Unsupervised Deep Networks for Temporal Localization of Human Actions in Streaming Videos.
ISVC(2016)
摘要
We propose a deep neural network which captures latent temporal features suitable for localizing actions temporally in streaming videos. This network uses unsupervised generative models containing autoencoders and conditional restricted Boltzmann machines to model temporal structure present in an action. Human motions are non-linear in nature, and thus require continuous temporal model representation of motion which are crucial for streaming videos. The generative ability would help predict features at future time steps which can give an indication of completion of action at any instant. To accumulate M classes of action, we train an autencoder to seperate out actions spaces, and learn generative models per action space. The final layer accumulates statistics from each model, and estimates action class and percentage of completion in a segment of frames. Experimental results prove that this network provides a good predictive and recognition capability required for action localization in streaming videos.
更多查看译文
AI 理解论文
溯源树
样例
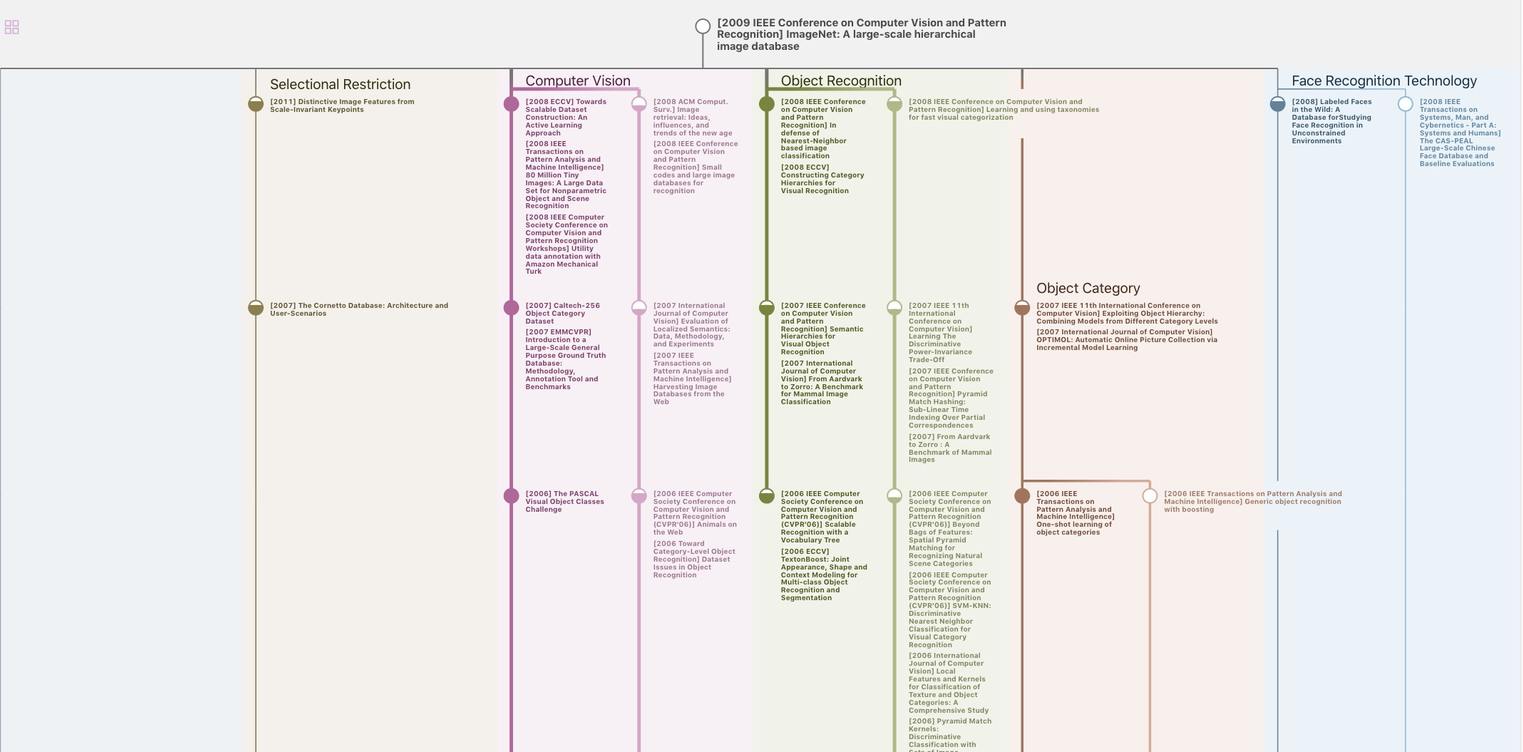
生成溯源树,研究论文发展脉络
Chat Paper
正在生成论文摘要