Two-Stage Data Augmentation For Low-Resourced Speech Recognition
17TH ANNUAL CONFERENCE OF THE INTERNATIONAL SPEECH COMMUNICATION ASSOCIATION (INTERSPEECH 2016), VOLS 1-5: UNDERSTANDING SPEECH PROCESSING IN HUMANS AND MACHINES(2016)
摘要
Low resourced languages suffer from limited training data and resources. Data augmentation is a common approach to increasing the amount of training data. Additional data is synthesized by manipulating the original data with a variety of methods. Unlike most previous work that focuses on a single technique, we combine multiple, complementary augmentation approaches. The first stage adds noise and perturbs the speed of additional copies of the original audio. The data is further augmented in a second stage, where a novel fMLLR-based augmentation is applied to bottleneck features to further improve performance. A reduction in word error rate is demonstrated on four languages from the IARPA Babel program. We present an analysis exploring why these techniques are beneficial.
更多查看译文
关键词
speech recognition, deep neural networks, data augmentation
AI 理解论文
溯源树
样例
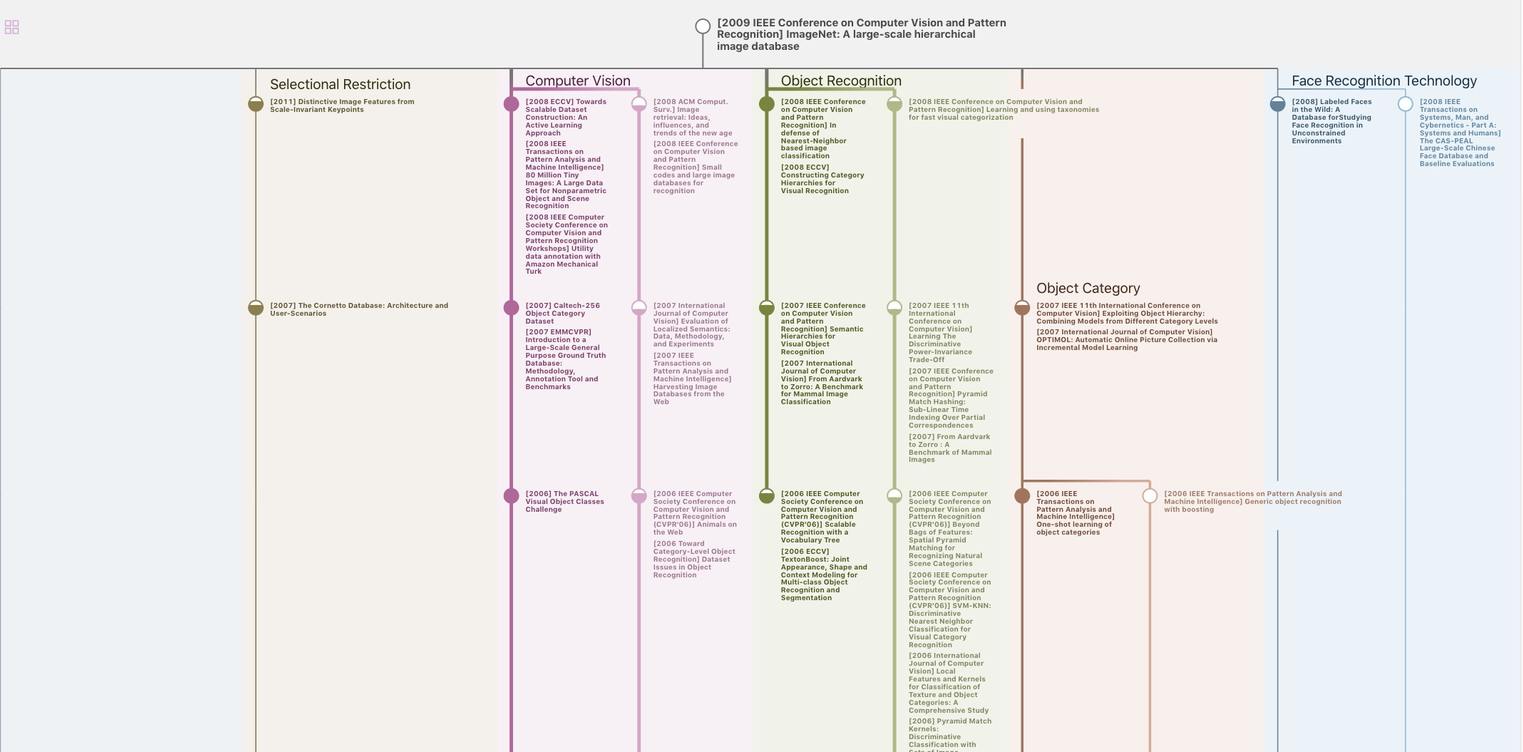
生成溯源树,研究论文发展脉络
Chat Paper
正在生成论文摘要