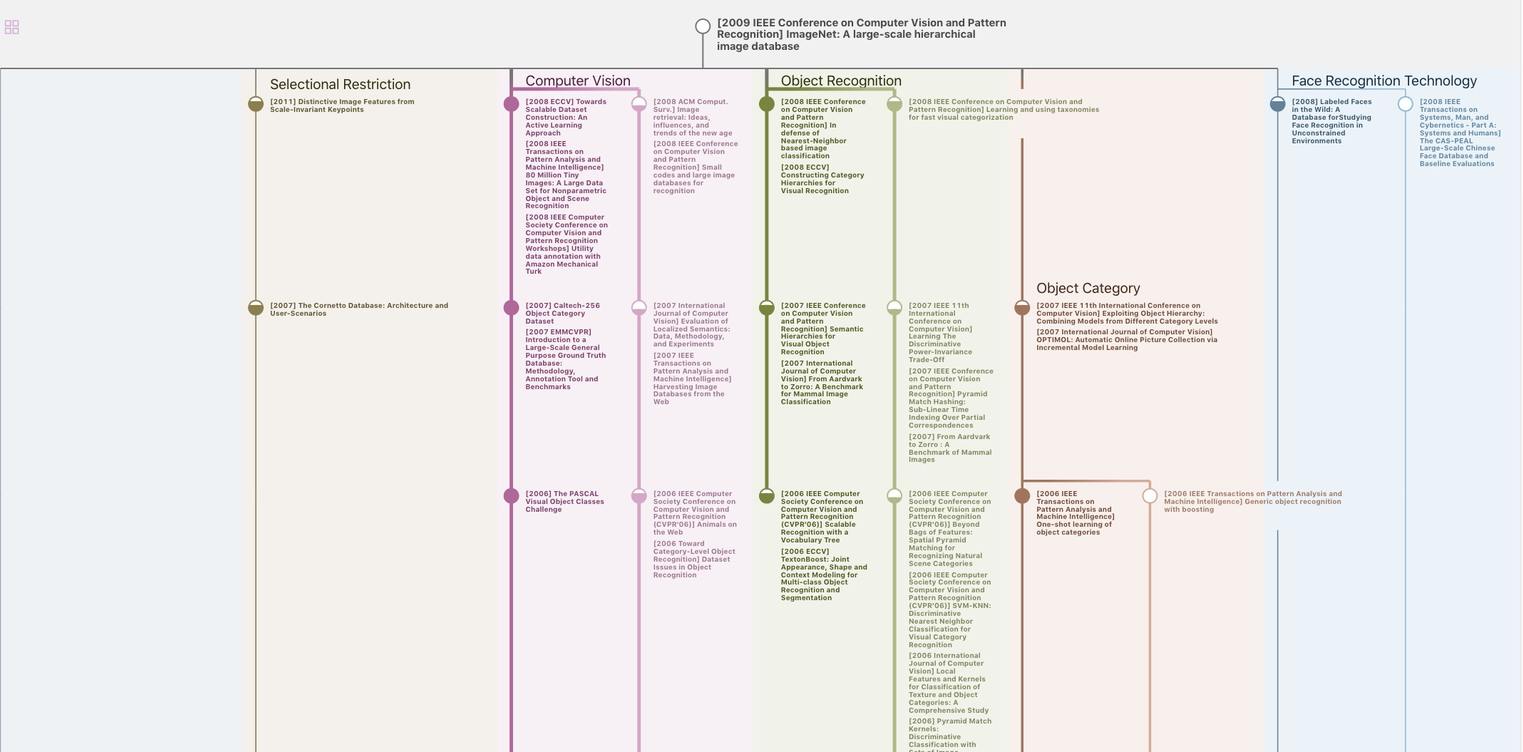
生成溯源树,研究论文发展脉络
Chat Paper
正在生成论文摘要