Action Recognition: From Static Datasets to Moving Robots
2017 IEEE International Conference on Robotics and Automation (ICRA)(2017)
摘要
Deep learning models have achieved state-of-the- art performance in recognizing human activities, but often rely on utilizing background cues present in typical computer vision datasets that predominantly have a stationary camera. If these models are to be employed by autonomous robots in real world environments, they must be adapted to perform independently of background cues and camera motion effects. To address these challenges, we propose a new method that firstly generates generic action region proposals with good potential to locate one human action in unconstrained videos regardless of camera motion and then uses action proposals to extract and classify effective shape and motion features by a ConvNet framework. In a range of experiments, we demonstrate that by actively proposing action regions during both training and testing, state-of-the-art or better performance is achieved on benchmarks. We show the outperformance of our approach compared to the state-of-the-art in two new datasets; one emphasizes on irrelevant background, the other highlights the camera motion. We also validate our action recognition method in an abnormal behavior detection scenario to improve workplace safety. The results verify a higher success rate for our method due to the ability of our system to recognize human actions regardless of environment and camera motion.
更多查看译文
关键词
action recognition,static datasets,moving robots,deep learning models,background cues,computer vision,stationary camera,autonomous robots,camera motion,ConvNet framework
AI 理解论文
溯源树
样例
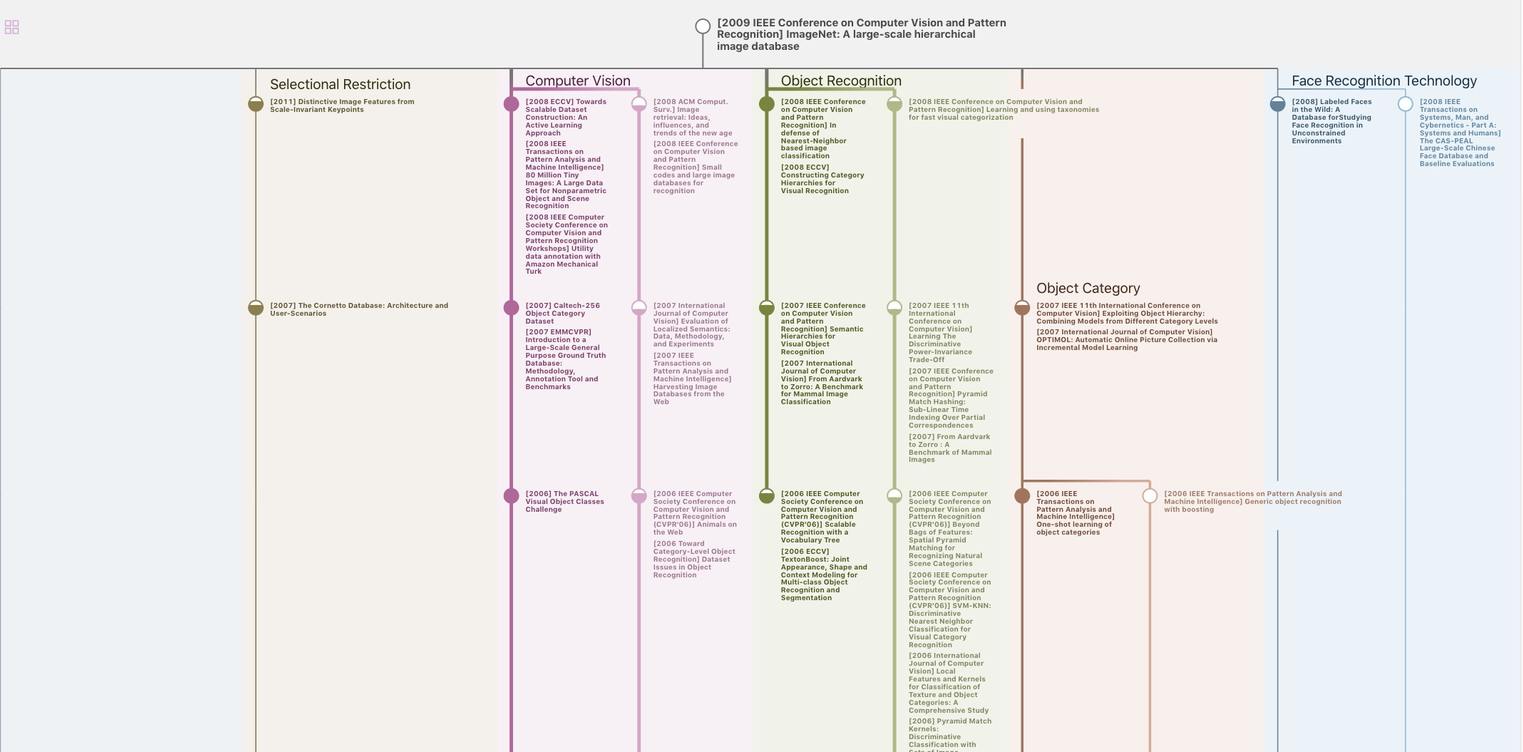
生成溯源树,研究论文发展脉络
Chat Paper
正在生成论文摘要