Factorized Bilinear Models for Image Recognition
2017 IEEE INTERNATIONAL CONFERENCE ON COMPUTER VISION (ICCV)(2017)
摘要
Although Deep Convolutional Neural Networks (CNNs) have liberated their power in various computer vision tasks, the most important components of CNN, convolutional layers and fully connected layers, are still limited to linear transformations. In this paper, we propose a novel Factorized Bilinear (FB) layer to model the pairwise feature interactions by considering the quadratic terms in the transformations. Compared with existing methods that tried to incorporate complex non-linearity structures into CNNs, the factorized parameterization makes our FB layer only require a linear increase of parameters and affordable computational cost. To further reduce the risk of overfitting of the FB layer, a specific remedy called DropFactor is devised during the training process. We also analyze the connection between FB layer and some existing models, and show FB layer is a generalization to them. Finally, we validate the effectiveness of FB layer on several widely adopted datasets including CIFAR-10, CIFAR-100 and ImageNet, and demonstrate superior results compared with various state-of-the-art deep models.
更多查看译文
关键词
DropFactor,image recognition,Factorized Bilinear layer,FB layer,fully connected layers,convolutional layers,computer vision tasks,Deep Convolutional Neural Networks,Bilinear models
AI 理解论文
溯源树
样例
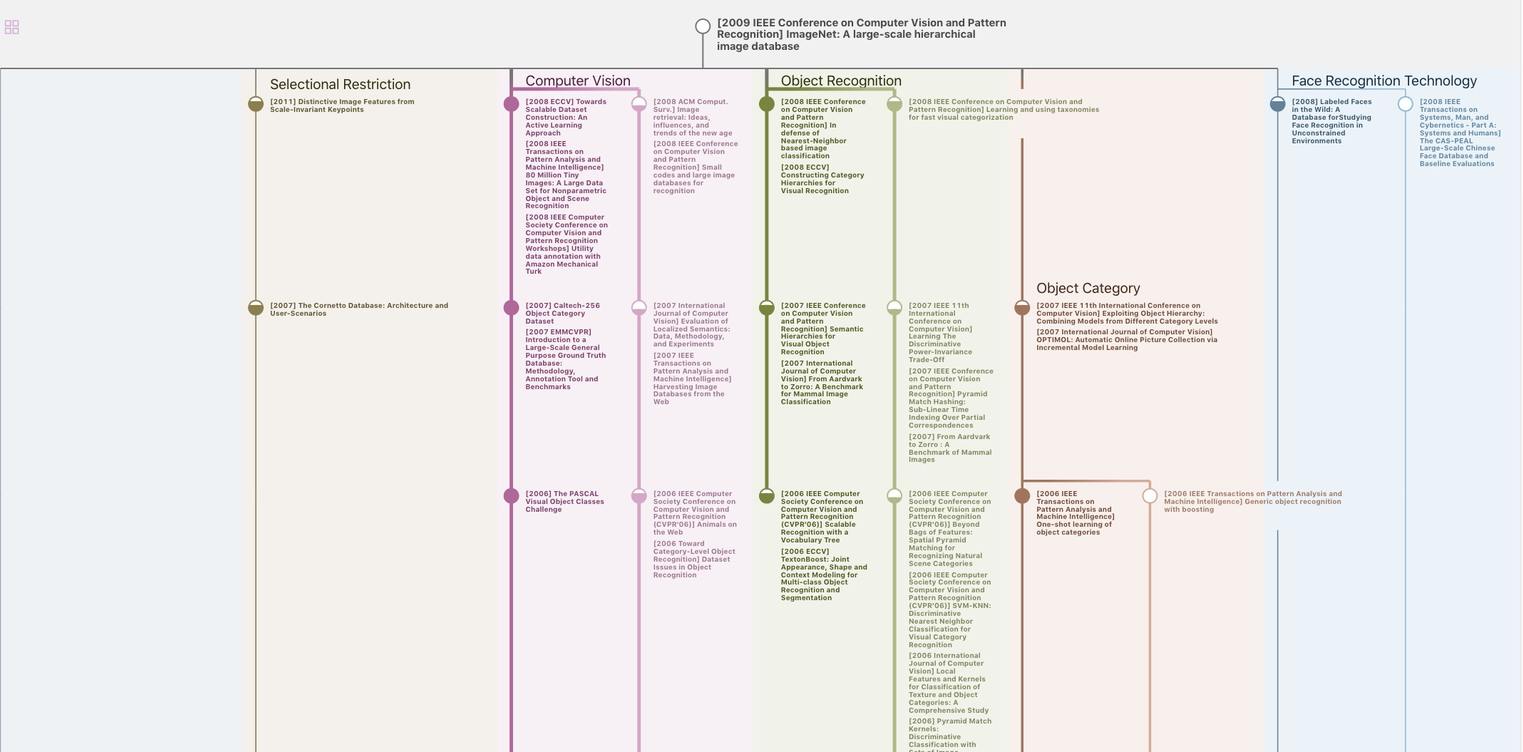
生成溯源树,研究论文发展脉络
Chat Paper
正在生成论文摘要