EnhanceNet: Single Image Super-Resolution Through Automated Texture Synthesis
2017 IEEE International Conference on Computer Vision (ICCV)(2017)
摘要
Single image super-resolution is the task of inferring a high-resolution image from a single low-resolution input. Traditionally, the performance of algorithms for this task is measured using pixel-wise reconstruction measures such as peak signal-to-noise ratio (PSNR) which have been shown to correlate poorly with the human perception of image quality. As a result, algorithms minimizing these metrics tend to produce over-smoothed images that lack high-frequency textures and do not look natural despite yielding high PSNR values. We propose a novel application of automated texture synthesis in combination with a perceptual loss focusing on creating realistic textures rather than optimizing for a pixel-accurate reproduction of ground truth images during training. By using feed-forward fully convolutional neural networks in an adversarial training setting, we achieve a significant boost in image quality at high magnification ratios. Extensive experiments on a number of datasets show the effectiveness of our approach, yielding state-of-the-art results in both quantitative and qualitative benchmarks.
更多查看译文
关键词
single image super-resolution,automated texture synthesis,high-resolution image,single low-resolution input,pixel-wise reconstruction measures,peak signal-to-noise ratio,image quality,highfrequency textures,high PSNR values,realistic textures,ground truth images
AI 理解论文
溯源树
样例
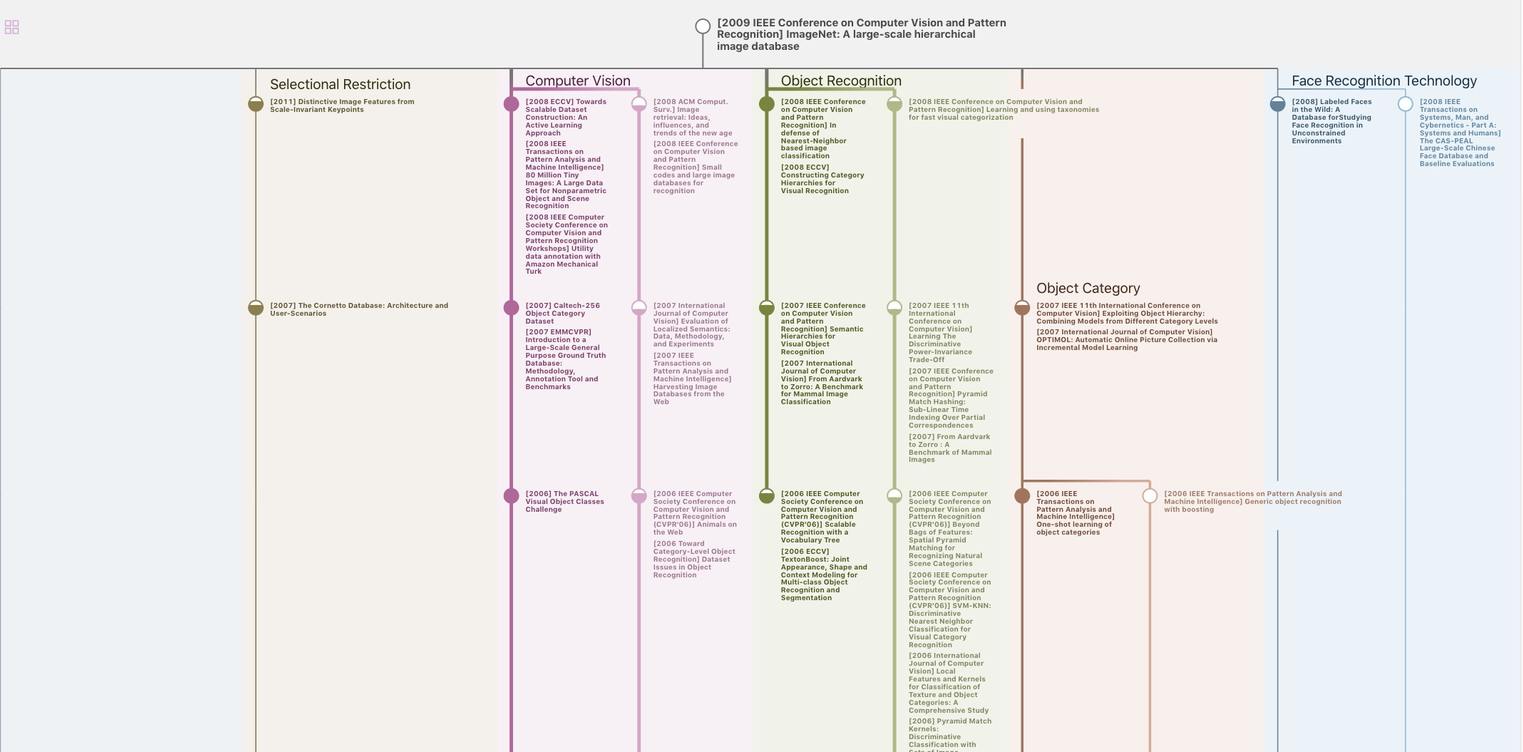
生成溯源树,研究论文发展脉络
Chat Paper
正在生成论文摘要