Anomaly Detection in Electrical Substation Circuits via Unsupervised Machine Learning
2016 IEEE 17th International Conference on Information Reuse and Integration (IRI)(2016)
摘要
Cyber-physical systems (CPS), such as smart grids, include cyber assets for monitoring, control, and communication in order to maintain safe and efficient operation of a physical process. We propose that CPS intrusion detection systems (CPS IDS) should seek not just to detect attacks in the host audit logs and network traffic (cyber plane), but should consider how attacks are reflected in measurements from diverse devices at multiple locations (physical plane). In electric grids, voltage and current laws induce physical constraints that can be leveraged in distributed agreement algorithms to detect anomalous conditions. This can be done by explicitly coding the physical constraints into a hybrid CPS IDS, making the detector specific to a particular CPS. We present an alternative approach, along with preliminary results, using machine learning to characterize normal, fault, and attack states in a smart distribution substation CPS, using this as a component of a CPS IDS.
更多查看译文
关键词
Anomaly detection,Machine learning,Smart grid,Cyber-physical system security,IEC 61850
AI 理解论文
溯源树
样例
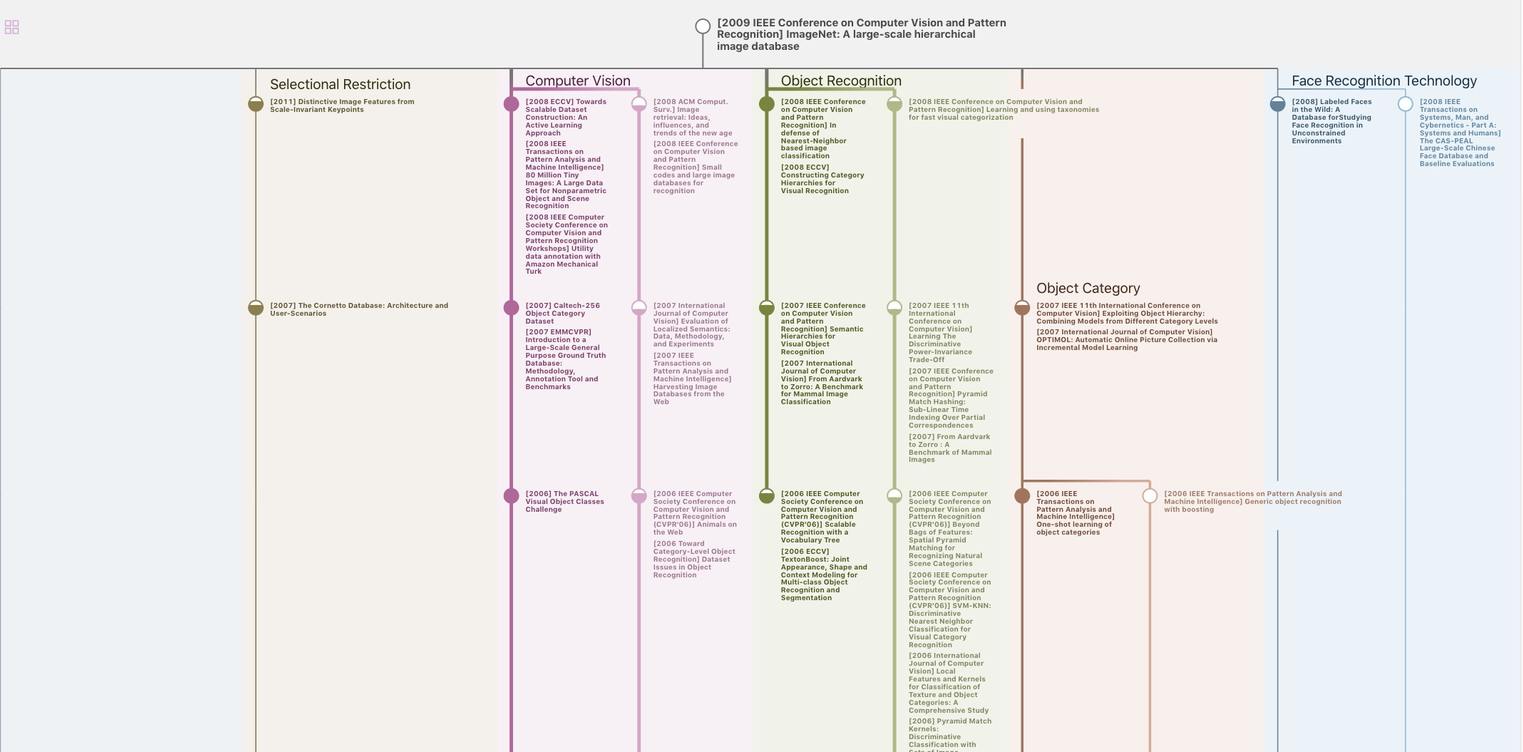
生成溯源树,研究论文发展脉络
Chat Paper
正在生成论文摘要