Personalized location models with adaptive mixtures.
SIGSPATIAL/GIS(2016)
摘要
Personalization is increasingly important for a range of applications that rely on location-based modeling. A key aspect in building personalized models is using population-level information to smooth noisy sparse data at the individual level. In this paper we develop a general mixture model framework for learning individual-level location models where the model adaptively combines different types of smoothing information. In a series of experiments with Twitter geolocation data and Gowalla check-in data we demonstrate that the proposed approach can be significantly more accurate than more traditional smoothing and matrix factorization techniques. The improvement in performance over matrix factorization is pronounced and may be explained by the tendency of dimensionality reduction methods to over-smooth and not retain enough detail at the individual level.
更多查看译文
关键词
Location data, Personalization, Mixture models
AI 理解论文
溯源树
样例
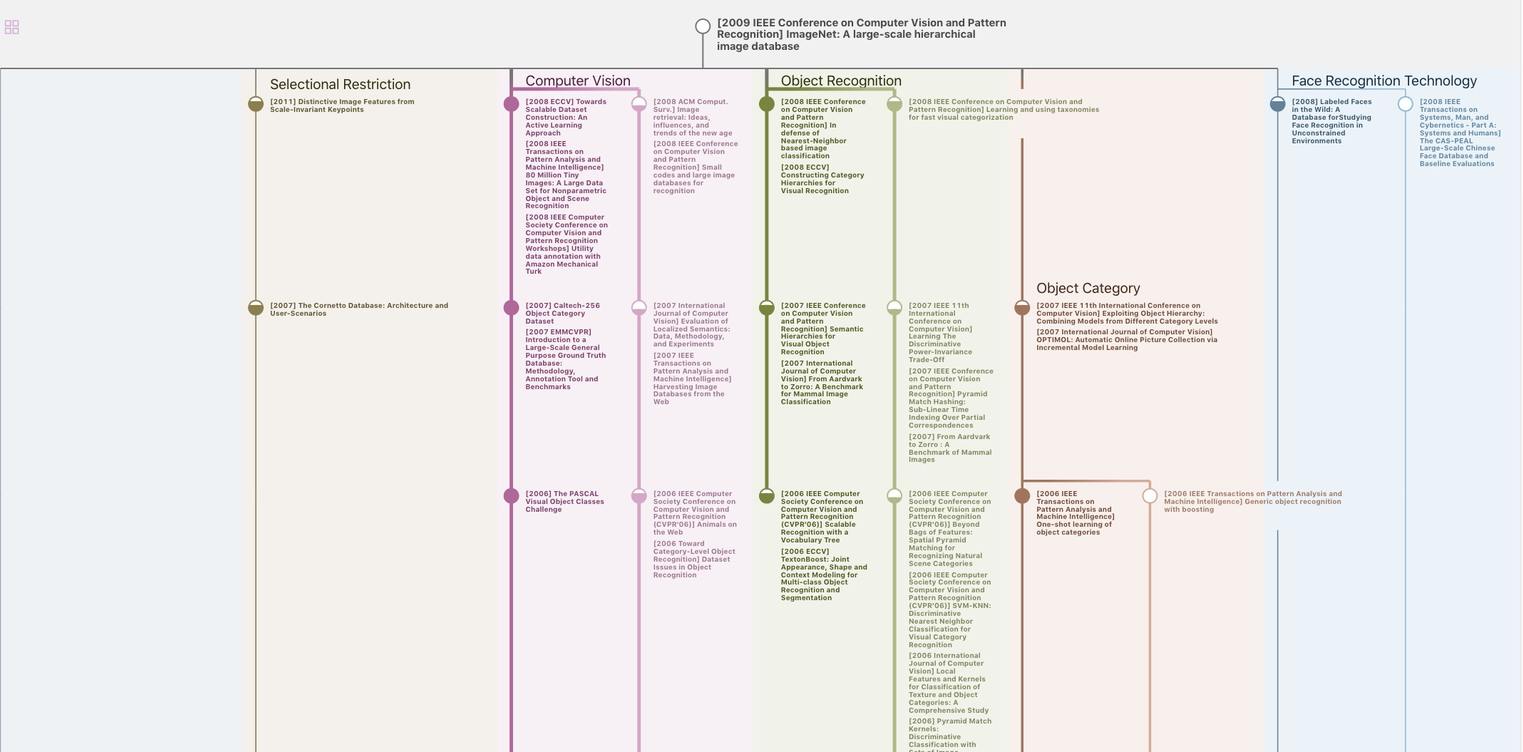
生成溯源树,研究论文发展脉络
Chat Paper
正在生成论文摘要