Using the Causal Graph to Enhance Translations to Solve Contingent Planning Problems
2016 5th Brazilian Conference on Intelligent Systems (BRACIS)(2016)
摘要
Planning with partial observation, an area called contingent planning, is a complex and challenging problem since it requires to keep track of belief states to search for a contingent plan of actions. Recent approaches considers the agent's knowledge about the world to compile a contingent planning problem into a full observable planning problem, described in an epistemic logic language, and then use an efficcient full observable planner to solve the translated problem. In this paper we use the concept of relevance and causality to propose a new translation based in a structure called Causal Graph that can improve the belief tracking task of contingent Planning problems described in a more general planning language, in particular problems envolving actions with uncertainty on its conditional effects.
更多查看译文
关键词
Automated Planning,Knowledge Representation and Reasoning
AI 理解论文
溯源树
样例
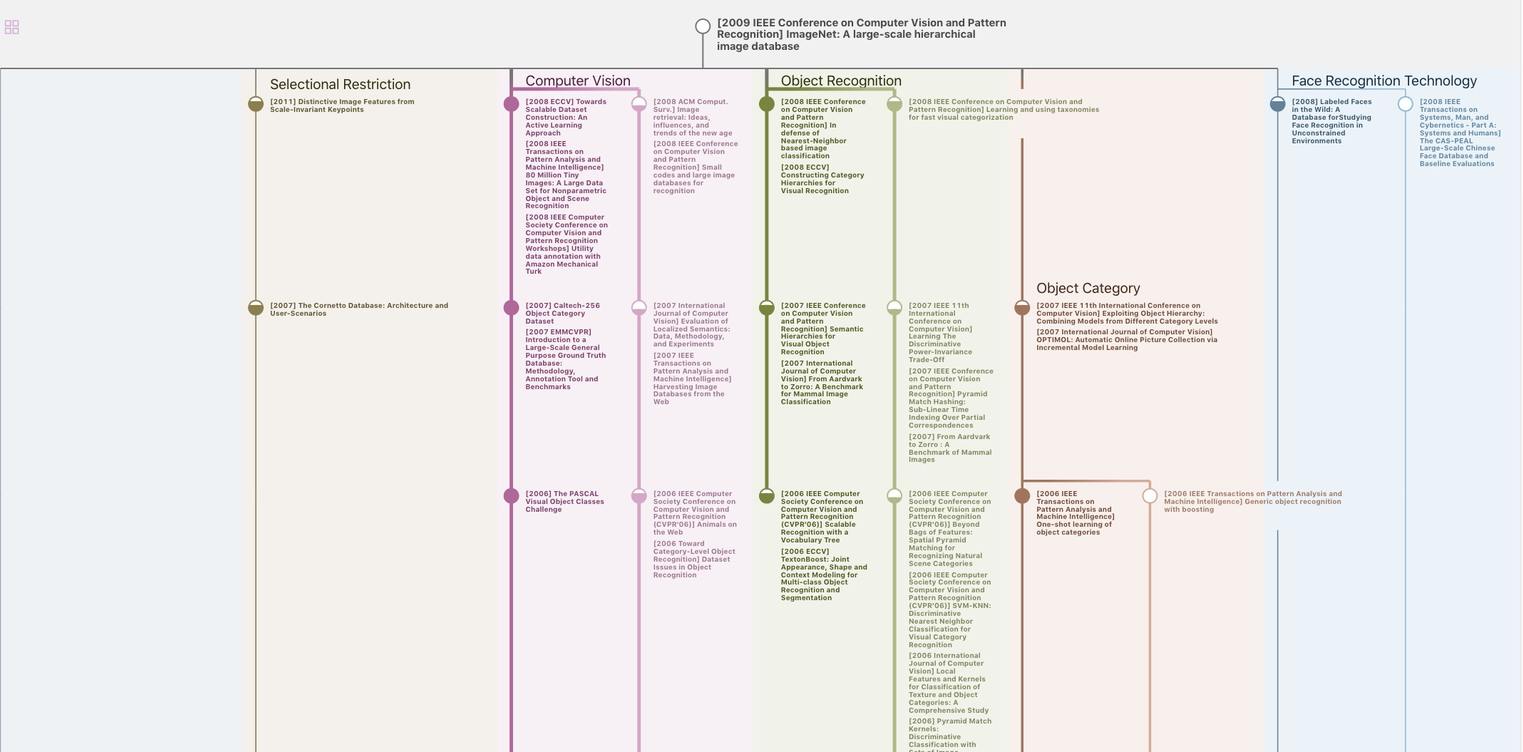
生成溯源树,研究论文发展脉络
Chat Paper
正在生成论文摘要