Text Classification With Topic-Based Word Embedding And Convolutional Neural Networks
BCB(2016)
摘要
Recently, distributed word embeddings trained by neural language models are commonly used for text classification with Convolutional Neural Networks (CNNs). In this paper, we propose a novel neural language model, Topic-based Skip-gram, to learn topic-based word embeddings for biomedical literature indexing with CNNs. Topic-based Skip-gram leverages textual content with topic models, e.g., Latent Dirichlet Allocation (LDA), to capture precise topic-based word relationship and then integrate it into distributed word embedding learning. We then describe two multimodal CNN architectures, which are able to employ different kinds of word embeddings at the same time for text classification. Through extensive experiments conducted on several real-world datasets, we demonstrate that combination of our Topic-based Skip-gram and multimodal CNN architectures outperforms state-of-the-art methods in biomedical literature indexing, clinical note annotation and general textual benchmark dataset classification.
更多查看译文
关键词
text classification,convolutional neural networks,word embeddings,medical subject headings
AI 理解论文
溯源树
样例
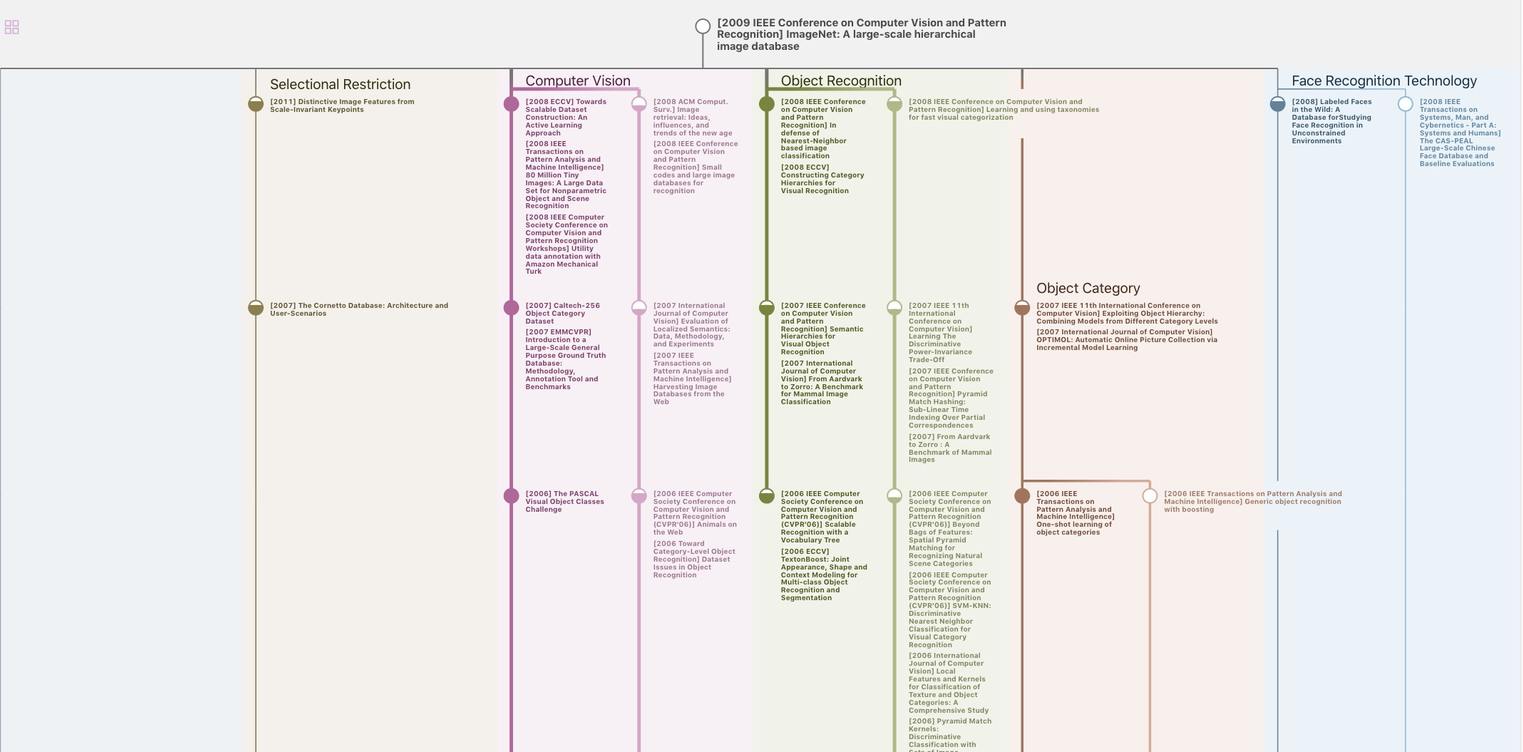
生成溯源树,研究论文发展脉络
Chat Paper
正在生成论文摘要