Scene Labeling Using Sparse Precision Matrix
2016 IEEE Conference on Computer Vision and Pattern Recognition (CVPR)(2016)
摘要
Scene labeling task is to segment the image into meaningful regions and categorize them into classes of objects which comprised the image. Commonly used methods typically find the local features for each segment and label them using classifiers. Afterwards, labeling is smoothed in order to make sure that neighboring regions receive similar labels. However, these methods ignore expressive connections between labels and non-local dependencies among regions. In this paper, we propose to use a sparse estimation of precision matrix (also called concentration matrix), which is the inverse of covariance matrix of data obtained by graphical lasso to find interaction between labels and regions. To do this, we formulate the problem as an energy minimization over a graph, whose structure is captured by applying sparse constraint on the elements of the precision matrix. This graph encodes (or represents) only significant interactions and avoids a fully connected graph, which is typically used to reflect the long distance associations. We use local and global information to achieve better labeling. We assess our approach on three datasets and obtained promising results.
更多查看译文
关键词
scene labeling,sparse precision matrix,image segmentation,local feature finding,classifiers,label smoothing,sparse estimation,concentration matrix,covariance matrix,graphical lasso,energy minimization,graph encoding,fully connected graph
AI 理解论文
溯源树
样例
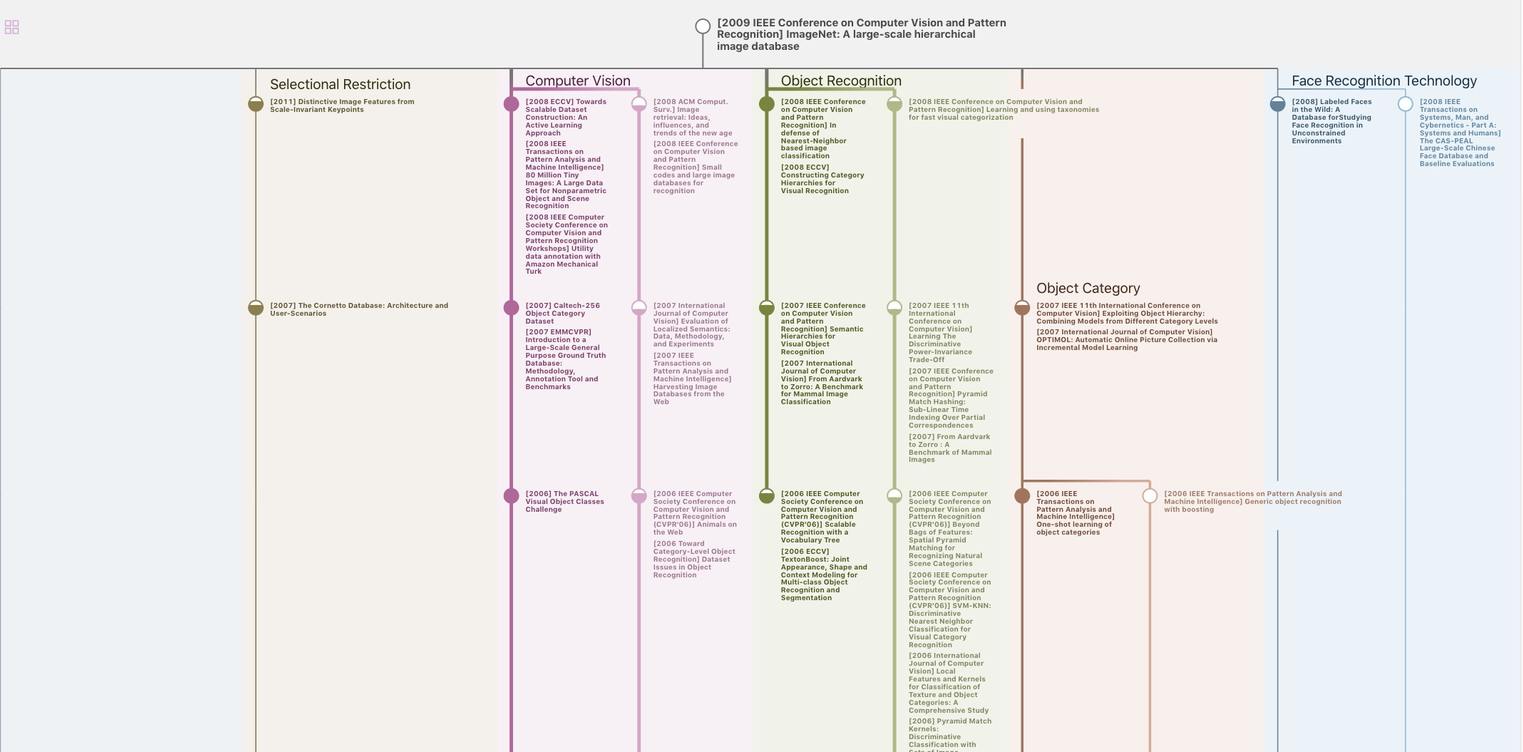
生成溯源树,研究论文发展脉络
Chat Paper
正在生成论文摘要